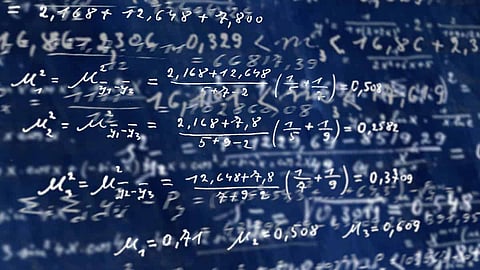
The entire world is digitalized today. There is a sense of knowledge; there is a feeling of communication in every traditional gadget that makes our life so easy, so smooth. All these technological progressions are taken forward by programming which is a bunch of software that is intended to take care of an issue. Most importantly, every program is based upon a logic/solution which is called an Algorithm.
The algorithm is one of the stepping stones of our innovative world and is driven by the researchers and specialists in the background that design these various algorithms. Computer scientists and specialists create and deploy algorithms to make explicit jobs simpler and quicker to perform. Engineers use algorithms to figure out large sets of data, to discover crucial data more quickly.
Algorithms drive automated machines to make decisions. These machines are progressively making choices that have real-world implications and outcomes. The accessibility to huge data collections has made it simple to extract new bits of knowledge through computers. Subsequently, algorithms, which are a set of step-by-step guidelines that computers follow to carry out a task, have become more complex and prevalent devices for automated decision making.
Algorithms are utilizing volumes of micro and macro scale information to impact decisions influencing individuals in a range of tasks, from making film suggestions to helping banks decide the financial soundness of people. Therefore, the safety and reliability of algorithms is an increasing concern. Any predisposition in these algorithms could adversely affect individuals or groups of individuals. Machines, similar to people, are guided by information and experience.
If that information or experience is mixed up or abnormal, a biased decision can be made, regardless of whether that decision is made by a human or a machine. However, because machines can treat likewise individuals and objects in a different way, research is beginning to reveal some alarming examples in which the reality of algorithmic decision making falls short of our expectations.
Given this, a few algorithms risk imitating and in some events, intensifying human biases, especially those influencing secured groups. For instance, automated risk assessments utilized by U.S. judges to decide bail and sentencing limits can produce wrong conclusions, bringing about huge combined consequences for specific groups, similar to longer jail sentences or higher bails forced on individuals of color.
Various circumstances and factors lead to biases in algorithms. However, algorithms don't have an ethical character. It is we who need to figure out how to determine what we want. Historical human predispositions are molded by unavoidable and frequently deeply implanted prejudices against specific groups, which can prompt their propagation and amplification in computer models.
Every choice we make includes a specific sort of bias. People like you and me generally create biases while analyzing information. Purposely or accidentally, we all have certain internal biases that can be portrayed in the data collection process involved when building AI models.
Algorithms haven't proved to be vastly developed. In an ideal world, we would need our algorithms to settle on better-informed choices without inclinations to guarantee better social equity, i.e., equivalent opportunities for people and groups, (for example, minorities) within society to utilize resources, have their voices heard, and be represented in the public arena.
At the point when these algorithms carry out the responsibility of intensifying racial, social, and gender inequality, rather than reducing it; it becomes important to assess the moral repercussions and possible misuse of the technology. It is significant for algorithm designers and administrators to look for such potential negative feedbacks that cause an algorithm to turn out to be progressively biased after some time.
The issue is the algorithms that we are placing into these systems are not traditional hand-coded algorithms. Rather, these are the yield of machine learning processes. What's more important, nowhere in an AI training procedure is an individual sitting down and coding everything the algorithm must do in each situation.
They simply determine an objective function. Certain specialists argue the algorithms utilized by professionals in AI are very transparent. They're not sophisticated. They're short and straightforward and they're encoding a logical principle. Researchers also believe the issue is with the scientific rule in any case, not with the individuals implementing it or the algorithm executing it.
The source of terrible behavior isn't some malintent of a software engineer and that makes it harder to control. You need to understand the source of irregular behavior and how to fix it. One of them, importantly, is a bias that is already present in the data. AI algorithms are just attempting to discover patterns in the information you provide to them. There is no reason to believe they're going to evacuate biases that are already present in the data.
Properly assembled algorithms help save time by finding and programming the fastest way to complete something. Accordingly, it is essential to comprehend the source of bias associated with the algorithm to keep the algorithm effective and precise.
Join our WhatsApp Channel to get the latest news, exclusives and videos on WhatsApp
_____________
Disclaimer: Analytics Insight does not provide financial advice or guidance. Also note that the cryptocurrencies mentioned/listed on the website could potentially be scams, i.e. designed to induce you to invest financial resources that may be lost forever and not be recoverable once investments are made. You are responsible for conducting your own research (DYOR) before making any investments. Read more here.