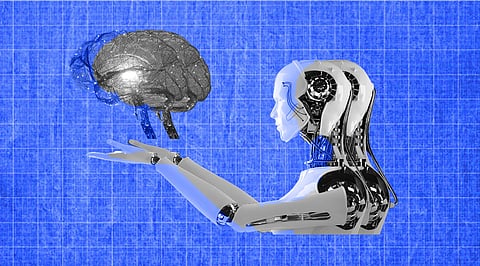
To increase the precision of AI systems, the data-centric approach involves methodically optimising datasets. This strategy is considered promising by machine learning experts since refined data produces superior results to raw data. A data-centric strategy seeks to ensure high-quality data input rather than tinkering with model settings.
The training data used in machine learning consists of labelled pictures, words, audio files, videos, and other types of data. The developed model and its optimization will perform badly if the training data is subpar. Through AI-based chatbots, this might result in terrible consumer experiences, but in a biological algorithm or an autonomous car, it might be fatal.
Data quality depends on exact, accurate, and consistent annotation creation. You cannot create a model correctly if your data are not correctly labelled. You won't be able to create a robust model if your data amount is insufficient. Data annotation, however, involves more than simply the quantity and quality of labelled data; it also involves the type of tags you use for the models you're creating. After all, even if we continued with the "model-centric" strategy, our model would remain static without providing best-in-class data labelling. This is the first step in developing a computer vision model: high-quality, scaleable labelled data. Whether you're performing detection, segmentation, or classification, you must annotate your data before building a computer vision model.
A data-stable strategy is one that is data-centric. This indicates that you are handling your data's whole lifecycle. You need to monitor the development of your dataset even before you create your model. Your datasets must be able to be filtered, sorted, copied, combined, versioned, and queried right down to the metadata level. As your AI project progresses, providing a single safe visualisation layer for all of your unstructured data will help you better comprehend the mountain of acquired data. Data engineers, data scientists, and data operators may evaluate data sets more rapidly and effectively with the help of robust tools.
The power to automate your analysis and data management routines will probably be the most crucial component of effectively maintaining a data-centric versus model-centric strategy as you ultimately grow your AI project. Being capable of pre- and post-processing your datasets is just as important as releasing your models into production. The key is being able to grow your work as you rewrite and optimise your constantly-adapting models and being able to generate human-in-the-loop data validation. With the help of Dataloop's solution, businesses can build unique data automation pipelines that combine machine learning and human labelling jobs using a drag-and-drop interface with no programming required.
Join our WhatsApp Channel to get the latest news, exclusives and videos on WhatsApp
_____________
Disclaimer: Analytics Insight does not provide financial advice or guidance. Also note that the cryptocurrencies mentioned/listed on the website could potentially be scams, i.e. designed to induce you to invest financial resources that may be lost forever and not be recoverable once investments are made. You are responsible for conducting your own research (DYOR) before making any investments. Read more here.