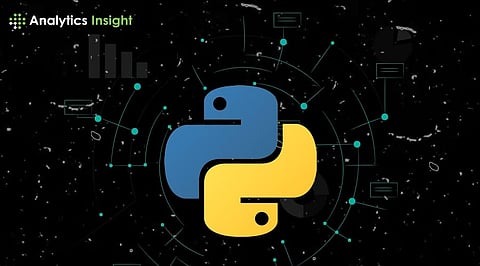
In today's data-driven world, the ability to extract insights from vast amounts of information is a valuable asset. Python, with its ease of use, powerful libraries, and vast community, has become the go-to language for data science. But what key Python skills should you acquire for handling data effectively?
Python is instrumental in data science due to its user-friendly syntax and extensive libraries like NumPy and pandas, simplifying tasks such as data manipulation and analysis. Additionally, Python serves as a primary language for machine learning frameworks like TensorFlow and PyTorch, making it a versatile and essential tool for developing and deploying machine learning models in data science workflows. Let us delve into the core of Python for data science.
Before diving into data-specific libraries, a solid understanding of Python's core concepts is crucial. This includes:
Syntax: Object-oriented programming (OOP), operators, data types, variables, control flow statements, and functions.
Data Structures: Lists, tuples, dictionaries, sets, and their manipulation using list comprehensions, generators, and lambda functions. These structures efficiently store and organize diverse data types.
Modules and Packages: Importing and utilizing external libraries like NumPy, Pandas, and Matplotlib, which expand Python's functionalities for numerical computing, data manipulation, and visualization.
Pandas, a proficient tool for data wrangling, excels in:
NumPy in Python enables efficient numerical computing, providing arrays and functions for advanced mathematical operations:
Visualization is key to communicating insights effectively. Matplotlib and Seaborn provide versatile tools for:
The path to the field of machine learning begins with Scikit-learn. It offers:
For complex problems, dive into deep learning frameworks like TensorFlow and PyTorch:
As you progress, explore specialized libraries for:
The journey to data science mastery is continuous. Start by building a strong foundation in Python and core libraries, then gradually expand your skillset based on your specific interests and goals. Practice consistently, and participate in online communities.
Join our WhatsApp Channel to get the latest news, exclusives and videos on WhatsApp
_____________
Disclaimer: Analytics Insight does not provide financial advice or guidance. Also note that the cryptocurrencies mentioned/listed on the website could potentially be scams, i.e. designed to induce you to invest financial resources that may be lost forever and not be recoverable once investments are made. You are responsible for conducting your own research (DYOR) before making any investments. Read more here.