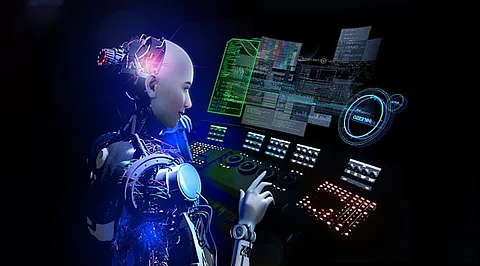
Artificial Intelligence (AI) has invaded the healthcare sector long back. It is making accountable impacts on treatment and overviewing of patients. However, psychiatry department stands out when it comes to utilising AI applications. It has taken a long way before reaching the current initial stage where AI is being used for analyzing patients but only by a handful of psychiatrists.
Medicine is already reaping a fruitful benefit from artificial intelligence and big data. It has shown promising results in diagnosing disease, interpreting images and concentrating on treatment plans. Though psychiatry is in many ways a uniquely human field, requiring emotional intelligence and perception that computers can't stimulate, experts say that AI could have an impact. The field could profit from artificial intelligence's ability to analyze data and pick up on patterns and warning signs so subtle humans might never notice them.
However, connecting psychiatry with artificial intelligence and big data is not an easy job. Psychiatrists and behavioral health researchers found it difficult to make the connection on how to implement artificial intelligence into actual psychiatry use cases. Today, both medicine and technology are breaking their barriers to make a change.
Predictive modeling is generally building machine learning algorithms that are used to predict future events by utilizing historical data. Predictive modeling in psychiatry is aiding doctors to predict which treatment is likely to work for patients with issues like anxiety and depression. Generally, doctors segment patients on three rows according to their response to the treatment.
Before the invasion of artificial intelligence, doctors used to manually segment the patients according to clinical intuition, presentation and history to predict which group the patient belonged to. However, most of the human analysis was mere guesses rather than accurate answers. This swayed the treatment from being exact to somewhere close it. Henceforth, utilizing artificial intelligence with predictive modeling would help improve the matching of the patients to the right group, so the right treatment can be started quickly.
Classifying and concentrating on non-responders is a critical task. They are patients who need immediate attention. Artificial intelligence segregates them and indicates it to the psychiatrist who can show special care for the needy.
Computational phenotyping is utilizing computational techniques such as machine learning to classify illnesses and other clinical concepts from data itself. Traditionally, phenotyping psychiatric disorders involved using supervised learning and relied on domain experts with two main limitations.
Even though when the initiative began well, the traditional approach to phenotyping psychiatric disorders failed to acknowledge that a psychiatric disorder as a single condition may really have several subtypes with different phenotypes, as seen to be the case with depression and schizophrenia. Recently, computational phenotyping is using unsupervised learning to find novel patterns with regards to grouping psychiatric disorders based on observation of prognostic similarity. This unsupervised learning approach of utilizing computational power and machine learning clustering algorithms shows great potential for finding patterns in Electronic Health Records that would otherwise be hidden and that can lead to a greater understanding of psychiatric conditions and treatments.
The process of phenotyping involves raw patient data from different sources such as demographic information, diagnosis, medication, procedure, lab tests and clinical notes. Computational phenotyping turns the raw patient data into psychiatric concepts or phenotypes by utilizing computational power and clustering machine learning algorithm.
While treating patients, doctors often compare the current patient with previous patients with a similar disorder. This is called case-based reasoning. Psychiatrists use a computer algorithm to address the case-based reasoning.
Whenever a patient comes, the psychiatrist does an examination of the patient and search for similar past cases in the database. The computer algorithm then provides a list of those potentially similar patients. The psychiatrist will provide some supervision on that result to find those truly similar patients through this specific clinical context. He/she will take them as a group and see which treatment worked best. The psychiatrist recommends the same treatment to the current patient.
Artificial intelligence is believed to make more changes in psychiatry soon. Already, mobile apps and online bot consulting are being highly utilized by people. The future that researchers and scientists look for in artificial intelligence is human-like AI robots that comfort mentally unstable patients.
Join our WhatsApp Channel to get the latest news, exclusives and videos on WhatsApp
_____________
Disclaimer: Analytics Insight does not provide financial advice or guidance. Also note that the cryptocurrencies mentioned/listed on the website could potentially be scams, i.e. designed to induce you to invest financial resources that may be lost forever and not be recoverable once investments are made. You are responsible for conducting your own research (DYOR) before making any investments. Read more here.