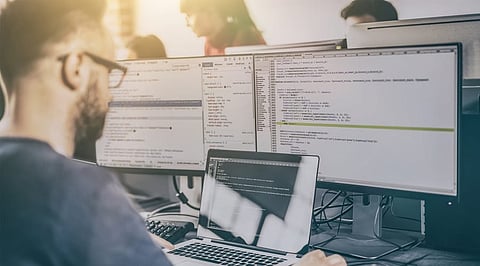
We are in the modern era of data platforms where we get complete solutions for ingesting, analyzing, and visualizing the data from multiple systems in an organization. The self-serve platforms are provided to the users on a subscription-based model, and some organizations would prefer building the whole stack by themself. Building a data platform that suits the needs of its users will require the organization to invest a lot of time in thinking and implementing it from end to end based on the use case. This leads us to a debate: To build or buy data platforms? This article will help you to decide the best choice for you.
Data is complex and data sources are plentiful. A modern data platform should have the following features to handle complex data and multiple data sources effectively.
• Keep it simple: The idea of a data platform is to democratize the data and to keep it simple right from the beginning. The platform should be easier to set up and also have a minimal number of steps from the sign-up to getting started in linking the sources. The components should be built on Open Standards and if REST APIs are provided to the components, it's easier for integration.
• Self-serve: If an organization is data-driven, the data can never be siloed. The platform can be intuitively used by different teams in the organization, understand the context and derive insights from the data.
• Robustness: Modern data platforms are robust with a separation between data and compute layers. This helps in the increased availability and scaling of the computing power when required. Costs are optimized as well since the computing power is elastic and auto-scalable.
• Business Intelligence: Modern data platforms come with batteries included. The teams can utilize the platform's BI infrastructure to publish their findings in the forms of applications and dashboards. This helps in the faster delivery of insights.
• Data Security: Unless the data resides in your cloud, you need to make sure that the SaaS solutions securely handle your data. It needs to be compliant with the federal laws and the policies imposed by the data owners.
No matter how you build or evaluate a potential paid data platform, you need to make sure that it contains all of these salient features.
Now, to the part of whether to build or buy. Let's compare the individual components of the data platform and compare their pros and cons.
The connectors of the platform should support the extraction and loading of a variety of data sources as micro-batches and streams. With cheaper compute resources, it is wiser to transform the data post-loading. Depending on the number of data sources that you extract from, the connectors in the data platform need to be capable of extraction and loading. When you buy a data platform, you are provided with the widely used connectors, out of the box. However, if newer sources or destinations that are not supported by the platform need to be connected to your data lake or data warehouses, that will take a considerable effort to implement in a paid solution. In the case of open-source connectors, some of the community-driven data connectors provide custom connector development kits that can be used to develop connectors. Although this has an overhead of development and maintenance of your side, the advantages are comparatively better.
Maintaining data quality is another important aspect of a data platform. Quality can be maintained by running transformations on top of the loaded data, that's derived from a data lake or data warehouses. Transformations are run in the form of SQL scripts on top of the data warehouses. This makes SQL skills indispensable for various teams across the. Paid data platforms allow the users to do basic as well as complex transformations using Python. In the open-source world, these transformations are driven by both SQL and Python. From a cost perspective, in both cases, computing resources will cost you because there's an additional overhead cost on paid solutions regarding the events processed which could increase your monthly bill.
Building a cloud-native data platform requires the data lakes and data warehouses to be on the cloud. So, this could be object storage, in the case of data lakes or analytical databases for building data warehouses, or even a combination of best of both worlds, now known as a Lake House. The choice of this part of the data platform is highly crucial since the whole data flow and transformations are dependent on this. So, what are the considerations that need to be validated when choosing data storage? The object storage is required to have minimal latency and a high SLA percentage. The warehouses need to be robust, durable, and scalable too. Based on the usage of the compute resources for transformations, the data warehouses need to be modified accordingly.
We are down to the last piece of the puzzle, the business intelligence part of a data platform. There are a variety of open-source tools as well as paid tools that are available. Flexibility to create apps, design dashboards, and perform roll up on the data are some of the characteristics of a good BI tool. Considering this, the open-source tools are easier to use but creating dashboards and developing better scalable visualizations can be better done using a purchased visualization tool. This could drive a huge positive impact in reaching potential customers and improving business growth.
Building versus buying data platforms can be looked at from a cost vs productivity perspective. It's always good to have a data platform that is performant enough in driving the growth and potential of the organization. Use some of these pointers to help you make the best decision for your company.
Join our WhatsApp Channel to get the latest news, exclusives and videos on WhatsApp
_____________
Disclaimer: Analytics Insight does not provide financial advice or guidance. Also note that the cryptocurrencies mentioned/listed on the website could potentially be scams, i.e. designed to induce you to invest financial resources that may be lost forever and not be recoverable once investments are made. You are responsible for conducting your own research (DYOR) before making any investments. Read more here.