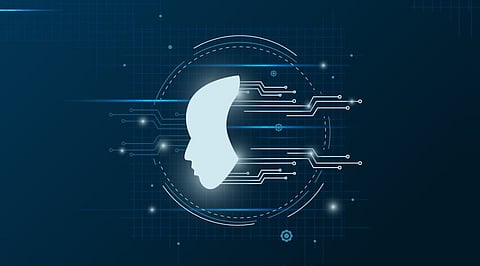
It is the science, of decision making. It is about learning the optimal behaviour in an environment to obtain maximum reward. This optimal behaviour is learned through interactions with the environment and observations of how it responds, similar to children exploring the world around them and learning the actions that help them achieve a goal.
In the absence of a supervisor, the learner must independently discover the sequence of actions that maximize the reward. This discovery process is akin to a trial-and-error search. The quality of actions is measured by not just the immediate reward they return, but also the delayed reward they might fetch. As it can learn the actions that result in eventual success in an unseen environment without the help of a supervisor, reinforcement learning is a very powerful algorithm.
IoT is already an established technology wherein multiple devices or "things" are connected across a network and they can communicate with each other. These devices are increasing continually, so much so that there might be more than 64 billion IoT devices by 2025. All these devices collect data that can be analysed and studied to obtain useful insights. That's where Machine Learning becomes so important! Machine Learning algorithms can be used to convert the data collected by IoT devices into useful actionable results reinforcing reinforcement learning.
Everyone has heard about software engineering, but now it's AI Engineering that is on the rise as a profession that comes through reinforcement learning This is a very important development because the integration of RL in the industry as it has been very ad-hoc and haphazard without any regulations of best practices.
The goal of full AutoRL is to be able to produce optimal models for new tasks, with a minimal amount of human intervention and computation time. In order to build a machine learning model, there are a number of decisions that need to be made, such as which algorithm or architecture to use and how to set the hyperparameters.
We all know that in recent years, expert-designed deep learning architectures have achieved incredible performance across a wide range of tasks from image segmentation to language generation.
RL-powered cybersecurity tools can also gather data from the company's communication networks, transactional systems, digital activity, and websites, plus external public sources, and use RL algorithms for recognizing patterns and identifying the threatening activity — such as finding out suspicious IP addresses and possible data breaches.
Join our WhatsApp Channel to get the latest news, exclusives and videos on WhatsApp
_____________
Disclaimer: Analytics Insight does not provide financial advice or guidance. Also note that the cryptocurrencies mentioned/listed on the website could potentially be scams, i.e. designed to induce you to invest financial resources that may be lost forever and not be recoverable once investments are made. You are responsible for conducting your own research (DYOR) before making any investments. Read more here.