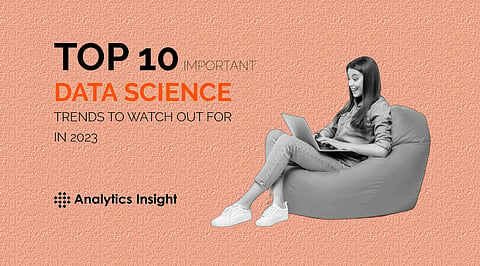
Data science is one of the IT industry's fastest-growing subsectors, and it has become an essential part of business operations.
Whether it's the healthcare sector, the financial sector, or an online retailer like Amazon, data gathering and analysis typically play a critical part in predicting the future of each new market area.
Innovations in Big Data Analytics, Data Science, and Artificial Intelligence are changing how organizations are managed all around the world and are among the top 10 data science trends for 2023. Data will thus be the differentiator if you need to tell today who won the competition and what they did differently since data is the new gold of the contemporary day.
Artificial intelligence is the technological development that will most likely influence how we live, work, and do business in the future. By using it, business analytics will be able to make more accurate predictions, spend less time on laborious processes like data collecting and cleaning, and empower employees to act on data-driven insights regardless of their position or level of technical expertise.
To address not just the chosen business possibilities but also those that would be lost due to a lack of experienced data scientists within the organization, the objective is to make data access simple, quick, and reliable for the business.
Automated machine learning is the most current advancement in data analytics, and it doesn't seem to be going anywhere anytime soon. The democratization of data science is currently being driven through automated machine learning. Automatic machine learning improves the efficiency of labor-intensive, repetitive tasks that previously required physical labor. Thanks to auto ML, data scientists are no longer concerned with time-consuming tasks like data preparation and purification.
NLP is one of the many subfields of artificial intelligence, linguistics, and computer science. Because of the available processing performance and the large quantity of data it demands, it has gained popularity in recent years.
Data governance is crucial for all aspects of data processing, analytics, and research, in other words, for any interaction between people and non-humans with data. Providing a platform for secure data exchange throughout an organization while abiding by any data security and privacy rules, such as GDPR, is the process of assuring high-quality and monitored data.
The DaaS (Data-as-a-Service) business in the healthcare industry has seen increasing opportunities since the COVID-19 pandemic first surfaced. DaaS usage is predicted to expand as more customers have access to high-speed internet.
Data fabric is the name given to a group of architectures and services that provide end-to-end functionality across a variety of endpoints and several clouds. As it is a solid architecture, it offers a common data management strategy and practicality that we can spread across a range of on-premises cloud and edge devices.
As it will flawlessly, quickly, and consistently automate tedious and repetitive processes, RPA will be a cutting-edge software technology in 2023. Both significant and difficult jobs will have time for humans.
Federated learning uses edge devices (like mobile phones) or servers to access dispersed data using machine learning techniques. Never is the initial data sent to a centralized server. It stays attached to the tool. As no one else may access the data, this technique has the advantages of data security and privacy. The localized versions of the algorithm are trained with local data. The learning outputs can then be shared with a central server to produce a "global" model or algorithm. Then, to continue learning, the edge devices can re-share data.
Generally speaking, it describes the procedure of moving digital assets, such as data, workloads, IT resources, or applications, to cloud infrastructure that is built on an on-demand, self-service environment. With the least degree of uncertainty possible, efficiency and real-time performance are the goals.
Join our WhatsApp Channel to get the latest news, exclusives and videos on WhatsApp
_____________
Disclaimer: Analytics Insight does not provide financial advice or guidance. Also note that the cryptocurrencies mentioned/listed on the website could potentially be scams, i.e. designed to induce you to invest financial resources that may be lost forever and not be recoverable once investments are made. You are responsible for conducting your own research (DYOR) before making any investments. Read more here.