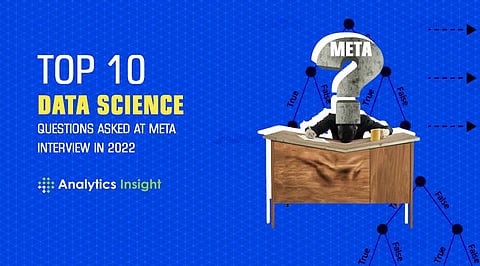
Artificial Intelligence remains at the very heart of our work across Meta, the parent of Facebook, Instagram, and WhatsApp. It would be very difficult to identify a single product that hasn't been transformed by this work. By now, Meta understands the benefit of artificial intelligence and data science. This has led to the rising demand for skilled data science professionals and created opportunities for fresher as well as experienced ones. So, here we have listed some of the most-asked data science interview questions and the answers that you need to know to start your career with Meta in 2022.
Logistic regression calculates the relationship between the dependent variable (our label of what we want to predict) and one or more independent variables (our features) by measuring probability using its underlying logistic function (sigmoid).
A random forest is built up of a number of decision trees. If you split the data into different packages and make a decision tree in each of the different groups of data, the random forest brings all those trees together.
Overfitting refers to a model that is only set for a very small amount of data and ignores the bigger picture.
There are three main methods to avoid overfitting:
Univariate data contains only one variable. The aim of the univariate analysis is to describe the data and find patterns that exist within it.
Bivariate data contains two different variables. The analysis of this type of data deals with causes and relationships and the analysis is done to determine the relationship between the two variables.
Multivariate data involves three or more variables, it is categorized under multivariate. It is the same as a bivariate but contains more than one dependent variable.
To handle missing data values, we can opt for the following process:
If the data set is large, we can simply remove the rows with missing data values. It is the fastest way; we use the rest of the data to predict the values.
For smaller data sets, we can substitute missing values with the mean or average of the rest of the data using the pandas' data frame in python. There are different ways to do so, such as df.mean(), df.fillna(mean).
Dimensionality reduction refers to the process of converting a data set with vast dimensions into data with fewer dimensions (fields) to convey similar information concisely. This reduction is useful in compressing data and minimizing storage space. It also minimizes computation time as fewer dimensions lead to less computing. It removes redundant features
Following are the steps to maintain a deployed model:
Monitor: continual monitoring of all models is required to determine their performance accuracy. When you change something, you want to figure out how your changes are going to affect things. This needs to be monitored to ensure it's doing what it's supposed to do.
Evaluate: Evaluation metrics of the current model are measured to determine if a new algorithm is required.
Compare: The new models are compared to each other to determine which model performs the best.
Rebuild: The best-performing model is rebuilt on the current state of data.
A recommender system predicts how a user would rate a specific product based on their preferences.
It can be split into two different areas:
As an example, Last.fm recommends tracks that other users with similar interests play often. This is also commonly seen on Amazon after making a purchase; customers may notice the following message accompanied by product recommendations: "Users who bought this also bought…"
For example, Pandora uses the properties of a song to recommend music with similar properties. Here, we look at content, instead of looking at who else is listening to the music.
We use the elbow method to choose k for k-means clustering. The idea of the elbow method is to run k-means clustering on the data set where 'k' is the number of clusters.
Within the sum of squares (WSS), it is defined as the sum of the squared distance between each member of the cluster and its centroid.
These are the top 10 data science questions and answers to help them in cracking Meta interviews for data science professionals.
Join our WhatsApp Channel to get the latest news, exclusives and videos on WhatsApp
_____________
Disclaimer: Analytics Insight does not provide financial advice or guidance. Also note that the cryptocurrencies mentioned/listed on the website could potentially be scams, i.e. designed to induce you to invest financial resources that may be lost forever and not be recoverable once investments are made. You are responsible for conducting your own research (DYOR) before making any investments. Read more here.