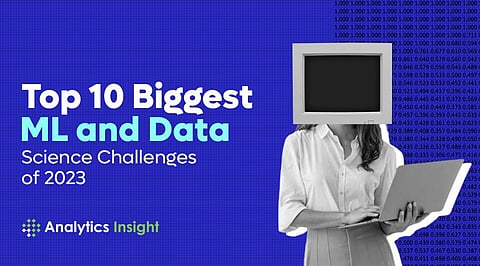
Data is the new oil for tech companies. Businesses rely upon ML and data science to gain insights into raw materials. Machine Learning algorithms can be used for understanding patterns, and learning from a given data set, all with no little programming. And data science collects, organizes, and cleans the data sets in order to solve critical business problems. But there are a few ML and data science challenges that businesses need to look upon.
Businesses need to know how to approach data science challenges. As there is too much data accessible, businesses must also understand what kind of solutions data science gives. Here we have briefed out the top 10 biggest ML and data science challenges of 2023.
Finding and collecting necessary data assets is the first step in any ML or data science project. However, the availability of suitable data is one of the major challenges that organizations face. The major issue is that organisations collect massive amounts of data without modifying it to determine whether it is useful or not. This is done to drive a general fear of missing out on key insights in the data as well as the widespread availability of low-cost data storage. Unfortunately, this only serves to clog organizations with useless data that causes more harm than good.
Companies use different kinds of tools to collect data about their sales, customers, employees etc. This can cause problems when it comes to data management and consolidation.
To stay above water and avoid being drowned by growing mounds of data, organisations require a centralised platform that can integrate their data sources and provide instant access to, organised, structured and meaningful information — potentially saving enormous amounts of money and time.
Cyberattacks have become a common problem in recent years, hence the need for securing data is becoming essential. And organisations have been granting interested parties to access their datasets because of this they are facing the additional challenge of ensuring ongoing security and compliance with data protection regulations such as GDPR. To overcome this, organizations must exercise more control over data regulations.
While many consider data preparation to be the most difficult part of any ML project, it is a critical process that ensures ML models are built on high-quality data. This eventually results in a more powerful model that can make more accurate predictions. Fortunately, many tools are now available on the market to assist ML teams in pre-processing their data by automating certain aspects of the data cleansing process. This saves ML teams a significant amount of time that they can use to develop their models.
To address the challenge of managing ever-increasing data volumes, organisations are increasingly turning to big data platforms for storage, management, cleansing, and analytics, allowing them to extract the insights that their organizations require when they need them.
Organizations frequently fail to take full ownership of their datasets, making it difficult to find the right person who can answer your questions. This issue can be solved by thoroughly documenting datasets and other data assets. That's all there to it. Thorough documentation prevents basic questions from arising repeatedly, which is a drain on resources and a waste of time.
Organizations are increasingly pushing for faster delivery and self-service reporting for extracting insights. To achieve this, they are turning to a new generation of analytics tools and platforms that can rapidly reduce the time it takes to generate insights. It also delivers real-time and high-quality insights.
Organizations frequently struggle to find the right people who have an appropriate level of knowledge and domain expertise. In addition to that, they often experience problems in helping the team function correctly.
Instead of asking all team members to handle all of these tasks, divide them up among individual team members. This promotes efficiency and allows the team to work effectively.
Data lineage is the process of comprehending, recording, and visualising data as it flows from data sources to consumption. Knowing where a dataset came from isn't always enough to fully comprehend. Identifying data lineage can show a significant impact on data migrations, data governance, and strategic data reliance.
Smaller businesses face significant challenges in assembling their ML teams — logistics, cost, expertise, etc. To overcome this, there are numerous tools and solutions on the market. These tools enable organisations to fully outsource their ML projects without jeopardising the overall quality of ML models.
Join our WhatsApp Channel to get the latest news, exclusives and videos on WhatsApp
_____________
Disclaimer: Analytics Insight does not provide financial advice or guidance. Also note that the cryptocurrencies mentioned/listed on the website could potentially be scams, i.e. designed to induce you to invest financial resources that may be lost forever and not be recoverable once investments are made. You are responsible for conducting your own research (DYOR) before making any investments. Read more here.