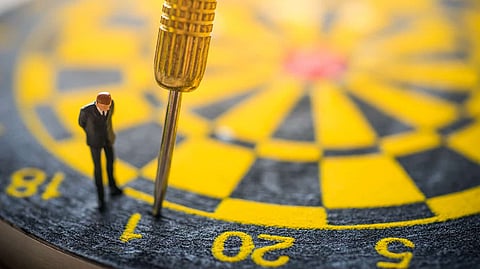
One thing the pandemic taught businesses is that digital transformation is imperative. Organizations lagging in their uptake of digital endeavors often found themselves going into overdrive to catch up as the world made massive and immediate shifts in how we worked, shopped, and communicated. And just because we're beginning to approach some level of before-COVID normality does not mean that businesses will abandon what they've started. Customers have come to expect a stellar digital experience, so organizations will need to push forward with their transformation efforts if they expect to maintain a competitive edge.
One of the most popular ways to evolve a business is by leveraging an organization's data and the insights that it can provide. The use of AI, specifically machine learning, remains a key technological trend whose promise to make work easier and more flexible has never been more relevant. The problem is that in too many cases, the adoption of machine learning stops before it even starts.
Why? Because most companies still haven't figured out how to implement machine learning on any scale. This is mostly due to fears about cost, security, compliance, and ethics as well as a generally poor understanding of exactly how machine learning can be applied and how to make sense of large amounts of data. So, the excuses pile up and the project stays incomplete. In fact, 87% of data science projects never make it into production – a modern-day Canterbury Tales that never gets across the finish line.
Four Reasons Machine Learning Fails
Too often, the adoption of machine learning doesn't get much further than initial conversations. Let's explore the four main reasons why:
1. Little understanding about how, when, and why to use it. Einstein said that out of complexity you can find simplicity. Unfortunately, when it comes to machine learning, many businesses fail to see this. Companies confused by how machine learning could be applied to their problems or even their own industry will simply avoid it altogether.
2. High price tags. Two cost-related concerns hamper machine learning deployment. The first is the cost of the technology. Not only is software expensive, sometimes reaching the high six figures, but machine learning requires high-specification infrastructure typically built on more powerful and expensive GPU resources versus common CPU-based infrastructures. The second is related to a lack of internal skill sets required to carry out machine learning and analyze the data. Whether you need data architecture, data science skills, or both, the demand is greater than supply. Qualified data scientists and other machine learning experts command high salaries.
3. The quantity and quality of data. Businesses are often quick to give up because they believe there isn't enough data to work with or what they have is just too unstructured to interpret. Yes, models need enough data to understand the question and return results with confidence and accuracy. However, the amount required depends on the purpose and complexity of the project. Many use cases need less data than you think. And while structured data is easier for machine learning because trends stand out, most businesses possess more unstructured data, such as audio, video, and document files. Because analyzing unstructured data is more labor intensive, businesses will shut down the prospect of machine learning before it even gets started.
4. Safety and ethical concerns. Because many of machine learning's popular use cases involve analyzing customer data, businesses must take time to think about data safety, including security, privacy, and governance. There are two tricky bits here: First is the requirement to adhere to privacy laws, many of which guard against automated decision-making without consent. The second is that customer data is a desirable target for hackers. Using it improperly risks exposure. Ethics is another area of concern. You not only must be able to explain decisions made around the data but also be able to ensure that data doesn't bring in any bias. Because machine learning calculations are often too complex for humans to understand or are kept tightly controlled, explaining or even knowing exactly how the technology reached its conclusion can be difficult.
While these four issues are complicated, they can be overcome. A business can start by finding a use case applicable to its industry and setting a logical set of goals for constructive and profitable deployment of machine learning. By conducting a strategic review of every process and comparing what your hard deployment costs will be alongside the proposed benefits, you can decide where machine learning can best be applied. Companies also need to assess what tools are right. Some processes need automating; others need better decision making. Then there is the issue of data. By performing a thorough data audit, you can assess what is available rather than looking at what is needed.
A cost-effective and less intrusive way to experience machine learning is to access the expertise, skills, infrastructure, and technology through a managed service. – a significantly more cost-effective option than bringing machine learning in house. An experienced managed services provider brings the specialized skills and support required to navigate the complex world of privacy, security, compliance and ethical concerns.
If machine learning projects are approached correctly and with the right partners, businesses can tackle the obstacles and reap the benefits. Although it's easier to stop a project before you really get started because of how hard it may appear, remember that no one wants to read an unfinished book.
Julian Box is the founder and chief executive officer at Calligo. Box founded Calligo in January 2012 and is responsible for delivering Calligo's vision of building a client-centric business, delivering innovative managed data services that optimize every stage of the data journey, while ensuring that privacy continues to be at the heart of the organization and its services. He has over 30 years of experience helping organizations streamline operations through the innovative application of technology. A serial start-up founder, Box has raised hundreds of millions of dollars from investors and has sold several businesses, some for over $1 billion.
Join our WhatsApp Channel to get the latest news, exclusives and videos on WhatsApp
_____________
Disclaimer: Analytics Insight does not provide financial advice or guidance. Also note that the cryptocurrencies mentioned/listed on the website could potentially be scams, i.e. designed to induce you to invest financial resources that may be lost forever and not be recoverable once investments are made. You are responsible for conducting your own research (DYOR) before making any investments. Read more here.