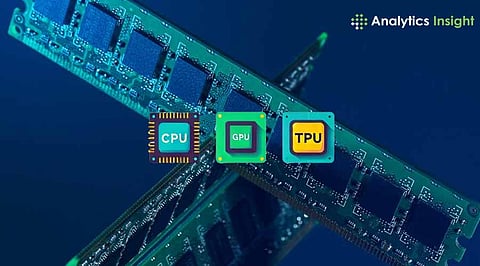
Many terms are used in today’s growing technology, such as CPU, GPU, and TPU. What these might seem to people as a collection of letters that stand for some words, all of these processing units have core tasks of processing to let computers, gaming consoles, and even AI hardware work efficiently. It’s time to understand the differences between CPU vs GPU vs TPU what these components are, why they are crucial, and how they may vary from each other.
CPU is an abbreviation for Central Processing Unit and is also called the “heart” of a computer. It is intended to carry out general-purpose computations; as such, it is simple and can adapt to various applications. This is particularly true for processes that do not require massive computing power, such as running an operating system or working with files such as Microsoft Word, Excel, etc.
CPUs are antiquated for flexibility even though they’re not the swiftest with all tasks on the board. According to recent statistics, it is possible to increase an upper-rank CPU per second up to 100 billion instructions. However, if it lacks specificity, it may not be as good for more specific tasks such as graphics rendering or machine learning.
The GPU, or Graphics Processing Unit, was initially created to handle graphics-related tasks, like rendering images in video games or editing software. While CPUs are great for a broad range of tasks, GPUs are specifically designed for parallel processing, running many calculations simultaneously. A high-end GPU can have thousands of cores, making it ideal for repetitive calculation tasks.
Today, GPUs are not just for gaming; they’re a popular choice for scientific computing and AI. Due to their structure, GPUs can process information up to 10 times faster than CPUs in some scenarios, making them perfect for tasks like video rendering, cryptocurrency mining, and training machine learning models.
TPU stands for Tensor Processing Unit, and it's the newest addition to the processing world, developed by Google specifically for machine learning. Unlike CPUs and GPUs, TPUs are tailor-made for AI hardware and deep learning tasks, especially those involving neural networks. They’re used mainly in Google’s cloud infrastructure, powering applications like image recognition and natural language processing.
TPUs excel at matrix operations, which are at the core of many machine learning algorithms. A TPU can perform these operations up to 15-30 times faster than a GPU, according to Google, making it highly efficient in its specialized domain. However, a TPU is specialized and unsuitable for general-purpose computing power or graphics tasks.
Choosing the right processor types depends on the task. A CPU does the job well for everyday computing—email, browsing, and document editing. A GPU might be more suitable for gamers, designers, or data scientists due to its ability to handle intensive graphics and parallel processing tasks. If someone is diving into deep learning and AI, a TPU might be the ideal choice, though access can be limited outside specialized environments.
CPU: Great for general-purpose tasks, handling most computer functions.
GPU: Best for graphics and parallel processing, ideal for gaming and some scientific computing.
TPU: Built specifically for AI, providing lightning-fast speeds for deep learning tasks.
Understanding each’s strengths between CPU vs GPU vs TPU makes it easier to decide which processing powerhouse is best suited for specific needs. Each has a unique role in the tech world, contributing to the daily innovation we experience.