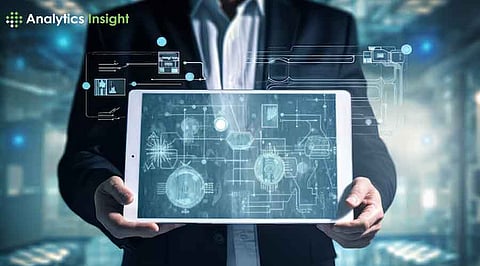
Data Mesh is going to be the revolutionary way of building scalable and efficient data platforms. Most of the traditional centralized data architectures faced situations that were quite challenging while scaling and managing the vast amount of data generated by modern organizations. Data Mesh offers the approach in a decentralized manner, instead of having the concentration on monolithic data platforms, domain-oriented, self-serve data infrastructure is the approach. This article delves into the basic trends in Data Mesh, detailing principles, advantages, and how to construct scalable data platforms.
Data Mesh is a conceptual framework developed by Zhamak Dehghani in 2019. It solves the challenges of scaling a data platform by proposing a decentralized approach, including the ownership and responsibility of the data, that is split among various domains of an organization. The model opposes the traditional architectural approaches of data relying on central data lakes or warehouses.
The core principles are listed as domain-oriented decentralization, self-serve data infrastructure, and federated computational governance. In other words, Data Mesh proposes ownership and management of the data by those teams closest to the sources of said data. This signifies that the quality, availability, and accessibility of the data products are fully in the hands of domain teams.
a. Domain-Oriented Decentralization: Data Mesh supports the concept of owned data curated by those teams closest to the data sources. This means that domain teams are responsible for the quality, availability, and accessibility of their data products.
b. Data as a Product: Data is treated as a product. It has a life cycle of its own product management, development, and maintenance. Every data product must have an owner, defined consumers, and a roadmap for enhancement.
c. Self-Serve Data Infrastructure: Besides advocating for a self-serve data infrastructure through which teams can easily access and process data for analysis without central IT teams, it has also focused on the inclusion of necessary capabilities in data discovery, data quality, and data governance.
d. Federated Computational Governance: While ownership of the data is decentralized, in the case of governance, it is federated. That simply means there is some sort of overarching principle and standard guiding the practice of data management across the organization to maintain consistency and compliance.
a. Define Clear Data Product Ownership: Clearly define ownership for each data product, including ownership of data quality, documentation, and support. This should be assigned to product managers or data stewards who will be responsible for the life cycle of data products, ensuring they meet the needs of their consumers.
b. Deploy Robust Self-Serve Data Infrastructure: Invest in self-service data tools that enable teams to access, manage, and analyze data independently. This involves investing in intuitive interfaces to explore, integrate, visualize, tap into sources of data, and process said data.
c. Focus on Data Quality and Observability: Develop and enforce the best practices for high-quality data. Establish the tools and mechanisms that monitor health, track lineage, and validate accuracy throughout the data flow. Implement metrics, alerts, and other means to proactively handle potential issues so that the data is reliable.
d. Adopt a Domain-Driven Approach: Align data management to business domains so that data initiatives can be relevant and impactful. Let domain teams own their data products, empowering them to work collaboratively across teams to effectively use integrative data across domains.
e. Establish Federated Governance Frameworks: Design governance frameworks that guide while allowing freedom. Develop organizational guidelines for data management, including standards related to privacy and security; define compliance monitoring and reporting mechanisms.
a. Increased Adoption of Domain-Driven Design: DDD has become a critical factor in the implementation of data mesh. Besides, structuring data management around business domains it also drives alignment of data initiatives with business objectives. This trend is also driving the emergence of domain-oriented data platforms where each domain team is empowered to manage their data as a product, which in turn will foster better alignment between data strategy and business needs.
b. Advancements in Data Product Management: Treating data as a product, has become a trend now. Data product management itself is getting more and more sophisticated, including product roadmaps, user feedback loops, and processes for continuous improvement. It aims to create more robust data products that have thorough documentation, are easily consumable, and are continuously refined based on user needs.
c. Enhanced Self-Serve Data Tools: Self-serve data tools are highly sought after. Organizations are investing in technologies that enable teams to serve and analyze data on their own. Data discovery, cataloging, and visualization tools become increasingly usable and integrated to drive non-technical users to take advantage of data. In this respect, it is a necessary component to make the Data Mesh as decentralized as possible.
d. Focus on Data Quality and Observability: This trend ensures data quality and observability, with data ownership, becoming ever more decentralized. Organizations are adopting this trend of practices for monitoring of data quality and lineage tracking, with automation in validating data. It maintains the trustworthiness and reliability of data products to be maintained across various domains.
e. Cloud-Native Technology Integration: Data Mesh increasingly integrates cloud-native technologies. Cloud platforms provide scalable and flexible infrastructures that go well with the decentralized principles of Data Mesh. Data lakes, serverless computing, and managed data services are some of the services used to natively build and manage data products in the cloud.
Data Mesh represents a significant shift in how organizations perceive data management, placing strong emphasis on decentralization, domain ownership, and self-serve infrastructure. As the field continues to evolve, staying updated with the corresponding trends and best practices will be necessary to develop scalable and effective data platforms.
By utilizing the principles of data mesh, including domain-driven design, self-serve tools, and federated governance into activity, organizations can deliver new levels of agility, innovation, and data-driven decision-making. With greater traction, data mesh will change the way organizations harness and manage their data assets, leading the way to data platforms that are increasingly more resilient and scalable in the years ahead.
1. What is Data Mesh?
A: Data Mesh is a decentralized approach to data architecture where data ownership and management are distributed across domain teams, promoting scalability and alignment with business objectives.
2. How does Data Mesh differ from traditional data architectures?
A: Unlike traditional centralized data architectures, Data Mesh distributes data ownership across domains, treating data as a product and enabling self-serve data infrastructure.
3. What are the key principles of Data Mesh?
A: The key principles include Domain-Oriented Decentralization, Data as a Product, Self-Serve Data Infrastructure, and Federated Computational Governance.
4. Why is Domain-Driven Design important in Data Mesh?
A: Domain-driven design aligns data management with business domains, ensuring that data initiatives are relevant and effectively support business goals.
5. What are the benefits of implementing a self-serve data infrastructure?
A: A self-serve data infrastructure empowers teams to independently access, analyze, and manage data, reducing reliance on central IT and accelerating data-driven decision-making.