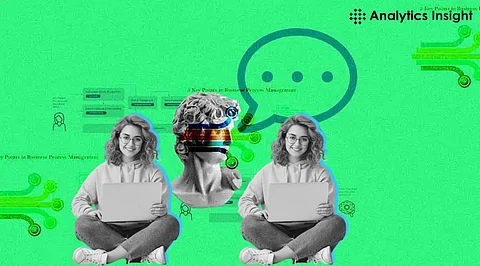
In 2024, the coordination of SQL (Organized Question Language) in managing Machine Learning undertakings has arisen as a basic part of the information science and examination scene. SQL, a standard language for overseeing and controlling social information bases, offers a hearty structure for dealing with information preprocessing, highlight designing, model preparation, and assessment, consequently smoothing out the ML work process and improving proficiency and versatility.
SQL assumes a critical part in information readiness and investigation, permitting information researchers to separate, change, and burden (ETL) information from different sources into organized data sets. With SQL inquiries, examiners can perform information purifying, accumulation, sifting, and testing activities to guarantee information quality and uprightness prior to taking care of it into ML models. Moreover, SQL empowers exploratory information examination (EDA) by working with elucidating measurements, perception, and speculation testing to acquire experiences into the basic information dissemination and examples.
Highlight designing is a pivotal move toward ML model turn of events, including the making of instructive elements from crude information to work on prescient execution. SQL gives amazing assets to highlight age and change, empowering information researchers to determine new elements, encode absolute factors, standardize mathematical elements, and handle missing qualities proficiently. By utilizing SQL's abilities, examiners can carry out complex element designing pipelines straightforwardly inside the data set climate, speeding up the iterative course of model turn of events and refinement.
SQL can be incorporated consistently with ML structures and libraries to perform model preparation and assessment assignments straightforwardly inside the information base climate. With SQL-based AI expansions, for example, SQL Server AI Administrations, Prophet AI, and PostgreSQL's PL/Python, information researchers can execute ML calculations, train prescient models, and survey model execution utilizing natural SQL linguistic structure. This mix improves on the organization and versatility of ML work processes, as models can use the computational assets of the data set framework for parallelized handling and effective execution.
Underway conditions, SQL empowers continuous forecasts and derivation by inserting ML models straightforwardly into SQL inquiries or put away techniques. With methods, for example, model scoring capabilities and client characterized capabilities (UDFs), associations can convey ML models inside their current data set foundation to perform on-the-fly expectations, suggestions, and dynamic assignments because of client questions or occasions. This approach guarantees low-inactivity, high-throughput handling of prescient assignments, making it reasonable for applications calling for continuous bits of knowledge and responsiveness.
One of the critical benefits of involving SQL for managing ML undertakings is its adaptability and execution. By bridling the dispersed registering abilities of cloud-based data sets or appropriated handling structures like Apache Flash, associations can scale their ML pipelines to process gigantic datasets and oblige developing responsibilities easily.
SQL-based ML work processes work with administration, consistence, and auditability by giving discernibility and straightforwardness into information handling and model results. With SQL questions filling in as executable documentation of information changes, highlight designing advances, and model preparation methodology, associations can keep up with administrative consistence, comply to information security guidelines, and guarantee reproducibility and responsibility in ML tasks. Also, SQL-based admittance controls and job based consents empower granular security strategies to safeguard delicate information and alleviate online protection gambles.
The coordination of SQL in managing ML undertakings offers a strong structure for start to finish information handling, model turn of events, and organization in 2024. By utilizing SQL's capacities for information arrangement, include designing, model preparation, and ongoing derivation, associations can smooth out their ML work processes, improve adaptability and execution, guarantee administration and consistence, and open noteworthy bits of knowledge from their information resources.
Join our WhatsApp Channel to get the latest news, exclusives and videos on WhatsApp
_____________
Disclaimer: Analytics Insight does not provide financial advice or guidance. Also note that the cryptocurrencies mentioned/listed on the website could potentially be scams, i.e. designed to induce you to invest financial resources that may be lost forever and not be recoverable once investments are made. You are responsible for conducting your own research (DYOR) before making any investments. Read more here.