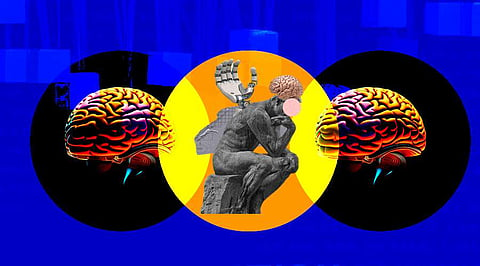
An approach for evaluating the Design Parameters of large language models (LLMs) as actual agents was developed by almost two dozen researchers from Tsinghua University, Ohio State University, and the University of California at Berkeley.
These models are frequently judged by how well they perform on tests written in plain English for humans or by how effectively they write content that is thought to be human-like. In comparison, there has been far little study on the subject of LLM models acting.
Agents with artificial intelligence (AI) carry out specified duties, such as adhering to a set of guidelines while operating in a particular setting. Researchers, for instance, commonly train an AI agent to navigate a difficult digital environment to evaluate how machine learning may be applied to create safe autonomous robots.
Due to the high expenses associated with training models like ChatGPT and Claude, conventional machine learning agents like the one in the aforementioned video are not frequently developed as LLMs. The biggest LLMs have, nevertheless, demonstrated potential as agents.
The team from Tsinghua, Ohio State, and UC Berkeley developed AgentBench, which they claim is the first tool of its type, to evaluate and appraise the capabilities of LLM models as genuine agents.
The key hurdle in designing AgentBench, according to the researchers' preprint article, was moving beyond conventional AI learning settings, such as video games and physics simulations, and figuring out how to apply LLM capabilities to real-world issues so they could be successfully tested.
They developed a series of tests that are multidimensional and gauge a model's capacity for difficult work in many settings.
These include having models carry out operations in a SQL database, utilizing an operating system, organizing and carrying out domestic cleaning activities, making online purchases, and several other high-level tasks that demand methodical problem-solving.
Even while they acknowledged that "top LLMs are becoming capable of tackling complex real-world missions," the researchers emphasized that open-sourced rivals still have a "long way to go."
Join our WhatsApp Channel to get the latest news, exclusives and videos on WhatsApp
_____________
Disclaimer: Analytics Insight does not provide financial advice or guidance. Also note that the cryptocurrencies mentioned/listed on the website could potentially be scams, i.e. designed to induce you to invest financial resources that may be lost forever and not be recoverable once investments are made. You are responsible for conducting your own research (DYOR) before making any investments. Read more here.