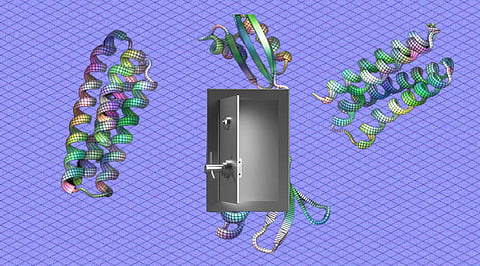
Making a protein is similar to building a cabinet. The process of constructing the protein's skeleton starts first. The tricky aspect is determining the ideal "hotspots" to attach hinges to the structure in order to attach doors, shelves, and other components that will finally give the cabinet its complete functionality.
In a sense, the architectures of proteins contain hotspots as well. These fascinating crevices and crannies create elaborate docks for other proteins or medications to cling to, living up to their designation of "functional sites." The majority of our fundamental biological activities are carried out at the sites. They are also a veritable gold mine for developing novel medications and medical treatments.
What is the problem? Mapping functional locations are challenging. To identify specific binding sites, researchers have historically had to mutationally alter each probable region of a protein, exchanging one amino acid for another. It's really tiresome, like a detective going through hundreds of suspects—and there may be many.
The entire rulebook was overthrown by a recent Science study. A team at the University of Washington, under the direction of Dr. David Baker, used an AI's "imagination" to create a variety of useful sites from scratch. A deep learning system that forecasts the general location of a protein's functional domain but then further shapes the structure exhibits the "creativity" of the system mind at its best.
The scientists used the new software to create vaccines against common, if occasionally fatal, infections and medications that fight cancer as a reality check. In one instance, an idea was generated by the digital mind that, when evaluated in isolated cells, matched an existing antibody perfectly against a common pathogen. To put it another way, the algorithm "created" a hotspot from a viral protein, leaving it open to attack by drug designers.
The method is the first attempt at creating proteins around their functions via deep learning, opening the door to therapies that were previously unthinkable. However, the software is not just for finding natural protein hotspots. In a press release, Baker stated, "The proteins we discover in nature are great molecules, but engineered proteins can do so much more." "Things that none of us expected it would be capable of," the algorithm is "performing."
The Baker team has experience using artificial intelligence to predict proteins. They just released Rosetta, a program that can predict a protein's 3D structure based solely on its amino acid sequence, and it completely revolutionised the discipline of structural biology. Additionally, they mapped protein structures and created entirely new protein "screwdrivers" to disassemble unfavourable protein interactions. Late last year, they unveiled trRosetta, a deep learning network that generalises how chains of amino acids assemble themselves into complex structures at the nanoscale.
The Baker lab has experience predicting proteins using artificial intelligence. Recently, a tool called Rosetta that predicts a protein's three-dimensional structure purely from its amino acid sequence radically revolutionised the field of structural biology. In order to break up undesirable protein connections, they also mapped protein structures and developed brand-new protein "screwdrivers." They debuted trRosetta around the end of last year, a deep learning system that extrapolates how chains of amino acids put themselves together to form intricate structures at the nanoscale. Should you engage a potential intruder or retreat? In other words, proteins are the fundamental components of life, and understanding their structure allows us to gain access to it.
The truth is that not every component of a protein is made equal. Functional areas are the "hands" of a protein, which can grip onto other proteins or drugs, trigger enzymatic activities, or fend off invading infections. These sites are hard to identify and much harder to duplicate since they are built right into the structure of the protein.
Is it conceivable for a computer to imagine an amino acid chain that naturally folds into a functioning site given some prior knowledge? This was the question that the current study attempted to answer using a version of Rosetta.
The issue might appear strange, yet there is an earlier instance in a different field. OpenAI produced a variety of images from just text captions by using a neural network. The DALLE algorithm, a derivative of the famous AI text generator GPT-3, used straightforward text prompts to produce fantastical but convincing-looking graphics by recognising patterns from its learning. After the tool's original release, Dr. Hany Farid of UC Berkeley remarked that it "takes the deepest, darkest regions of your imagination and converts it into something that is strangely pertinent."
Join our WhatsApp Channel to get the latest news, exclusives and videos on WhatsApp
_____________
Disclaimer: Analytics Insight does not provide financial advice or guidance. Also note that the cryptocurrencies mentioned/listed on the website could potentially be scams, i.e. designed to induce you to invest financial resources that may be lost forever and not be recoverable once investments are made. You are responsible for conducting your own research (DYOR) before making any investments. Read more here.