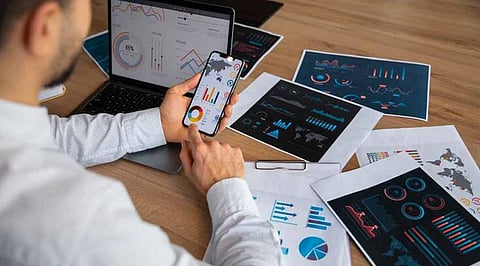
Have you noticed that markets move at lightning speed? So businesses can't afford to rely on guesswork. Predictive analytics acts quickly and proactively. It involves the use of data, statistical algorithms, and machine learning techniques to determine the probability of future outcomes based on historical data. With the right infrastructure, such as a dedicated server, companies can efficiently process large datasets and run complex analytics seamlessly.
Simply put, it doesn't just analyze what happened yesterday, it predicts what will happen tomorrow.
Now think about the benefits a company can get from this: At the very least, the business will stay one step ahead. Otherwise, a proactive approach allows companies to adapt their strategies, optimize inventory, and launch products at the right time.
Predictive analytics relies on a variety of data sources. Each type of data has unique insights to offer, and this helps businesses make more informed decisions.
Here are some key examples of such data sources:
Historical sales data
Social media data (Twitter, Facebook, Instagram, etc.)
Data on customer behavior
Economic data
Competitor analysis data
Combining these data sources allows companies to create a comprehensive view of the market and makes their forecasting models more accurate.
Predictive analytics uses several methods to turn raw data into actionable insights.
The methods include:
These are algorithms that learn from data patterns and improve over time. Popular algorithms include decision trees, neural networks, and more.
Regression methods such as linear and logistic regression help predict future outcomes by analyzing the relationship between variables. They can be used to predict sales or demand based on factors such as price, marketing costs, or customer demographics.
This method is ideal for predicting future values based on previous observations of data over time. It is commonly used to forecast demand, predict stock prices, and identify seasonal trends.
Various tools and platforms are available for companies that want to implement these methods:
Dedicated servers from Hostzealot (powerful computing power and data processing capabilities + reliable hosting solution)
Python & R (data analysis and predictive modeling)
Tableau (integration of predictive analytics)
Implementing predictive analytics involves several important steps. Each of these steps plays a vital role in predicting market trends and turning insights into actionable strategies.
Let's take a look at the steps:
This can be historical sales records, customer behavior data, social media trends, or industry reports. The quality and variety of the data collected will have a significant impact on the accuracy of the forecasts.
This step involves removing duplicates, handling missing values, and standardizing formats. Data cleaning ensures that the dataset is accurate and reliable, providing a solid foundation for model building.
Depending on the goals, businesses can choose machine learning algorithms, regression analysis, or time series forecasting. The model is then trained on historical data to learn patterns and make predictions.
This step helps to identify potential problems with over- or under-fitting and to refine the model for better performance.
After validation, the results of the predictive model should be used to formulate business strategies. For example, if the model predicts a surge in demand for a particular product, the company can adjust inventory levels or optimize marketing efforts.
In addition to being a powerful tool, predictive analytics can also be associated with certain challenges. But where there are problems there are also solutions.
For example:
Poor data quality, such as incomplete, outdated, or inconsistent information, can lead to unreliable predictions. Companies should invest time in data cleaning and validation to ensure that their data is accurate and ready for analysis.
It is called overfitting when a model is overly complex and captures noise rather than real patterns. Regularly testing and improving models can help avoid this pitfall.
It can be difficult to turn findings into practical solutions. Companies should ensure that insights are communicated to decision-makers and used for strategic planning.
When dealing with large amounts of data, especially customer-related information, companies must comply with data protection regulations.
The following practices can help with effective predictive analytics:
Ensure high data quality
Use a variety of data sources
Regularly test and update models
Use the right infrastructure
Integrate predictive insights into decision-making processes
So, predictive analytics not only helps to optimize inventory but also improves customer experience and sales forecasting.
To maximize the potential of predictive analytics, you need the right infrastructure. Using supporting tools such as dedicated servers ensures that companies have the necessary resources to grow. This robust setup provides the stability needed for continuous data analysis, allowing companies to confidently implement predictive strategies.
Ultimately, implementing predictive analytics and leveraging dedicated servers provides companies with the tools they need to make smarter decisions, drive growth, and stay competitive.