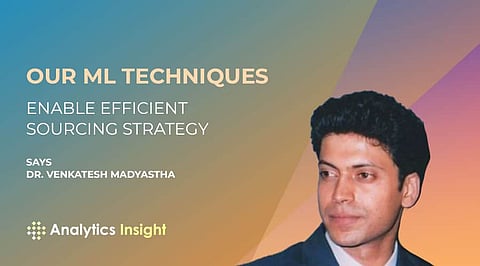
Lending & savings has far moved out from the shadow of the traditional banking sector. Digital platforms are offering a tranche of innovative products which could have been hardly imagined a few years ago. Thanks to cutting-edge technologies such as artificial intelligence and big data, online platforms are finding it safe to innovate with financial tools to serve clients better. MyShubhLife, an instant personal loan platform exactly falls into this category. Leveraging ML-based ensemble approaches, they can accurately predict repayment and default rates. Analytics Insight has engaged in an exclusive interview with Dr Venkatesh Madyastha, Chief Data Scientist, of MyShubhLife.
We are India's next-gen digital lending and saving platform. We believe everyone deserves a chance to attain financial freedom and it is with this goal we have developed exclusive data-science models to make credit accessible to millions of borrowers. Our lending model uses advanced machine learning [ML] based algorithms and in-house feature selection modules to identify low risk borrowers. Additionally, we have an Early Warning System [EWS] that monitors borrowers monthly EMI repayments, once the loan is disbursed. Our proprietary EWS uses advanced ML-based ensemble approaches to predict whether the borrower is likely to: (a) bounce, (b) bounce but pay during the month, or, (c) not pay during the month. Furthermore, in the case of (b) our models are precise and robust enough to accurately predict the week of the month that the repayment is likely to be made. Our EWS model maps the customers' probability of default through a colour code system, thereby ensuring that our collections team can better prioritize their actions.
When it comes to digital lending, some of the key trends that are emerging from Fintechs in the space of AI/ML/DL include:
In Big Data Analytics, predictive analysis is a key trend – such methods are implemented to examine modern data and historical events to know the patterns customers usually display. Predictive analysis helps in calculating the borrowers' next steps and strategizing accordingly thus enabling organizations like ours to arrive at steps taken to identify a borrower's future move. Furthermore, many Fintech companies are using advancements in Machine Learning [ML] to learn from borrowers and market trends in order to create different intelligent outcomes, which lead to enhanced accuracy, decision-making, and efficiency in data processing. New trends in ML are enabling users to track user-defined log-based metrics and search massive data sets for criteria that provide them with the desired business outcome.
With the influence of ML, we are now on the verge of a significant digital revolution that will benefit customers while also reducing operating costs and increasing wallet share for Fintechs. The adoption of technologies here is primarily driven by shifting customer behaviour in favour of higher-value digital lending services and mobile applications. Thanks to this data driven approach, customers can connect with Fintechs on their own terms, and receive financial support or guidance as needed and how and when they want it. It will be a creative combination of technology, people, and products that will redefine the next generation of customers for Fintechs. CIOs and leaders will have to keep abreast with the latest technology. It's important for leaders and CIO's of today to keep themselves abreast with current trends in data science, while encouraging futuristic ideas driven by technological advancements in the fintech business.
The roadmap for the rest of the year and 2023 looks interestingly positioned for the application of Deep Learning (DL) methodologies towards credit risk modelling. The bureau history for a customer is rich in information about the customer's financial health, which we believe can be explored in its entirety by the application of relevant DL techniques. We also believe that AI and ML can help us source customers more efficiently via intelligent digital marketing. To this end, we are planning to deploy our in-house marketing model which is once again based on ML techniques. This model provides an efficient sourcing strategy for reaching out to new customers via SMS based on region. It also gives us indications of what the SMS language & length should be and what's the best time to send the message. Additionally, verification and validation of existing models for credit risk as well as for EWS is a monthly exercise, which we intend to continue with the same fervour. Based on the performance accuracy of our models, these will either be re-tuned, re-designed or re-engineered in the coming year.
We have all the ingredients to be a digital bank for the next half billion by 2026. Looking at a growth rate of 13x, this credit segment is poised for growth from $25 billion to $335 billion, MyShubhLife is at the forefront of this change.
The senior leadership at MyShubhLife comes with a combination of vast and rich experience in banking, technology and data science. This enables a healthy handshake between various departments such as risk, marketing etc. with the Data Science department that helps drive the technology. Additionally, all the departments boast passionate and self-driven team members who have thorough experience in their specific areas such as risk, marketing, and data science, which are pretty much the foundation of MSL. The synergy between the leadership and the teams working at MSL ensures that the models and methods are a hybrid of data and experience.
Various latest technologies like Artificial Intelligence (AI), Machine Learning, Neural Networks, evolutionary algorithms, and Big Data Analytics, have allowed computers to crunch varied, diverse and deep datasets than ever before. AI enables a customer-centric approach, cost optimization, enhanced security, and real-time data integration which are crucial to the business model of lending firms like ours.
Although unpublished so far, our innovations primarily are in the areas of credit risk modelling, early warning and intelligent digital sourcing via marketing. Our credit risk model is separate for existing credit customers and new to formal credit customers. The most recent enhancement of our credit risk Model is an ensemble model that looks at early, midterm and long-term indicators to compute a risk band for customers. Our early warning system is also an ensemble that is capable of not only identifying problematic customers on a monthly basis but also of indicating typical payment dates within the month. Our marketing model is designed to source new customers efficiently and intelligently via SMS campaigns and adapt the campaign to specific regions of the country as well as specific days of the month. Additionally, we have also developed a smart underwriter statistical tool that can compute the current obligations of customers without manual intervention. All these innovations are happening in the AI/ML/DL Analytics space at MyShubhLife.
Join our WhatsApp Channel to get the latest news, exclusives and videos on WhatsApp
_____________
Disclaimer: Analytics Insight does not provide financial advice or guidance. Also note that the cryptocurrencies mentioned/listed on the website could potentially be scams, i.e. designed to induce you to invest financial resources that may be lost forever and not be recoverable once investments are made. You are responsible for conducting your own research (DYOR) before making any investments. Read more here.