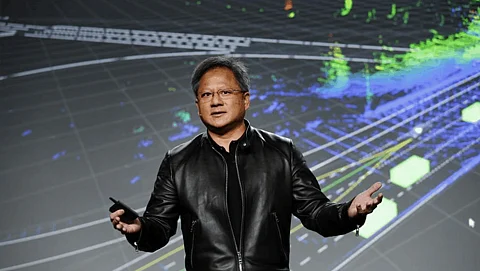
MLOps or Machine Learning Operations is considered as the next generation of DevOps with enhanced capabilities of artificial intelligence (AI). MLOps applies DevOps principles as it itself is a software system. However, separately they mean:
MLOps is a set of techniques and practices used by data scientists to work along with operations teams. Through this, they can manage the deployment of ML and Deep Learning models to pull off large scale productions. Whereas, DevOps refers to developing and operating large-scale software systems that aim to reduce the development cycles while increasing speed of deployment and create dependable releases.
As the products of today are getting more diverse in their servings, their design has to be efficiently diverse and productive. This calls for an extremely proficient data engineering to transform quintessential data in a form easily utilized by machine learning algorithms. To achieve this, it is necessary to establish an equilibrium between data engineering and data science, and hence IT Operations and data science. Here, MLOps comes into the picture that tends to enhance and eventually complete the life cycle of an ML developer.
In short and crisp, the evolving market need and diversification at different verticals have mandated the incorporation of DevOps for machine learning or MLOps.
And understanding this need, one of the leading tech companies, NVIDIA has embarked its journey into MLOps. The company has developed a product named NGC which provides a fully managed registry of optimized containers designed to run any framework one needs. NVIDIA's NGC Registry is used by data scientists to employ all the tools they need on any computing resource in just one step. By launching any machine learning or deep learning framework in minutes through NGC they can exceedingly improve MLOps.
Tony Paikeday, director of product marketing for the NVIDIA DGX portfolio of AI supercomputers and NVIDIA accelerated data science platform, in an interview said, "Wherever enterprises opt to run their training models, companies quickly need to figure out how to operationalize their data science. One problem is that data scientists tend not to be trained engineers, and don't necessarily follow good DevOps practices. Worse, data scientists, engineers, and IT operations often work in isolation. All of this contributes to making AI brittle and immature within the enterprise."
According to him, the Holy Grail, at least to some, is MLOps, a bringing together of AI and Operations, similar to what has been done between Development and Operations (DevOps). As noted by Kyle Gallatin, an ML engineer at Pfizer, the goals of MLOps include reducing the time and difficulty to push models into production, reducing friction between teams and enhance collaboration, improving model tracking, versioning, monitoring, and management, creating a truly cyclical lifecycle for the modern ML model and standardizing the machine learning process to prepare for increasing regulation and policy.
NVIDIA, said Paikeday, is building a platform that allows data scientists to work closely with DevOps folks and thus reduce the friction between these sparring groups. The company, besides being a GPU-based firm, is even more focused today on building the software that will serve as connective tissue for data scientists and DevOps within the enterprise so that AI moves from artisanal to industrial.
Join our WhatsApp Channel to get the latest news, exclusives and videos on WhatsApp
_____________
Disclaimer: Analytics Insight does not provide financial advice or guidance. Also note that the cryptocurrencies mentioned/listed on the website could potentially be scams, i.e. designed to induce you to invest financial resources that may be lost forever and not be recoverable once investments are made. You are responsible for conducting your own research (DYOR) before making any investments. Read more here.