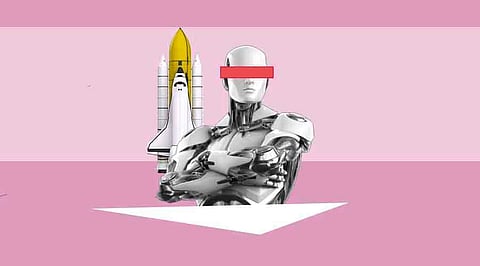
In today's fast-paced industrial landscape, the importance of predictive maintenance cannot be overstated. Traditional, reactive maintenance approaches often lead to costly downtime, inefficient operations, and preventable breakdowns. This is where machine learning (ML) powered startups are stepping in to revolutionize the field of maintenance. By leveraging the power of data analytics, sensors, and artificial intelligence, these innovative companies are enabling organizations to predict equipment failures, optimize maintenance schedules, and ultimately save significant time and resources.
Predictive maintenance is the practice of using data, sensor technology, and advanced analytics to predict when a piece of equipment or machinery is likely to fail. By identifying issues before they occur, organizations can perform maintenance tasks proactively, avoiding unplanned downtime and reducing maintenance costs. Traditional maintenance strategies typically fall into two categories: reactive and preventative. Reactive maintenance involves fixing equipment only after it has already failed, leading to costly repairs, lost productivity, and potential safety hazards. Preventative maintenance, on the other hand, is based on a schedule, which can lead to unnecessary maintenance and wasted resources, as maintenance is performed regardless of the actual condition of the equipment.
ML-powered startups are capitalizing on the vast amounts of data generated by industrial equipment to develop sophisticated predictive maintenance solutions. Here are some key innovations they bring to the table:
Data Integration: These startups employ data integration platforms that gather and consolidate data from various sources, such as sensors, IoT devices, and historical maintenance records. This consolidated data serves as the foundation for predictive models.
Machine Learning Algorithms: Machine learning models, including neural networks, decision trees, and random forests, are used to analyze the integrated data. These algorithms can detect patterns and anomalies that may indicate impending equipment failures.
Predictive Models: ML-powered startups create predictive models that provide insights into equipment health. These models can estimate the remaining useful life of assets and predict when maintenance should be performed. By doing so, they help organizations reduce downtime and optimize maintenance schedules.
Real-time Monitoring: These startups often offer real-time monitoring solutions, allowing organizations to receive instant alerts and notifications when issues are detected, ensuring rapid response and minimal disruption.
Customization: ML-powered solutions can be tailored to specific industries and equipment, allowing for highly accurate predictions. Whether it's manufacturing, energy, aviation, or healthcare, these startups can adapt their models to suit various needs.
The adoption of ML-powered predictive maintenance brings a multitude of benefits to organizations:
Cost Reduction: By predicting equipment failures and performing maintenance only when necessary, companies can significantly reduce operational costs.
Increased Efficiency: With real-time monitoring and optimized maintenance schedules, equipment uptime is maximized, leading to improved overall efficiency.
Enhanced Safety: Preventing equipment failures before they happen reduces the risk of accidents and ensures a safer working environment.
Improved Sustainability: Efficient maintenance practices not only save costs but also reduce the environmental footprint by minimizing the need for spare parts and resources.
Competitive Advantage: Organizations that implement ML-powered predictive maintenance gain a competitive edge by reducing downtime, increasing productivity, and enhancing their reputation for reliability.
Several startups have already made substantial contributions to the field of predictive maintenance. For instance, Uptake, an industrial AI company, has partnered with major players in various industries to deliver predictive maintenance solutions, helping customers save millions in maintenance costs and avoid unplanned downtime. In the aviation sector, Neuraspace uses machine learning to predict component failures in aircraft, allowing airlines to optimize maintenance, reduce delays, and improve passenger safety.
As industries become increasingly reliant on technology and data, ML-powered startups are at the forefront of predictive maintenance innovations. By harnessing the power of machine learning, data analytics, and real-time monitoring, these companies are helping organizations proactively address maintenance needs, reduce costs, enhance efficiency, and gain a competitive edge. In the ever-evolving landscape of industrial maintenance, these startups are proving to be invaluable partners for businesses looking to thrive in a data-driven world.
Join our WhatsApp Channel to get the latest news, exclusives and videos on WhatsApp
_____________
Disclaimer: Analytics Insight does not provide financial advice or guidance. Also note that the cryptocurrencies mentioned/listed on the website could potentially be scams, i.e. designed to induce you to invest financial resources that may be lost forever and not be recoverable once investments are made. You are responsible for conducting your own research (DYOR) before making any investments. Read more here.