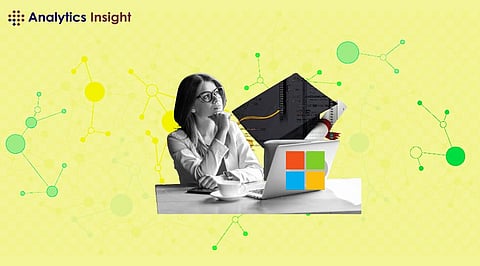
In the realm of data science, the ability to efficiently manipulate and analyze data is paramount. As we delve deeper into 2024, one of the most powerful tools at a data scientist's disposal remains window functions. These functions offer a versatile and efficient way to perform complex analytical tasks within datasets, making them indispensable in the modern data science toolkit.
Window functions, also known as windowing or analytic functions, operate on a set of rows related to the current row within a dataset. They allow for computations across a specified range of rows, enabling advanced data manipulation and aggregation without the need for complex joins or subqueries. In essence, window functions provide a window into the dataset, empowering data scientists to extract valuable insights with ease.
One of the key advantages of window functions is their ability to partition data into distinct subsets based on specified criteria. This partitioning enables precise control over how computations are performed, allowing for tailored analysis of different segments within the dataset. Whether it's calculating moving averages, ranking data based on specific attributes, or identifying outliers within a time series, window functions offer a comprehensive solution for a wide range of analytical tasks.
Staying ahead of the curve is essential. Mastering window functions not only streamlines your data analysis workflow but also opens up new avenues for exploration and discovery. Let's delve into some of the ways you can leverage window functions to elevate your data science skills in 2024.
1. Enhanced Data Aggregation: Window functions excel in performing aggregate calculations across sliding windows of data. Whether you're computing rolling averages, cumulative sums, or running totals, window functions offer a streamlined approach to data aggregation. By specifying the appropriate window frame and partitioning criteria, you can gain valuable insights into trends and patterns within your dataset, facilitating informed decision-making and analysis.
2. Precise Ranking and Ordering: Sorting and ranking data is a common task in data science, especially when dealing with large datasets. Window functions provide robust capabilities for ranking and ordering data based on specified criteria, such as numerical values or timestamps. Whether you're identifying top-performing assets in a financial portfolio or prioritizing customer segments based on revenue generation, window functions offer a flexible and efficient way to rank data with precision.
3. ime-Series Analysis: Time-series data is ubiquitous in various domains, including finance, healthcare, and IoT. Window functions play a crucial role in time-series analysis by enabling computations across temporal windows of data. Whether you're analyzing stock prices, monitoring sensor data, or forecasting demand, window functions offer powerful tools for deriving actionable insights from time-varying datasets. From calculating moving averages to detecting anomalies, window functions empower data scientists to extract valuable signals from temporal data streams.
4. Advanced Statistical Analysis: Beyond basic aggregations and rankings, window functions facilitate advanced statistical analysis within datasets. Whether you're computing percentile ranks, quantiles, or statistical outliers, window functions offer a rich set of analytical capabilities for exploring data distributions and patterns. By combining window functions with statistical techniques such as regression analysis or hypothesis testing, data scientists can gain deeper insights into the underlying dynamics of their data, uncovering hidden relationships and trends.
5. Seamless Integration with SQL and Data Analytics Platforms: One of the notable advantages of Windows functions is their seamless integration with SQL-based databases and data analytics platforms. Whether you're using popular SQL databases like PostgreSQL, MySQL, or Apache Spark for big data processing, window functions are supported natively, allowing for efficient execution of analytical queries. This integration simplifies the adoption of window functions within existing workflows, enabling data scientists to leverage their full potential without the need for specialized tools or frameworks.
Conclusion:
Mastering window functions is essential for data scientists seeking to elevate their skills and stay ahead in the rapidly evolving field of data science. By harnessing the power of window functions, data scientists can perform advanced data manipulation, aggregation, and analysis with ease, unlocking new insights and opportunities within their datasets.
Join our WhatsApp Channel to get the latest news, exclusives and videos on WhatsApp
_____________
Disclaimer: Analytics Insight does not provide financial advice or guidance. Also note that the cryptocurrencies mentioned/listed on the website could potentially be scams, i.e. designed to induce you to invest financial resources that may be lost forever and not be recoverable once investments are made. You are responsible for conducting your own research (DYOR) before making any investments. Read more here.