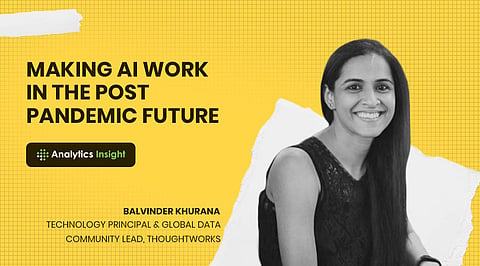
"Every time we figure out a piece of it, it stops being magical; we say, 'Oh, that's just a computation,'" said Rodney Brooks, the director of MIT's Artificial Intelligence Laboratory, twenty years ago about AI. In the two decades since, this has only become truer. There is some level of AI in every aspect of our lives — from online shopping, healthcare and news to even enhanced virtual backgrounds in our meetings. The more omnipresent AI is, the more invisible it becomes.
Given the pandemic accelerated the need for organizations to be smarter, quicker, more agile, AI is beginning to play a more integrated role in business success. Not just businesses, even governments, not-for-profits, and communities are seeking to leverage AI for their needs. Today, our customers are exploring a wide range of applications for AI/ML: from AI-generated whiskey to building solutions for retail on the Open Network for Digital Commerce (ONDC).
This has brought about some key changes to the way we've been approaching AI. For starters, the idea of analytics and reporting is becoming obsolete. Organizations today are seeking teams that automate analytics and build dynamic insights and action. They are willing to pay for useful data, fueling growth among third-party data aggregators.
There are concerns too, though. The biggest business concern is in driving value from AI projects. Many large organizations have tried big AI projects and failed, making them more cautious about experimentation. Issues of ethics are rising across the globe, forcing businesses to consider explainability, interpretability, and causality. In fact, this is also extending to AI efficiency and using as little compute and data as possible to minimize environmental impact.
With so much to think about, building sustainable AI solutions can be overwhelming for most enterprises. But it doesn't have to be. From our experience working with some of the leading enterprises in the world, we've built an approach to successful AI applications. Here are what drives them.
Finding the right problem to solve. When there are large volumes of data, a wide range of insights are to be expected. But not all observations are business problems to be solved — in fact, some of them are not problems at all. The most important thing about leveraging AI is to find and prioritize the right problems that are both feasible and impactful in achieving business goals.
Finding the right solution for the problem. Seasoned technologists know that AI is a tool, not a magic wand. This means that every problem has possible AI and non-AI solutions. Instead of adopting AI for its own sake, we must thoroughly and honestly evaluate if that's the best solution for the problem at hand. An unwavering focus on business metrics helps make these decisions simpler.
Making the most of data. In academia, the trend is shifting towards 'foundation models' — models that are trained in a task-agonistic way adaptable to any number of applications. This is likely to be adopted by the industry as well, especially those facing challenges in accessing good quality data in large amounts. Creating small but good data sets might lead to better and more accurate models that require smaller compute.
Building failsafe. As an evolving tech, there is a lot that could go wrong with AI. So, it is crucial at this point that we also learn about the cost of error or inaccuracy in the AI solution we are proposing. Testing this solution with a sample of users and obtaining feedback helps us ensure that we learn early and evolve quickly or, we fail fast in case our solutions do not work.
Setting up sustainable systems. AI is and will be to enterprises what software was in the previous generation. Every business will have a variety of data platforms and AI/ML projects running simultaneously. Achieving success with AI will need a strong foundation in architecture principles and delivery practices.
For instance, at Thoughtworks, we use a software engineering approach called Continuous Delivery for Machine Learning (CD4ML) in which a cross-functional team produces ML applications based on code, data, and models in small and safe increments that can be reproduced, retrained, and reliably released at any time, in short, adaptation cycles. CD4ML helps maintain productivity, collaborate effectively, and deliver value in practice. Another emerging practice is MLOps or DataOps (similar to DevOps), which uses time-tested principles to operationalize AI.
This is still just a start, though. AI is evolving rapidly and unlocking various opportunities for businesses. But the risks of bias and exclusion are high too. Leveraging AI successfully requires a holistic approach encompassing tech, business value, processes, and social responsibility at large. This needs you to double down on existing best practices while being open to entirely new possibilities.
Balvinder Khurana, Technology Principal & Global Data Community Lead, Thoughtworks
Join our WhatsApp Channel to get the latest news, exclusives and videos on WhatsApp
_____________
Disclaimer: Analytics Insight does not provide financial advice or guidance. Also note that the cryptocurrencies mentioned/listed on the website could potentially be scams, i.e. designed to induce you to invest financial resources that may be lost forever and not be recoverable once investments are made. You are responsible for conducting your own research (DYOR) before making any investments. Read more here.