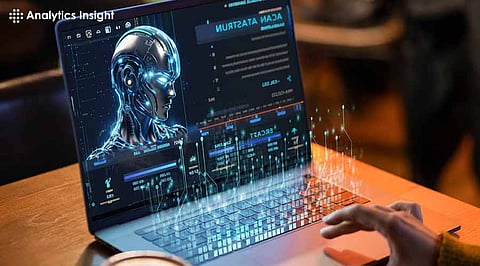
As new technologies are being developed in the foreseeable future, a new technique in processing data is categorized as Automated Machine Learning or AutoML. Thus, this approach is making machine learning easily accessible to all those who wish to apply data science in solving various issues. Analyzing the realm of AutoML, we discover the prospects of industries’ transformation, the improvement of decision-making, and the advancement of professionals and organizations as a whole.
AutoML history is conceived from the requirement to reduce automation complexity and recurrent and time-consuming processes of Machine Learning. Progenitors of machine learning models in the pre-AI era hence needed to master algorithm types, data preprocessing, feature selection, and proper hyperparameters to use. Nevertheless, modern AutoML services have already elaborated the ways how to choose the proper algorithms and their parameters automatically and make AI available for a wider spectrum of users.
Another benefit of AutoML is time-saving and of course, it is concerned with resource-saving as well. AutoML is beneficial in optimizing the whole process of model selection and tuning, which can free the data scientist and analyst’s time to work on more important aspects of the project. It lowers the threshold for participation by ‘normal’ users who can still help in the progress toward more data-driven decisions while denying their lack of deep understanding of machine learning.
It turns out AutoML is not a tool uniquely to tech companies; It has started creating ripples in different industries. Currently, it is applied in healthcare to anticipate the likelihood of the patient’s status and the tailor-made approaches. In finance, it assists in fraud protection and the evaluation of the level of risk. AutoML is applied in the retail industry for demand forecasting and classification of customers. Here are some samples of how AutoML is being applied to try and make use of data across various fields.
Nonetheless, AutoML comes with implications on its use as its main disadvantage: Some AutoML solutions involve a black box type, where the reasoning of the tool is not made clear. This can give rise to some problems of credibility and responsibility especially in sensitive applications. Furthermore, although AutoML is capable of eradicating many of the barriers to principle deployment, it is not designed to totally eliminate heuristics. Subsequently, data scientists are required to analyze the data, regulate the utilization, and produce context-specific meanings.
Moving on to the future of AutoML the following can be said. With the passage of time and the development of this technology, one could anticipate more sophisticated, as well as, more transparent AutoML systems. The combination of AutoML with other new technologies, and explainable Artificial Intelligence (XAI) will probably solve the problem of transparency. Moreover, future advancements in AutoML are expected to boost the capacities of this tool as a key means of data processing.
Automated Machine Learning is a major advancement in the field of data science. It’s revolutionizing data analytics through optimizing, democratising, and increasing the application of machine learning in different sectors. Nevertheless, further improvements of AutoML are still in progress, and thus, AutoML is a substantiated part of the modern data analysis ecosystem.
AutoML is a vivid example of the tremendous advancements in ML and AI taking place at the moment. It is not a trend it is a revolution that defines the way people analyze data and draw meaningful insights from them. As we continue to witness the growth of AutoML, one thing is clear: the turn of data analysis has come and has been taken over by automation systems.