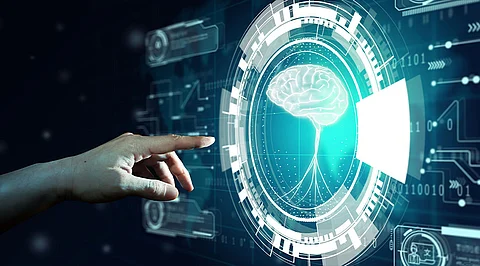
The business field is propelled by language. We believe it is present in everything from emails and documents to company records and beyond. Organizations, however, fight to increase the value of linguistic data, no matter how important it is to the project. Not only is there a tremendous amount of language data available to and maintained within organisations, but there is also a rapidly growing volume of it.
Organizations must devise a plan for exploiting the vast amounts of unstructured data at their disposal. So, while this push toward NLP is a good start, it is only that… a start. Natural language understanding (NLU) is where the true contrast for the business is made.
NLU is a specialization of NLP and is an aspect of artificial intelligence. Whereas NLP divides language into machine-understandable chunks, NLU goes above and beyond to help machines comprehend, decode, and reproduce that language. It offers unstructured data (such as contracts, emails, texts, social media, and other business reports) a design, allowing organisations to scale the reading, compiling, and analysing of message data for easier inquiry.
NLU bridges the gap between human communication and computer comprehension. We can use it to decipher the meaning of words in context through categorization, and to extract vital data from text content.
Building NLU capacity can be approached in a variety of ways. Each of them has its own set of advantages and disadvantages. The following are some of the more well-known approaches:
A symbolic methodology is based on language ideas that have already been established. An information diagram depicts information in a clear and concise manner, with rich, expressive, and meaningful renderings of ideas, both specific and general to a topic. This information backs up the clever clarifications of thinking outcomes.
This technique is human-driven, as it relies on phonetic rules and the information stored in the information diagram to understand language and its components by looking at etymological and semantic links. You can use this interaction to analyse language, extract information, and sort content.
Subject matter experts (SMEs) or possibly information engineers (KEs) are essential in this interaction because it frequently necessitates an undeniable level of control and the ability to adjust rules depending on the scenario.
The machine learning (ML) task of learning a capability that links a contribution to a yield is known as supervised learning (SL). The capacity is obtained from marked preparation data, which consists of a collection of preparing models. An information object and optimum yield esteem are included in every model. A directed learning calculation deconstructs the preparation data and generates a gathered capacity that may be used to plan new models.
In an ideal circumstance, the calculation is used to successfully choose class names for unobtrusive events. This necessitates the calculation to "reasonably apply" predictions from the preparation data to new and unobtrusive circumstances. The alleged speculation error is used to estimate the algorithm's factual quality.
For a long time, AI and symbolism have been regarded as the most effective approaches to dealing with natural language comprehension. They've been pitted against each other as completely unrelated options. As a result, associations have been forced to reconsider their positions in one way or another. Associations can use both ML and symbolic pairs in a mixed way, allowing them to recognise the key benefits of each. One important point to note is that a hybrid method does not imply that ML and representative function in conjunction.
Join our WhatsApp Channel to get the latest news, exclusives and videos on WhatsApp
_____________
Disclaimer: Analytics Insight does not provide financial advice or guidance. Also note that the cryptocurrencies mentioned/listed on the website could potentially be scams, i.e. designed to induce you to invest financial resources that may be lost forever and not be recoverable once investments are made. You are responsible for conducting your own research (DYOR) before making any investments. Read more here.