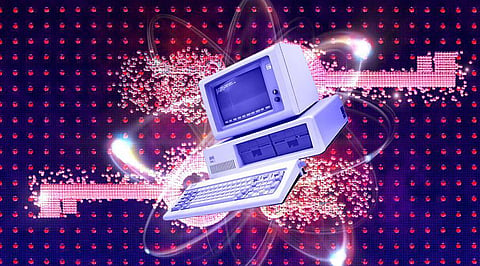
A new Caltech-led study in the journal Science presents how machine learning tools, run on traditional computers, are being used to make predictions about quantum systems and through this, it helps researchers resolve some of the trickiest physics and chemistry problems. While this concept has been exhibited experimentally before, this new report is the first to mathematically prove that the procedure works.
Before we get into the topic deeper, it's better to tell you that quantum computers are not magical. They sometimes appear to be magical, just because the fundamental math and physics behind them are a bit complicated. But the fact is, a quantum computer is slightly better than classical computers, nothing more. "Quantum computers are best for different types of physics and materials science problems," says lead author Hsin-Yuan (Robert) Huang, a graduate student working with John Preskill, the Richard P. Feynman Professor of Theoretical Physics, and the Allen V. C. Davis and Lenabelle Davis Leadership Chair of the Institute for Quantum Science and Technology (IQIM). "But we are not limited there yet and have been surprised to learn that classical machine learning methods can be used at the same time. Ultimately, this paper is about showing what humans can learn about the physical world."
Traditional computers, which are also known as classical computers are better at some particular tasks than quantum computers, like sending and receiving emails, creating spreadsheets and other documents, and desktop publishing. Quantum computers are not designed to replace classical computers but they are just meant to solve distinct problems than those solvable by traditional computers. It means any problem that is impossible to solve for traditional computers will also be impossible to resolve using quantum computers. But then the question arises if they only solve problems solvable on traditional computers, then what's all the hype about? Well, quantum computers are capable to solve some problems better, faster, and more efficiently than traditional computers.
At microscopic levels, the physical world appears to be an incredibly complex place dominated by the laws of quantum physics. In this realm, particles can live in a superposition of states, or two states at once. And a superposition of states can lead to entanglement, a circumstance in which particles are linked, or correlated, without even being in contact with each other. These unusual states and connections, which are extensively spread within natural and human-made materials, are challenging to represent mathematically. "Predicting the low-energy state of a material is difficult," says Huang. He further said "There are uncountable numbers of atoms, and they are superimposed and entangled. It's difficult to write down an equation to describe it all."
The new study is the first introductory mathematical demonstration that traditional machine learning can be used to fill the gap between us and the quantum world. Machine learning is a variety of computer application that mimics the human brain to learn from data. "We are traditional beings living in a quantum world," says Preskill. "Our brains and our computers are traditional, and as result, it limits our capability to interact with and acknowledge the quantum reality."
While previous studies have shown that machine learning applications can solve some quantum problems, these methods typically operate in ways that make it hard for researchers to observe how the machines entered their solutions. "Normally, when we talk about machine learning, we are not aware of how the machine solved the problem. It's a black box," says Huang. "But now we've essentially cracked out what's happening in the box through our numerical simulations." Huang and his colleagues did extensive numerical simulations in collaboration with the AWS Center for Quantum Computing at Caltech, which corroborated their theoretical results.
The new study will help scientists better understand and classify complex and exotic phases of quantum matter. "The worry was that people creating new quantum states in the lab might not be capable to grasp them," Preskill explains. "But now we can get reasonable classical data to explain what's happening. The classical machines don't just give us an answer like an oracle but provide us a deeper understanding."
Ultimately, future quantum-based machine learning tools will beat classical methods, the scientists say. In a related study appearing June 10, 2022, in Science, Huang, Preskill, and their collaborators report using Google's Sycamore processor, a rudimentary quantum computer, to illustrate that quantum machine learning is superior to classical approaches. "We are still at the very beginning of this field," says Huang. "But we do know that quantum machine learning will eventually be the most efficient.
Join our WhatsApp Channel to get the latest news, exclusives and videos on WhatsApp
_____________
Disclaimer: Analytics Insight does not provide financial advice or guidance. Also note that the cryptocurrencies mentioned/listed on the website could potentially be scams, i.e. designed to induce you to invest financial resources that may be lost forever and not be recoverable once investments are made. You are responsible for conducting your own research (DYOR) before making any investments. Read more here.