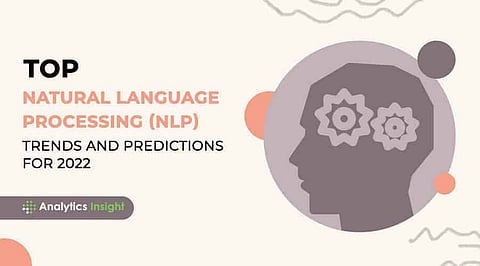
Natural language processing (NLP) is one of the hottest fields in artificial intelligence (AI) and machine learning (ML) right now. The global NLP industry is expected to reach US$42.04 billion by 2026, with a CAGR of 21.5%, according to Mordor Intelligence. This rapid expansion of NLP has resulted in the emergence of new trends and advancements in the field. Let's take a look at some NLP trends to look out for in 2022.
Transfer learning is a machine learning approach that involves training a model for one job and then repurposing it for a related activity. Instead of developing and training a model from start, which is costly, time-consuming, and requires a large quantity of data, you may simply fine-tune one that has already been trained. As a result, organizations can accomplish NLP jobs faster and with less labeled data. Transfer learning, which first gained popularity in the field of computer vision, is now being utilized in NLP applications such as intent classification, sentiment analysis, and named entity recognition.
NLP has become a critical tool for detecting and preventing the spread of fake news and disinformation, saving time and effort. We've already seen several intriguing methods to automatic fake news identification this year, and we'll likely see more of it in 2022, with so much incorrect information about Covid-19 circulating. Cyberbullying detection is another method NLP is being utilized to make a good influence. On social media, classifiers are being developed to detect the usage of abusive and derogatory language, as well as hate speech.
In 2022, sentiment analysis, also known as opinion mining, will continue to play a significant role, allowing businesses to monitor social media and gain real-time insights into how customers feel about their brand or products. Using natural language processing (NLP) tools to assess brand sentiment can assist businesses in identifying areas for improvement, detecting negative comments on the fly (and responding proactively), and gaining a competitive advantage. Analysis of the impact of marketing efforts and evaluating how consumers react to events such as a new product introduction are two more intriguing use cases for sentiment analysis in social media monitoring.
Reinforcement learning is a machine learning subfield that is expected to expand in 2021. Reinforcement algorithms, in essence, learn by doing, using a trial-and-error process that incorporates input from past acts and experiences. Reinforcement learning may be used in natural language processing to speed up tasks such as question answering, machine translation, and summarization. Currently, supervised algorithms are used to train NLP models, which are subsequently fine-tuned via reinforcement learning.
Too far, the majority of NLP advancements have been concentrated on English. Companies like Google and Facebook, on the other hand, are increasingly releasing pre-trained multilingual models that perform as well as or better than monolingual models. Open-source libraries are following in the footsteps of Google and Facebook, with recent improvements in language-agnostic sentence embeddings, zero-shot learning, and the availability of multilingual embeddings, thus we may anticipate seeing an increasing trend in multilingual NLP models in the coming year, 2022.
Combining supervised and unsupervised approaches for developing a model for NLP appears to produce more accurate results. Supervised learning, which is often used for tasks like topic categorization, necessitates a significant quantity of labeled data as well as several iterations before a model can generate good predictions. There is no labeled data in unsupervised learning: the model learns from input data and can recognize patterns and draw conclusions on unseen data on its own. Clustering is an example of this, in which related items are clustered together. Combining supervised and unsupervised learning has been proven to improve the performance of machine learning models, particularly for text interpretation.
Integrating natural language processing technologies with help desk software, for example, might automate time-consuming and laborious operations like labeling and routing customer support issues, freeing employees to focus on higher-value work. On the front lines of customer service, chatbots will continue to play an important role. Despite certain restrictions, they can typically have a basic conversation and do activities for which they have been trained. With advances in natural language processing (NLP) and rising customer service demand, we can expect to see significant progress toward the next generation of chatbots, which will be able to self-improve, hold more complex conversations, and possibly learn how to complete new tasks without prior training.
Back in the day, developing NLP models needed an extensive understanding of AI and machine learning, coding, open-source libraries, and other topics. No longer, thanks to low-code technologies that make life easier. While low-code or no-code tools have existed for some time, their use has been restricted to web and software development. We may see the emergence of these technologies in the NLP area as well in 2022. MonkeyLearn, a SaaS company, aims to democratize machine learning and natural language processing by making them accessible to non-technical people. The company has created a model builder that allows you to create, train, and integrate emotional analysis and text classification models using a point-and-click interface.
From a commercial standpoint, organizations that understand how AI will operate within a product, in addition to the technical skills required to launch and grow an NLP project, will do better. To remain competitive, all departments of an organization must comprehend the benefits of AI integration and how it will impact their roles. Many initiatives fail because of a lack of holistic AI integration, in which product managers, designers, marketing, salespeople, and others aren't actively involved in its adoption. In the coming year, 2022, the overall investment in knowledge, time, energy, and practice throughout the whole organization will determine its success.
BERT (Bidirectional Encoder Representations from Transformers) and ELMo (Embeddings from Language Models) will be the focus of the NLP community in 2022. These models have been trained on massive quantities of data and can enhance the performance of a wide range of NLP issues dramatically.
Join our WhatsApp Channel to get the latest news, exclusives and videos on WhatsApp
_____________
Disclaimer: Analytics Insight does not provide financial advice or guidance. Also note that the cryptocurrencies mentioned/listed on the website could potentially be scams, i.e. designed to induce you to invest financial resources that may be lost forever and not be recoverable once investments are made. You are responsible for conducting your own research (DYOR) before making any investments. Read more here.