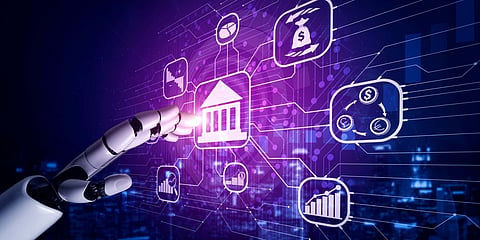
Machine Learning (ML) is reshaping the financial services like never before. It has become more prominent recently due to the availability of a vast range of data and more affordable computing power. It helps financial companies and banks to stand out of the box and achieve desired business growth.
In the modern era, financial institutions are running a race towards digitisation. Staying ahead of technological advancements is a mandatory resort for them. To keep up the pace, disruptive technologies like Artificial Intelligence (AI) and machine learning are improving the way finance sector functions. Leading banks and financial service companies are deploying AI technologies, including machine learning to streamline processes, optimize portfolios, decrease risk and underwrite loans amongst other things.
Machine learning in finance is all about digesting large amounts of data and learning from the data to carry out specific tasks like detecting fraudulent documents and predicting investments, and outcomes. Machine learning uses a variety of techniques to handle a large amount of data the system processes. Various financial houses like banks, fintech, regulators and insurance forms are adopting machine learning to better their services.
Machine learning uses statistical models to draw insights and make predictions. Some of the major use cases of machine learning in the financial sector are underwriting processes, portfolio composition and optimization, model validation, Robo-advising, market impact analysis, offering alternative credit reporting methods.
Machine learning is well known for its predictions and delivery of accurate results. The financial sector involves issues of data-rich problems which could be solved by the implementation of machine learning. Similar financial issues in banking and financial series can find a solution using machine learning algorithms.
Machine learning algorithms are designed to learn from data, processes, and techniques to find different insights. Here are some of the reasons why the financial sector should adopt machine learning,
• Improves productivity and user experience
• Enhances revenue
• Low operational cost due to process automation
• Gives security to transactions
Enhancing Financial Monitoring
Cyber risks in the financial sector are high. Unlike any other industry, finance involves a lot of money which could drive to a big loss or great fall if mishandled. Thus, financial monitoring is a provided solution for the issue through machine learning. Machine learning algorithms can be used to enhance network security significantly. Data scientists are also working on training systems to detect flags such as money laundering techniques, which can be prevented by financial monitoring.
Making Investment Predictions
Machine learning stands out for its feature to predict the future using the data from the past. The system analyzes a large set of data and comes up with answers to various future related questions. This gives machine learning the ability to have market insights that allows the fund managers to identify specific market changes. Henceforth, divergence in the market can be detected much earlier as compared to the traditional investment models.
Well known financial institutions like JPMorgan, Bank of America and Morgan Stanley are heavily investing in machine learning technologies to develop automated investment advisors.
Maximizing Process Automation
One of the major changes that AI is driving in the financial sector is replacing human labor. Banking sectors are the primary adopters of AI applications like chatbots, virtual assistant and paperwork automation. Financial service companies followed the suit. Machine learning allows finance companies to completely replace manual work by automating repetitive tasks through intelligent process automation. This enables better customer experience and reduces cost.
Furthermore, machine learning accesses data, interprets behaviour, and recognizes patterns which will better the functions of the customer support system. Wells Fargo uses ML-driven chatbots through Facebook Messenger to communicate with the company's users effectively.
Ensuring Safe Transaction
Machine learning is an expert in flagging transactional frauds. The mechanism analyzes millions of data points that go unnoticed by human vision. Ultimately, machine learning also reduces the number of false rejections and helps improve the precision of real-time approvals. These system models are built using previous client interaction and transaction history.
According to a report, it is predicted that for every US$1 lost to fraud, the recovery costs are US$2.92. Henceforth, detecting suspicious behavior and preventing real-time fraud is a mandatory move for the finance sector. Machine learning powered technologies are equipped to deal with the crisis.
Credit card fraud detection is the highest beneficiary of ML prediction making. The system is trained to monitor historical payments data which alarms bankers if it finds anything fishy.
Managing Risky Situations
The financial sector involves a lot of cash transactions between customers and the institutions. It increases the risk of being mishandled. However, machine learning techniques leverage security to the institutions by analyzing the massive volume of data sources. The system can go through significant volumes of personal information to reduce the risk.
For example, lending loan to an individual or an organization goes through a machine learning process where their previous data are analyzed. This could prevent from lending to fraudulent borrowers.
Enabling Algorithmic Trading (AT)
Algorithmic Trading (AT) has become a dominant force in global financial markets. Machine learning unravels the feature that allows trading companies to make decisions based on close monitoring of funds and news. It detects patterns that can enable stock price to go up or down. Some of the other benefits of Algorithm Trading are,
• Allows trades to be executed at a maximum price
• Human errors are substantially reduced
• Increases accuracy and reduces the chances of mistake
Insisting on Better Decision-making
Decision making by customers on both large and small investments is important for the finance institutions. Henceforth, financial sector organizations are suggesting customers with sources where they can get more revenue. This is possible with machine learning performing analysis on structured and unstructured data.
Amplifying Marketing Strategy
Machine learning helps financial institutions analyze the mobile app usage, web activity and responses to previous ad campaigns. This provides an insight into what could be the strategy of marketing. Machine learning and AI acts as a marketing tool under such circumstances.
Aiding Customer Retention Program
Credit card companies use machine learning technology to diagnose high-risk customers. The application includes a predictive, binary classification model to find out the customers at risk. Machine learning predicts user behavior and designs offers based on their demographic data and transaction activity.
Managing Customer Data on a Large-scale
Customer data is an asset that is valued at hundreds of millions of dollars at financial institutions. Data is the most crucial resource which makes efficient data management central to the growth and success of the business. The manual processing of data from mobile communication, social media activity, and market data is near impossible.
But AI and machine learning tools like data analytics, data mining, and NLP helps get valuable insights from data for better business profitability. For example, machine learning algorithms are being used for analyzing the influence of market developments and specific financial trends from the financial data of the customers.
Join our WhatsApp Channel to get the latest news, exclusives and videos on WhatsApp
_____________
Disclaimer: Analytics Insight does not provide financial advice or guidance. Also note that the cryptocurrencies mentioned/listed on the website could potentially be scams, i.e. designed to induce you to invest financial resources that may be lost forever and not be recoverable once investments are made. You are responsible for conducting your own research (DYOR) before making any investments. Read more here.