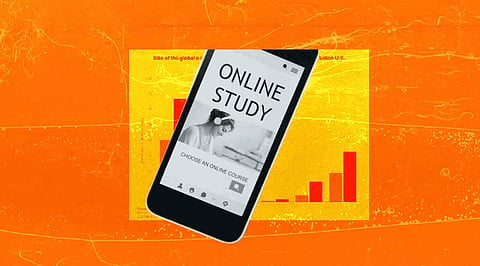
Self Supervised Learning Market Prediction: In the realm of artificial intelligence (AI) and machine learning (ML), self-supervised learning has emerged as a powerful paradigm shift, promising significant advancements in data analysis, processing efficiency, and cost-effectiveness. The Self-supervised Learning market size was valued at US$12.40 million in revenue in 2023 and is anticipated to reach US$50.61 million by 2028, with a CAGR of 32.49% over the forecast period.
The foundation of the self-supervised learning market's growth lies in the escalating adoption of AI and ML techniques across various sectors. Organizations are increasingly recognizing the potential of AI-driven solutions to enhance decision-making, automate processes, and uncover valuable insights from data. Self-supervised learning, with its ability to learn representations from unlabeled data, aligns perfectly with this trend, offering a scalable approach to leveraging vast amounts of unannotated data for training robust ML models.
In today's data-driven world, the demand for efficient and cost-effective data analysis solutions is paramount. Traditional supervised learning methods often rely on manually labeled datasets, which can be time-consuming, labor-intensive, and expensive to obtain. Self-supervised learning presents a compelling alternative by leveraging unlabeled data, thereby eliminating the need for extensive manual annotation. This not only accelerates the training process but also reduces the associated costs, making it an attractive proposition for organizations seeking scalable data analysis solutions.
The rapid advancements in deep learning algorithms serve as a catalyst for the expansion of the self-supervised learning market. Deep neural networks have demonstrated remarkable capabilities in learning complex representations from raw data, fueling breakthroughs in various domains such as computer vision, natural language processing, and speech recognition. Self-supervised learning techniques, combined with sophisticated deep learning architectures, enable more nuanced and nuanced understanding of data, paving the way for enhanced predictive accuracy and performance across diverse applications.
The application of self-supervised learning is not limited to a specific industry but spans across various sectors, including healthcare, finance, retail, and more. In healthcare, for instance, self-supervised learning models can analyze medical imaging data to assist in disease diagnosis and treatment planning. In finance, these models can uncover patterns in financial transactions for fraud detection and risk assessment. Similarly, in retail, self-supervised learning can analyze customer behavior and preferences to personalize marketing campaigns and improve customer engagement. The versatility of self-supervised learning algorithms makes them adaptable to a wide range of use cases, driving their widespread adoption and market growth.
The banking industry is experiencing a profound transformation driven by automation, predictive analytics, and the proliferation of internet-connected devices. Self-supervised learning holds immense potential in this domain, enabling banks and financial institutions to automate routine processes, enhance customer experiences, and mitigate risks more effectively. By analyzing vast amounts of transactional data, self-supervised learning models can identify patterns, anomalies, and trends, empowering financial institutions to make data-driven decisions in real-time.
The future of self-supervised learning market is poised for exponential growth, propelled by the increasing adoption of AI and ML techniques, the need for scalable data analysis solutions, advancements in deep learning algorithms, growing applications across industries, and automation in banking processes and predictive analytics. By harnessing the power of self-supervised learning, organizations can unlock new opportunities for innovation, efficiency, and competitiveness in an ever-evolving digital landscape.
Join our WhatsApp Channel to get the latest news, exclusives and videos on WhatsApp
_____________
Disclaimer: Analytics Insight does not provide financial advice or guidance. Also note that the cryptocurrencies mentioned/listed on the website could potentially be scams, i.e. designed to induce you to invest financial resources that may be lost forever and not be recoverable once investments are made. You are responsible for conducting your own research (DYOR) before making any investments. Read more here.