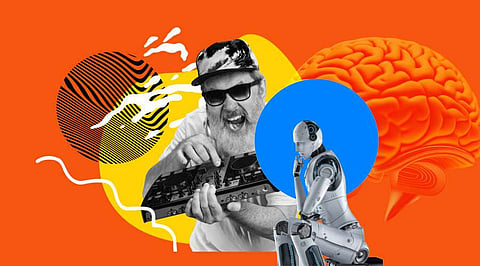
Machine learning (ML) is a branch of artificial intelligence (AI) that enables computers to learn from data and make predictions or decisions without being explicitly programmed. ML has many applications in various domains, such as computer vision, natural language processing, recommender systems, and self-driving cars. However, ML faces challenges and limitations, such as data quality, model robustness, interpretability, and ethical issues.
One of the challenges ML researchers are trying to address is accounting for uncertainty and human error in AI applications where humans and machines collaborate. Uncertainty is fundamental to human reasoning and decision-making, but many ML models must capture or handle it properly. For example, when a human provides feedback or labels to an ML model, the model often assumes that the human is always certain and correct, which is unrealistic. Humans can make mistakes, have doubts, or change their minds. Moreover, humans can have different levels of confidence or uncertainty depending on the context, task, knowledge, and experience.
To bridge the gap between human behavior and machine learning, researchers from the University of Cambridge, The Alan Turing Institute, Princeton University, and Google DeepMind have been developing a way to incorporate human error and uncertainty into ML systems. They adopted a well-known image classification dataset called CIFAR-10, which consists of 60,000 images of 10 different classes of objects, such as airplanes, cats, dogs, and trucks. They asked human annotators to label some of the images and indicate their level of uncertainty using a scale from 1 (very uncertain) to 5 (very certain). They also introduced some noise and label errors to simulate human mistakes.
The researchers then trained several ML models using the human-annotated data and evaluated their performance on a test set of images. They compared the models that used uncertain labels with those that used only certain labels or ignored the uncertainty information. They found that training with uncertain labels can improve the models' performance in handling uncertain feedback, although humans also cause the overall performance of these hybrid systems to drop. The researchers also analyzed how different types of uncertainty affect the models' learning process and outcomes.
The researchers hope their work can help improve the trust and reliability of AI applications where humans and machines work together, especially in safety-critical settings, such as medical diagnosis. They argue that accounting for human error and uncertainty is essential for designing more robust and ethical ML systems that align better with human values and preferences.
Join our WhatsApp Channel to get the latest news, exclusives and videos on WhatsApp
_____________
Disclaimer: Analytics Insight does not provide financial advice or guidance. Also note that the cryptocurrencies mentioned/listed on the website could potentially be scams, i.e. designed to induce you to invest financial resources that may be lost forever and not be recoverable once investments are made. You are responsible for conducting your own research (DYOR) before making any investments. Read more here.