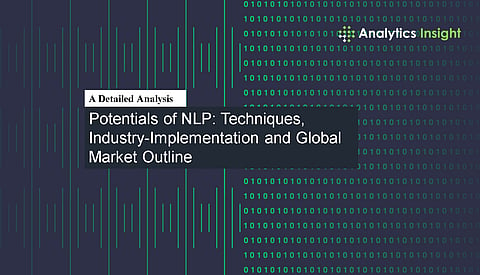
The area of research and application that is used to explore the ways in which computers can be employed to understand and manipulate natural language text and speech, is what we commonly call 'Natural Language Processing' or NLP. The researchers and experts who belong to this area aim to gather knowledge on how human beings understand and use language so that appropriate tools and techniques can be developed to make computer systems understand and manipulate natural languages to perform the desired tasks. Moreover, the number of disciplines on which the foundation of NLP lies are – computer and information sciences, linguistics, mathematics, electrical and electronic engineering, artificial intelligence and robotics, psychology – to name a few. Its tools and applications are numerous which include a number of fields of studies, such as machine translation, natural language text processing and summarization, user interfaces, multilingual and cross-language information retrieval (CLIR), speech recognition, artificial intelligence and expert systems, and so on.
As noted by a report, many researchers worked on this technology, building tools and systems which makes NLP what it is today. Tools like Sentiment Analyser, Parts of Speech (POS)Taggers, Chunking, Named Entity Recognitions (NER), Emotion detection, Semantic Role Labelling made NLP a good topic for research.
Sentiment Analyzer works by extracting sentiments about given topic. The analysis consists of a topic specific feature term extraction, sentiment extraction, and association by relationship analysis. Sentiment Analysis utilizes two linguistic resources for the analysis: the sentiment lexicon and the sentiment pattern database. It analyses the documents for positive and negative words and try to give ratings on scale -5 to +5.
For parts of speech taggers for languages like European languages, research is being done on developing it for other languages like Arabic, Sanskrit, Hindi etc. It can efficiently tag and classify words as nouns, adjectives, verbs etc. The most procedures for part of speech can work efficiently on European languages, but it won't on Asian languages or middle eastern languages. Sanskrit part of speech tagger specifically uses treebank technique. Arabic uses Support Vector Machine (SVM) approach to automatically tokenize, parts of speech tag and annotate base phrases in Arabic text.
Moreover, Chunking which is also known as Shadow Parsing, works by labelling segments of sentences with syntactic correlated keywords like Noun Phrase and Verb Phrase (NP or VP). Every word has a unique tag often marked as Begin Chunk (B-NP) tag or Inside Chunk (I-NP) tag. Chunking is often evaluated using the CoNLL 2000 shared task. CoNLL 2000 provides test data for Chunking. Since then, a certain number of systems arised, all reporting around 94.3% F1 score. These systems use features composed of words, POS tags, and tags.
The Named Entity Recognition in used at many places such as the Internet where the problem is people don't use traditional or standard English. This degrades the performance of standard natural language processing tools substantially. By annotating the phrases or tweets and building tools trained on unlabelled, in domain and out domain data. It improves the performance as compared to standard natural language processing tools.
Additionally, Emotion Detection is similar to sentiment analysis, but it works on social media platforms on mixing of two languages (English + Any other Indian Language). It categorizes statements into six groups based on emotions. During this process, they were able to identify the language of ambiguous words which were common in Hindi and English and tag lexical category or parts of speech in mixed script by identifying the base language of the speaker.
Besides, Sematic Role Labelling – SRL works by giving a semantic role to a sentence. For example, in the PropBank formalism, one assigns roles to words that are arguments of a verb in the sentence. The precise arguments depend on verb frame and if there exist multiple verbs in a sentence, it might have multiple tags. State-of-the-art SRL systems comprise of several stages: creating a parse tree, identifying which parse tree nodes represent the arguments of a given verb, and finally classifying these nodes to compute the corresponding SRL tags.
Also, Event discovery in social media feeds, using a graphical model to analyse any social media feeds to determine whether it contains name of a person or name of a venue, place, time etc. The model operates on noisy feeds of data to extract records of events by aggregating multiple information across multiple messages, despite the noise of irrelevant noisy messages and very irregular message language, this model was able to extract records with high accuracy. However, there is some scope for improvement using broader array of features on factors.
Further, the coolest aspect regarding text analysis is, it's all over! Regardless of industry, organizations and people want to settle on better-informed business decisions based off identifiable and quantifiable knowledge. With progressions in Text Analysis, organizations would now be able to mine text to insights and improve their service or offering to flourish in their market.
Applications
Identifying the potential of NLP, we have enlisted some of the most common and widespread applications of the technology.
Neural Machine Translation
Neural machine translation has improved the imitation of professional translations over the years of its advancement. When applied in neural machine translation, natural language processing helps educate neural machine networks. This can be used by businesses to translate low impact content including emails, regulatory texts, etc. Such machine translation tools speed up communication with partners while enriching other business interactions.
Sentiment Analysis
Sentiment analysis helps in estimating customer feedback on the brand and product while adjusting sales and marketing strategy. It is also termed as opinion mining which is capable of analyzing news and blogs assigning a value to the text (negative, positive or neutral) over social media platforms. As it stands now NLP algorithms can identify emotions such as happy, annoyed, angry, sad. Through the combination of sentiment analysis and NLP, marketers will have all it takes to develop actionable strategies and make well-informed decisions.
HR and Recruiting
Using NLP in human resources, HR professionals can speed up candidate searches by filtering out relevant resumes and designing bias-proof and gender-neutral job descriptions. Also by making the use of semantic analysis, software sifts through the considerable synonyms enabling recruiters to detect candidates that meet the job requirements.
Advertising
Through the analysis of digital footprint over social media, emails, search keywords, and browsing behavior, NLP enables advertisers to identify new audiences potentially interested in their products. Even a simple keyword matching routine companies can broaden the range of channels for ad placement, helping companies spend their ad budgets more effectively and target potential clients using NLP algorithms.
Healthcare
According to Becker's Hospital Review, NLP can improve clinical documentation, data mining research, computer-assisted coding, automated registry reporting. In the context of emerging cases, it helps in clinical trial matching, clinical decision support, risk adjustment, and hierarchical condition categories.
Additionally, for next-generation advancements, NLP enables ambient virtual scribe, computational phenotyping and biomarker discovery and population surveillance.
NLP applications are increasing at a fast pace and the technology has all it takes to accelerate customer service. NLP based software now even impact our personal lives as well. According to market reports, people will have more interactions with chatbots than with their spouses by 2020.
Besides, in the professional ecosystem, as aforementioned, NLP use cases provide a better and basic understanding of this technology can do to maximize productivity, streamline operations, deliver insights and keep up with the competition.
Search Autocomplete
Search autocomplete is another kind of NLP that numerous individuals use consistently and have nearly generally expected when looking for something. This is thanks to enormous part to pioneers like Google, who have been utilizing the feature in their search engine for quite a long time. The element is similarly as supportive on company sites.
Salesforce coordinated the feature into their own search engine. Clients keen on getting familiar with a topic or function of Salesforce's product may know one keyword, however, perhaps not the full term. Search autocomplete will enable them to find the right data and answer their inquiries quicker. This enables to chop down on the probability that they'll end up uninvolved and explore far from the site.
Financial Trading
Similarly, as with sports trading, having a knowledge into what's going on at a local level can be entirely significant to a financial trader. Domain explicit sentiment analysis/classification can add genuine value here. A similar manner by which fans have their own unmistakable vocab based on the game, so too do traders in specific markets. Intent recognition and Spoken Language Understanding services for identifying user intents (for example "purchase", "sell", and so forth) from short articulations can help dealers in choosing what to trade, how much and how rapidly.
Creditworthiness Assessment
By utilizing NLP, banks in developing nations would now be able to evaluate the creditworthiness of customers with practically zero financial records. Regardless of whether these customers have never utilized credit, the majority of them despite everything use cell phones, browse on the web and take part in different exercises that leave a lot of digital impressions. NLP algorithms analyze geolocation information, social media activity, browsing behavior to infer bits of knowledge into their habits, peer systems, and quality of their relationships. By assessing a lot of customer related factors, the software creates a credit score exceptionally prescient of client's further activity. Access to client information is only allowed on assent and the information can never be transferred to outsiders.
One of the instances of creditworthiness evaluation tools dependent on NLP and text mining is the Lenddo application created by a Singapore-based organization LenddoEFL. Lenddo has built up its patented innovation based on 4 years of actual online lending background that included collection, analysis and processing of billions of information points. In 2015, Lenddo opened its API for outsiders, for example, banks, lending organizations, utilities and credit card organizations worldwide to diminish risk, increase portfolio estimate, improve customer service, and verify candidates.
TV Advertising & Audience Analysis
TV programs or live broadcast events are probably the most discussed topics on Twitter. Advertisers and TV makers can both profit by utilizing Text Analytics in two particular ways. If producers can get a comprehension of how their group of spectators 'feels' about specific characters, settings, storylines, highlighted music and so on, they can make changes in a bid to appease their viewers and consequently increase the crowd size and viewers ratings. Advertisers can delve into social media networking platform streams to analyze the viability of product placement and commercials broadcasted during the breaks.
For instance, the TV character 'Cersei' from Game of Thrones is turning into a style symbol among fans, who consistently Tweet about her most recent gown. High street retailers that need to exploit this pattern could come up with a line of Queen of Westeros' style attire and adjust their commercials to shows like Game of Thrones. Text Analytics could likewise be utilized by TV Executives hoping to offer to advertisers. For instance, a TV organization could mine viewers' tweets and discussion activity to profile their group of spectators all the more precisely. So rather than just pitching the size of their group of spectators to publicists, they could wow them by recognizing their gender, area, age and so forth and their emotions towards specific products.
Sentiment Analysis
With regards to modifying sales and marketing strategy, sentiment analysis helps gauge how clients feel about your brand. This innovation, otherwise called opinion mining, originates from social media analysis and is fit for analyzing news and blogs allocating a value to the content (negative, positive or neutral). A Switzerland-based organization Sentifi utilizes NLP to discover influencers and characterize its key brand advocates. The present NLP algorithms go as far as recognizing emotions, for example, glad, irritated, grumpy, miserable. Obviously, with exact tools like these marketers currently, have everything necessary to create significant strategies and settle on informed decisions.
One of the main European retailers was hoping to harness the power of NLP and text mining for customer feedback sentiment analysis and employed a committed AI development team in Ukraine to come up with an answer. By including this sentiment analysis tool, the organization proposed to expand customer loyalty, drive business changes, and accomplish a considerable return on sales and marketing investments. Allocate, the organization that facilitated the retailer's devoted software team, performed data purifying and munging as the essential task and connected words tokenization alongside a Natural Language Toolkit that was utilized to characterize equivalent words, semantics, and the general tone of voice of feedback. Subsequently, the team executed the business rationale based on language characteristics, abbreviations, collocations, and vernacular articulations, and finished a comprehensive semantic analysis.
This arrangement helped the retailer streamline and overhaul their marketing and sales strategy, which brought about 30% income increment within a year of deployment.
According to Analytics Insight, NLP is not a new technology. Siri, Cortana and Google Assistant are the known voice-controlled natural language interfaces that use the technology. Major IT firms including Google, Microsoft, IBM and Apple have piqued interest in NLP and have been researching further in this area. The technology has the potential to change the way we communicate with machines using machine learning, big data, and artificial intelligence.
According to an industry report, the global Natural Language Processing (NLP) market size to grow from US$ 10.2 billion in 2019 to US$ 26.4 billion by 2024, at a CAGR of 21.0 percent during the forecast period 2019-2024. The report further depicts that the major growth factors of the NLP market include the increase in smart device usage, growth in the adoption of cloud-based solutions and NLP-based applications to improve customer service, as well as the increase in technological investments in the healthcare industry.
Road Ahead
The development of NLP applications is challenging since text and voice contain information at many granularities from hierarchical syntactic representation to high-level logical representation. The biggest challenge now is to build effective software that will understand the information which is often ambiguous, has emotions, tone, and often lack a structure. A lot of companies are delving deep into this area to address the problems using a blend of knowledge-engineered, and statistical and machine-learning techniques to disambiguate and respond to natural human language.
These developments will ultimately help organisations to cross out the use of specialized programming languages and use pure human input for information processing. Needless to say, it will open doors to more detailed insights and effective predictive models. With the high-volume of information available today, the NLP applications will effectively process information for enhanced insights and decision making. As Carly Fiorina, Former CEO of Hewlett-Packard Said," The goal is to turn data into information, and information into Insights".
Join our WhatsApp Channel to get the latest news, exclusives and videos on WhatsApp
_____________
Disclaimer: Analytics Insight does not provide financial advice or guidance. Also note that the cryptocurrencies mentioned/listed on the website could potentially be scams, i.e. designed to induce you to invest financial resources that may be lost forever and not be recoverable once investments are made. You are responsible for conducting your own research (DYOR) before making any investments. Read more here.