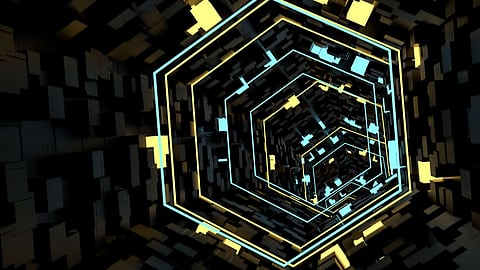
Neuromorphic computing is defined as the next-generation of AI which comprises the production and use of neural networks as analogue or digital copies on electronic circuits. It represents a new approach for non-Turing computation that intends to reproduce aspects of continuing dynamics and computational functionality found in biological brains. The concept, which was coined by American Scientist Carver Mead in the late 1980s, typically refers to a variety of brain-inspired computers, devices, and models.
There is no wonder that future computing systems will capitalize on an increased understanding of the human brain through the use of neuromorphic architectures and computational principles. Neuromorphic computing promises to provide a tool for neuroscience to comprehend the dynamic processes of learning and development in the brain and imply brain inspiration to generic cognitive computing. Unlike conventional capabilities, this biologically-inspired approach is energy efficient, with having faster execution, robustness against local failures and the ability to learn.
Since AI carries a large number of interpretations, subsets, and theories that define its capabilities, the major goal of this technology is to replicate human functionalities. Both AI and neuromorphic computing in many ways seek to imitate and even outperform human intelligence. However, both technological approaches are limited by the hardware capabilities on which these systems function.
Neuromorphic computers are able to perform complex calculations faster, more power-efficiently, and on a smaller footprint than traditional von Neumann architectures. These features deliver compelling reasons for developing hardware that employs neuromorphic architectures. The interest in neuromorphic computing is also driven by machine learning capabilities. The approach demonstrates promise in enhancing the overall learning performance for specific tasks. This moves away from hardware benefits to understanding potential application benefits of neuromorphic computing, to develop algorithms that can learn in real-time similar to biological brains. Neuromorphic architectures appear to be the most appropriate platform for deploying machine learning algorithms in the future.
Many neuromorphic systems leverage AER (address-event representation), including France's Prophesee and the Swiss firm aiCTX (AI cortex) which focused on sensory processing. AER is a communication protocol for conveying spikes between bio-inspired chips. That approach is equally elegant and practical, delivering the benefits of hard-wired connections between neurons without all the wiring. This means information from an incoming signal can simply flow through the processor in real-time, while discarding irrelevant information and the remainder will further be processed in the neural pipeline.
Though neuromorphic computing, also knowns as neuromorphic engineering, is relatively new and promises many advantages, there are many ethical considerations apply to neuromorphic systems as similar to human-like machines and AI. While these systems are aimed at reproducing a human brain, a unique set of ethical questions arises surrounding their usage. One of the most significant ethical challenges that may be placed on neuromorphic computing is public perception. In a survey by the European Commission, titled Special Eurobarometer 382: Public Attitudes Towards Robots, over 60 percent of EU citizens uncomfortable about the idea of using robots in the care of their children and elderly parents.
Moreover, as neuromorphic computing is still in its infancy, capabilities of this technology yet to realize. It also promises to be a powerful method of creating futuristic computing hardware and revolutionary AI software.
Join our WhatsApp Channel to get the latest news, exclusives and videos on WhatsApp
_____________
Disclaimer: Analytics Insight does not provide financial advice or guidance. Also note that the cryptocurrencies mentioned/listed on the website could potentially be scams, i.e. designed to induce you to invest financial resources that may be lost forever and not be recoverable once investments are made. You are responsible for conducting your own research (DYOR) before making any investments. Read more here.