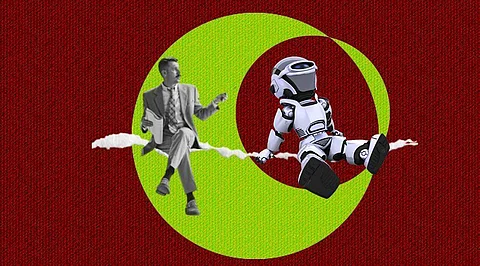
Robot companies are working on making robots work together in teams as well as programming them so they have the freedom to operate on the plant floor with human or robotic assistance. With swarms of these working together but separately, the system can determine which robot is in the best position to retrieve the next bin in order at any given time. Downtime is virtually eliminated because there's always another robot nearby if one gets stuck or needs to recharge its batteries. Battery charging is also autonomous.
The human role in sophisticated information-gathering systems is usually conceived to be that of the consumer. Human sensory and perceptual capabilities, however, outstrip our abilities to process information substantially. Using human operators as "perceptual sensors" is standard practice for both UAVs and ground robotics. Humans are called upon to "process" camera video to find targets and assist in navigation.
Whether in an industrial robot, or mobile robots for customer services, smart home, or medical healthcare, semiconductors from Infineon are the key enablers for all major robotic functions. They give machines human-like senses and real-time control. They connect them to the internet and energy-efficiently power their actions. And, they protect their identities, digital data, and hence the network from unauthorized access. The interaction of all these components – from hardware to software – is perfectly coordinated in order to be able to react as a system with the required intelligence.
A team of researchers from the University of Illinois at Urbana-Champaign and Stanford University led by Prof. Katie Driggs-Campbell have recently developed a new deep reinforcement learning-based method that could improve the ability of mobile robots to navigate crowded spaces safely. Their method, introduced in a paper pre-published on arXiv, is based on using people in the robot's surroundings as indicators of potential obstacles.
The idea of using people and their interactive behaviors to estimate the presence or absence of occluded obstacles was first introduced by Afolabi et al in 2018, specifically in the context of self-driving vehicles. In their previous work, Itkina and her colleagues built on this group's efforts, generalizing the "people as sensors" idea so that it considered multiple observed human drivers, instead of a single driver (as considered by Afolabi's team's approach).
To do this, they developed a "sensor" model for all the different drivers in an autonomous vehicle's surroundings. Each of these models mapped the driver's trajectory to an occupancy grid representation of the environment ahead of the driver. Subsequently, these occupancy estimates were incorporated into the autonomous robot's map, using sensor fusion techniques.
Most previously developed models viewing people as sensors are specifically designed to be implemented in urban environments, to increase the safety of autonomous vehicles. On the other hand, the new model was designed to improve a mobile robot's ability to navigate crowds of people.
Crowd navigation tasks are generally more complex than urban driving tasks for autonomous systems, as human behaviors in crowds are less structured and thus more unpredictable. The researchers decided to tackle these tasks using a deep reinforcement learning model integrated with an occlusion-aware latent space learned by a variational autoencoder (VAE).
The goal is to create intelligent factories and smart homes in which people, machines, systems, and products communicate with each other independently – but in a coordinated manner. They will enable smart value chains and product lifecycles, from development to manufacturing and assembly, product delivery and predictive maintenance, and recycling. The aim is to make manufacturing processes more flexible, scalable, and efficient to support the people who work with them and conserve scarce resources.
Join our WhatsApp Channel to get the latest news, exclusives and videos on WhatsApp
_____________
Disclaimer: Analytics Insight does not provide financial advice or guidance. Also note that the cryptocurrencies mentioned/listed on the website could potentially be scams, i.e. designed to induce you to invest financial resources that may be lost forever and not be recoverable once investments are made. You are responsible for conducting your own research (DYOR) before making any investments. Read more here.