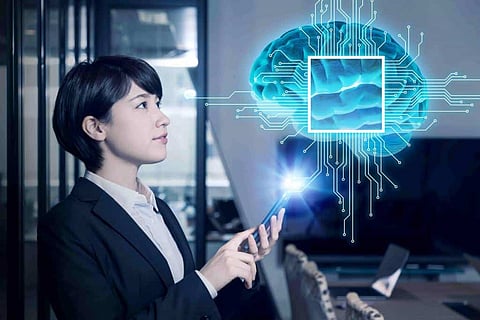
Mobile developers have a great deal to pick up from progressive changes that on-device ML can offer. This is a result of the innovation's capacity to reinforce mobile applications—in particular, taking into consideration smoother customer experiences equipped for utilizing incredible highlights, for example, giving exact area-based recommendations or promptly detecting plant illnesses.
This fast improvement of mobile machine learning has occurred as a response to various basic issues that traditional machine learning has toiled with. In truth, the composing is on the divider. Future mobile applications will require faster preparing paces and lower latency.
One may ask why AI-first mobile applications can't just run inference in the cloud. For one, cloud advancements depend on central nodes (envision a gigantic data center with huge amounts of storage space and computing power). What's more, such a unified methodology is unequipped for taking care of processing speeds important to make smooth, ML-fueled mobile experiences. Data must be processed on this centralized data center and after that sent down to the device. This requires some serious energy and money, and it's difficult to ensure information security. Let's review how machine learning is helping mobile applications to improve.
Voice services are telecoms' characteristic specialized field. A few organizations are banding together with the pioneers in speech and voice services, joining, for instance, Alexa environment. Others build up their own solutions or secure smaller new businesses. South Korean organizations are standing out. As of late, SK Telecom has presented its artificial intelligence-based voice assistant service for the home, which was a response to the move by its local rival – KT deployed its artificial intelligence collaborator to a hotel in South Korea with English language support.
Machine Learning is additionally helpful in decreasing churn rates, which can average every year from 10 to as much as 67%. Telecoms can prepare algorithms to anticipate when a customer is probably going to go to another organization, and what offer could keep them from doing it.
By automatically observing homes and sending constant alerts, subscribers can get extra value, and mobile services can give new income producing services. IoT joined with machine learning, can be utilized to watch, learn and automate a specific rehashed chain of occasions. For instance, after the front entryway is opened, the lights in the entrance and living room can be turned on, the warmth can be transformed up and the TV can be fixed on the client's preferred news show. Also, when there is irregular conduct, for example, a second-floor window opening and the front entryway is opened, a security alarm can be sent. The demand for the accommodation and security of smart home automation is relied upon to increase, as indicated by a report from Juniper Research that notes hardware and services deals for these homes are probably going to develop to $195 billion in the next two years.
Since information shouldn't be sent to a server or the cloud for processing, cybercriminals have fewer chances to misuse any vulnerabilities in this data transference, along these lines safeguarding the sanctity of the information. This enables mobile developers to meet GDPR guidelines on data security all the more effectively.
On-device ML solutions likewise offer decentralization, much similarly that blockchain does. As it were, it's harder for hackers to bring down a connected system of concealed devices through a DDoS assault when compared with a similar assault focusing on a centralised server. This innovation could likewise demonstrate to be valuable for drones and law authorization pushing ahead. The Apple cell phone chips are likewise improving client security and privacy. For example, they fill in as the foundation of Face ID. This iPhone feature depends on an on-device neural net that gathers data on all the various ways its user's face may look, filling in as a more precise and secure identification strategy. This and future classes of AI-empowered hardware will make ready for more secure cell phone experiences for clients, offering mobile engineers an extra layer of encryption to protect clients' information.
Mobile towers are the ideal product for ML predictive maintenance solutions. They are hard to access and require tedious on-site investigations of muddled modules, for example, power generators or air conditioners. Also, towers are powerless against interruptions, as they contain a ton of significant hardware.
There are different potential uses of ML in the maintenance of mobile towers, for example, empowered surveillance, where video and picture analysis can help recognize peculiarities. The media communications infrastructure is as of now outfitted with different sensors. The information those sensors gather can be utilized for preparing ML models, which will anticipate potential disappointments. This would diminish downtime and fix expenses, and furthermore improve the coverage.
Nokia utilizes ML algorithms are to change the best setup for 5G antennas and indoor positioning of articles or designing uplink and downlink channels. An active system configuration data can be cross-checked with asset management frameworks to amplify network use and improve coverage.
On-device MLI is likewise intended to spare you a fortune, as you won't need to pay external suppliers to actualize or keep up these solutions. As recently referenced, you won't require the cloud or the Internet for such solutions.
GPUs and AI-explicit chips are the costliest cloud services you can purchase. Running models on-device implies you don't have to pay for these clusters, because of the inexorably complex Neural Processing Units (NPUs) cell phones have nowadays.
Keeping away from the overwhelming, data-processing bad dream among mobile and the cloud is an enormous cost-saver for organizations that pick on-device ML solutions. Having this on-device derivation likewise brings down data bandwidth demands, at last sparing a heavy sum in expenses.
Mobile developers likewise spare enormously on the advancement procedure, as they won't need to construct and maintain extra cloud framework. Rather, they can accomplish more with a smaller developer group, accordingly enabling them to scale their development groups all the more proficiently.
Join our WhatsApp Channel to get the latest news, exclusives and videos on WhatsApp
_____________
Disclaimer: Analytics Insight does not provide financial advice or guidance. Also note that the cryptocurrencies mentioned/listed on the website could potentially be scams, i.e. designed to induce you to invest financial resources that may be lost forever and not be recoverable once investments are made. You are responsible for conducting your own research (DYOR) before making any investments. Read more here.