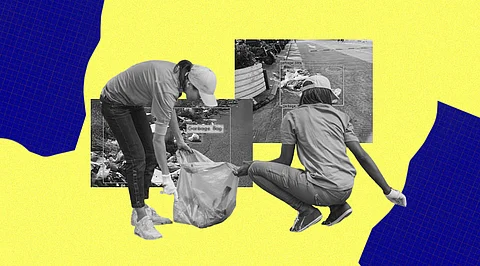
Litter is one product that never appears to experience supply chain issues. It is simple to locate, pick up, and appears to be everywhere. Sadly, the majority of us appear to view trash as someone else's issue, but you can at least contribute to the solution by using tools like this machine vision litter mapper. Everybody who has ever lived in an urban setting is aware of how dirty it can be. Municipalities make every attempt, yet rubbish still manages to move across towns like tumbleweeds. Simple solutions involve sending individuals with trash pickers to decontaminate city streets. Unfortunately, the location of trash is not always known. While trash accumulates in busier locations, street cleaners can wander through areas with lower densities of litter.
Many cities today have implemented a variety of strategies and made significant efforts to enhance the cleanliness of their streets. For instance, the cleanliness of public streets and sidewalks was assessed by New York City using an inspection program called Scorecard. The measurements are based on exacting photographic criteria of roadway and sidewalk cleanliness. To gauge the degree of street cleanliness in the city of Granada (Southern Spain), Sevilla et al. presented a cleanliness index in 2013. Intel et al. built an android-based application for the Bangladeshi capital city of Dhaka in 2015. The user themselves may help clean up their city, invite volunteers, or tell the city corporation. In 2015, Los Angeles has established a state-of-the-art street-by-street cleanliness evaluation system. The cleanliness of each street in Los Angeles has been mapped for the first time. Sanitation is better able to target locations with high demand and guarantee a fair distribution of services with the help of this new instrument. The majority of the existing technologies for the identification of litter are not entirely automated and still rely on human involvement, even though these approaches gave cities new ideas for cleaning their streets. To assess if the roadway is unclean, the cleaning staff must manually take and identify each image. Therefore, a potential and ideal system should, without human involvement, automatically and consistently detect trash in each collected image.
A team of four from North Carolina and Madrid created and maintains the Litterati app, which enables people in more than 115 countries to take images of garbage during clean-ups and submit them to a database that currently has more than 3.9 million pieces of litter recorded. With the help of a taxonomy C.O.M.B category (e.g., drink, food, personal hygiene), object (e.g., cup, bottle, can), material (e.g., plastic, metal, paper), and brand (e.g., McDonald's, Starbucks, Coca-Cola), Litterati aggregates all of these images, registers them, and then uses machine learning algorithms to gain insight into litter patterns, which are by nature spatial. Questions like: How does the weather affect the types of rubbish thrown? can be answered by their staff. using location intelligence. How does the kind of POI, such as a nightclub or a school, affect the frequency of littering?
The size of the issue was highlighted in 2017 when New York City set aside $32 million to control its growing rat population. Better garbage removal, which included spending money on 336 new solar-powered waste compactor bins and having municipal employees pick up trash more frequently, as well as greater enforcement and higher fines for unlawful dumping by private enterprises, was a crucial component of the solution. The newest garbage collection techniques utilized nowadays are high-tech and concentrated on picking up rubbish swiftly to minimize eyesores and avert vermin and sickness.
Las Vegas, Nevada, is one illustration. However, the gambling paradise does wish to maintain parks and public spaces free of litter and graffiti, especially in its downtown redevelopment district, which is distinct from the Vegas Strip. Although the city doesn't have significant rodent problems like much larger New York has, Las Vegas has created a high-tech strategy for maintaining the cleanliness of its downtown parks. To find garbage, graffiti, or other items that the city has marked in the AI software, an adaptive learning system employs video cameras and artificial intelligence (AI). This makes it possible to send out cleanup personnel when needed, which is less expensive than sending them out regularly. Las Vegas's in-house machine learning garbage collection.
The Las Vegas system, according to the city's director of information technology, Michael Lee Sherwood, has already been successfully implemented in two parks adjacent to the downtown area, and it may soon be adapted for use in other parks and on public roadways.
Sherwood outlined the process as follows: For that reason, real-time video streams from security cameras already installed in the parks are received by a cloud-based AI system on Microsoft Azure. The AI service developed by the city looks at the camera footage and makes judgments based on what the AI system has been taught to look for. If a park is considered to be dirty, a work order to clean it is created. Machine learning is used by many AI systems. Typically, a computer is repeatedly given photographs of a scene or an object in its ostensibly normal state. A computer may be configured to provide an alarm if it notices the same picture in an abnormal condition. The emergence of garbage is considered abnormal in Vegas. Utilizing thermal video footage of vehicles traveling the wrong way down a highway, police in the US states of Utah and Arizona are using AI to deliver automatic, real-time notifications to avert major collisions and fatalities. Other places, like Moscow's state-run clinics, have successfully utilized photographs of healthy lungs to compare to scans of lungs of persons suspected of lung cancer. While other AI researchers in Montreal are working to utilize high-resolution photos to determine when bridge spans are sinking, even slightly, over time, Montreal has experimented with using AI to discover sick street trees. Hence, Deep learning is very useful for detecting litter.
Smart garbage compactor bins from Bigbelly, for example, are used in several places across the world. Some of the trash cans that Bigbelly manufactures are solar- and wireless-powered, with sensors that can determine when a bin is full and transmit an alert for staff to come empty it. The business boasts collection reductions of up to 80%. The bins are frequently offered for use in areas with high foot traffic, such as cities and college campuses. City workers have occasionally had to adjust to on-demand labor schedules even though the bins don't detect rubbish lying about on parks and roads the way the Vegas application does.
If Bitcoin Fails to Claim its 200-Week MA, then BTC will See New Lows
Oracle Bangs US TikTok's Data Storage Deal! But Confused over Where to Store Data
Meta is Unleashing Profitable Tools for Metaverse Content Creators
Why Self-Taught Python Developers are Nobody's First Choice?
Github's AI-Powered Copilot Can Make Developers' Job Way Easier