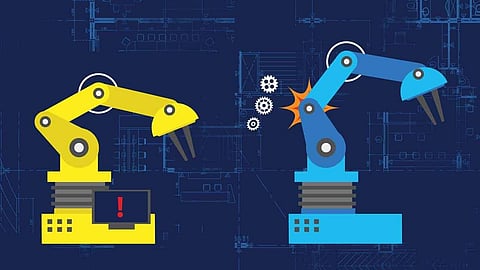
Predictive maintenance can provide measurable benefits for manufacturers who apply the technology on their production lines.
Predictive maintenance has become a growing requirement for industrial equipment operators responsible for preventing catastrophic system failures. Their ability to anticipate, and respond to, potential equipment malfunctions can enable them to preemptively schedule repairs and minimize overall disruptions to factory operation—ultimately benefiting the company's bottom line.
Yet skepticism still exists at times over whether predictive maintenance delivers measurable benefits. This doubt stems largely from companies that have difficulty determining what kind of ROI they will see from investing in the application and are unsure whether they have the proper amount of equipment failure data or even the right data to achieve a functional algorithm. Often predictive maintenance has been mislabeled as a "black box" solution, in which an application receives operational data from equipment and uses an algorithm to somehow predict the remaining useful life of a machine. But this is an inaccurate representation as it neglects the role domain knowledge plays in developing algorithms that can detect and predict failures.
Data scientists with mathematics backgrounds are the ones traditionally involved in predictive maintenance. Yet, they often lack the domain knowledge already existing within the engineer community. There is a great opportunity for companies to bridge the data science and engineering communities to generate the necessary equipment failure data that can better train predictive maintenance algorithms. Software simulation tools ease this process, as they can make predictive maintenance algorithms more powerful and ensure they need less data overall to be properly trained. These tools also enable those who may not be as familiar with predictive maintenance to implement different techniques to collect and train data.
To train these algorithms, companies need to know what failure data looks like. However, this kind of data is typically unavailable given equipment doesn't break down with great frequency and it's costly to run equipment to intentionally fail for the benefit of collecting failure data. To address this barrier, software tools such as simulation models help generate failure data by representing how physical equipment functions in the field across different testing scenarios. Here are three recent applications to demonstrate the approach:
• Oil field service company Baker Hughes used software tools to develop pump health monitoring software that uses data analytics for predictive maintenance. As a result, the company reduced equipment downtime costs by as much as 40 percent, while cutting down the need for extra trucks onsite.
• Packaging and paper goods manufacturer Mondi developed a health monitoring and predictive maintenance application with software tools to identify potential equipment issues. With these tools, the system was ready in a matter of months, despite the company's lack of data scientists with machine learning expertise.
• High-tech industrial group Safran (Spain) used simulation models to train a neural network used for active monitoring and prediction of anomalies of a hydraulic press. Using simulation models to generate data representing faulty machines allowed them to overcome the difficulty posed by a lack of real failure data from their machines.
This makes it clear that there is a great opportunity for companies to bring together their data science and engineering communities to generate the necessary equipment failure data through engineering simulation tools, and then use that data to better train predictive maintenance algorithms. Software simulation tools simplify this process, as they can make predictive maintenance algorithms more powerful and ensure they need less data overall to be properly trained. These tools also enable those who may not be as familiar with data science to implement different techniques for preprocessing data and training predictive models based on this data.
Currently, most predictive maintenance algorithms are onsite and close to equipment—like an edge server that collects data locally in a production facility or wind farm. Over the next few years, companies should expect to see rapidly increasing calculation power of industrial controllers and edge computing devices, as well as the use of cloud systems, to help achieve a new dimension of software functionality on production systems. Predictive maintenance will evolve and consider data not only from one machine or site but also across multiple factories and across equipment from different vendors. Depending on the requirements, these AI-based algorithms will be deployed on non-real-time platforms as well as on real-time systems such as programmable logic controllers (PLCs).
Ultimately, the most powerful use of predictive maintenance will be for feeding data from equipment all over the world into a cloud platform. The cloud allows manufacturers to both collect data from multiple areas and train predictive maintenance algorithms more efficiently, versus attempting to do the same locally. Despite some skepticism surrounding data security and ownership, companies should prepare for the realism of cloud-based predictive maintenance.
Predictive maintenance has several measurable benefits for manufacturers who apply this new technology on their production lines. Those who haven't determined how predictive maintenance can be monetized and fit into their respective business models risk being at a competitive disadvantage. However, there are resources available to enable the pairing of domain expertise and machine learning—meaning predictive maintenance and its benefits are within reach for every company.
Philipp Wallner,
Industry Manager, MathWorks
Join our WhatsApp Channel to get the latest news, exclusives and videos on WhatsApp
_____________
Disclaimer: Analytics Insight does not provide financial advice or guidance. Also note that the cryptocurrencies mentioned/listed on the website could potentially be scams, i.e. designed to induce you to invest financial resources that may be lost forever and not be recoverable once investments are made. You are responsible for conducting your own research (DYOR) before making any investments. Read more here.