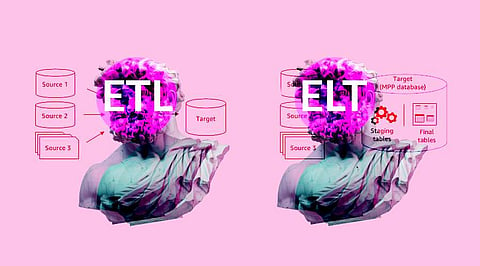
Meta launched Threads as a Twitter rival on July 6, 2023. And within the first 24 hours of the launch, the app registered over 30 million signups. Moreover, the proclaimed 'Twitter Killer' also reached the 100 million users bar in just five days, breaking the record of OpenAI's ChatGPT. However, the new microblogging platform has also paved the way for large data production, maintenance, and processing along with its launch.
The amount of data produced daily is ever-rising, with over 463 Exabytes of data to be created every day by 2025 globally. However, this huge chunk of data produced daily accompanies several challenges – capturing, storing, and analysing it properly for decision-making. And this is where understanding the difference between ETL and ELT processes becomes crucial.
The blog comprehensively covers the ETL vs ELT comparison along with their meaning, use cases and key differences to decide which is better.
ETL stands for Extract, Transform, Load and is a popular data integration strategy used by organisations worldwide. In ETL, the data is first transformed into a separate staging area and then loaded into the data warehouse(DWH) or the storage solution. This processed data is further used for analysis.
The data is first pulled from various sources (databases, applications, and files) during the Extract phase and then integrated during the Transform stage. Finally, during the Load stage, it is loaded into the data warehouse, whether cloud-based or on-site.
ELT, which stands for Extract, Load, Transform is another popular data integration strategy well-suited for companies dealing with large datasets and having cloud-based data warehouses.
The main difference between ETL and ELT in data warehousing lies in the process itself. In ELT, the data is first loaded in the DWH and then transformed as required for the analysis.
The main difference in ELT vs ETL is the order of data integration. However, there are other differences as well which must be considered before making the final choice:
ETL supports only structured and processed data in the data warehouse whereas, the ELT protocol enables both structured and unstructured data. Furthermore, ETL does not let raw data into the data warehouse while ELT transfers raw data directly to the warehouse.
ELT is relatively faster than ETL because it allows direct data transformation in the data warehouse. While ETL carries transformation on a separate processing server before loading.
The difference between ETL and ELT in data warehousing is that ETL is suitable for on-premise data warehouses while ELT is suitable for both cloud-based and on-premise warehouses.
ETL can be costlier than ELT in terms of storage solutions as on-site storage is more expensive than cloud-based storage. However, most ELT tools charge per query and running large queries can become expensive.
The ETL process is suitable for complex but small datasets while the ELT process works well for large pools of data.
Benifits | Drawbacks |
Loaded data is analytics-ready | Set-up cost is high |
Enhanced safety compliance | Slow loading & processing speed |
Widely adopted | Lack of flexibility |
Suitable for low storage | Unsuitable for large datasets |
Benifits | Drawbacks |
Faster processing and loading speed | Slower analysis |
More analytics options | High cost per query |
Loads raw data directly to the warehouse | Low safety compliance |
Supports large amounts of data | Relatively less adopted |
Low set-up cost |
|
A good ETL example can be a reporting system developed by an eCommerce company to track customer data from its various stores. The company can collect data from their stores and websites, transform it and store it in a data warehouse for analysis and decision-making.
Organisations like Walmart and Amazon use ETL protocol for their data integration needs.
The stock market is a common example of the ELT strategy. Since a large amount of data is generated in real time, the ELT protocol enables storing and analysis of the data faster.
Many cloud-based companies like Netflix and Spotify also prefer the ELT strategy to analyse the data directly in the DWH.
Traditionally, companies used ETL for their data integration needs. However, the emergence of cloud data warehouses gave rise to the ELT process which led to ETL vs ELT. ELT provides faster and unlimited loading of raw data and less maintenance than ETL. However, ETL also provides faster analytics and compliance with safety protocols like GDPR.
So, both the ETL and ELT processes have their strengths and weaknesses and increase data visibility across the organisation. Hence, it is advisable to assess both strategies properly to decide which is better.
In the ETL (Extract, Transform, Load) process, the data is transformed before it is loaded into the data warehouse. On the other hand, in the ELT (Extract, Load, Transform) process, the data is first loaded into the data warehouse and transformed later depending on the use case.
ETL and ELT transfer the data from databases and the business applications to the data warehouse. But, in the reverse ETL process, cleaned data is extracted from the warehouse and transferred to business applications for business operations.
A classic ETL example is the reporting system developed by companies for collecting data to make business decisions. Some other ETL use cases include collecting financial and consumer data for analysis and integrating data from various sources.
A stock exchange is a perfect example of the ELT process because it stores and analyses large amounts of data in real-time. Other ELT use cases are big data processing, collecting large datasets, and integrating data from various sources.
ELT is a new technology and hence has some advantages over ETL. However, many people still prefer ETL due to its developed infrastructure capable of handling complicated transformations.
Join our WhatsApp Channel to get the latest news, exclusives and videos on WhatsApp
_____________
Disclaimer: Analytics Insight does not provide financial advice or guidance. Also note that the cryptocurrencies mentioned/listed on the website could potentially be scams, i.e. designed to induce you to invest financial resources that may be lost forever and not be recoverable once investments are made. You are responsible for conducting your own research (DYOR) before making any investments. Read more here.