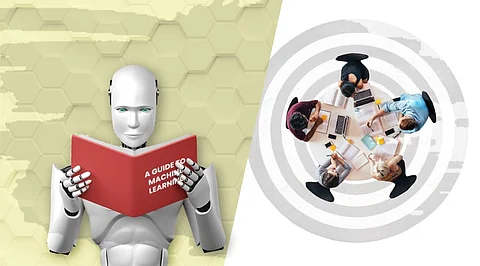
Artificial Intelligence and other disruptive technology are spreading their wings in the current scenario. Technology has become a mandatory element for all kinds of businesses across all industries around the globe. Let us travel back to 1958 when Frank Rosenblatt created the first artificial neural network that could recognize patterns and shapes. From such a primitive stage we have now reached a place where machine learning is an integral part of almost all softwares and applications.
Machine learning is resonating with everything now, be it automated cars, speech recognition, chatbots, smart cities, and whatnot. The abundance of big data and the significance of data analytics and predictive analytics has made machine learning an imperative technology.
Machine learning, as the name suggests is a process in which machines learn and analyze the data fed to it and predict the outcome. There are different types of machine learning like supervised, unsupervised, semi-supervised, etc. Machine learning is the stairway to reach artificial intelligence and it learns from algorithms based on the database and derives answers and correlations from them.
Machine learning is an integral part of automation and digital transformation. In 2016, Google introduced its graph-based machine learning tool. It used the semi-supervised learning method to connect clusters of data based on their similarities. Machine learning technology helps industries identify market trends, potential risks, customer needs, and business insights. Today, business intelligence and automation are the norms and ML is the foundation to achieve these and enhance the efficiency of your business.
A term identified by Gartner, Hyperautomation is the new tech trend in the world. It enables industries to automate all possible operations and gain intelligent and real-time insights from the data collected. ML, AI, and RPA are some of the important technologies behind the acceleration of hyperautomation. AI's ability to augment human behaviour is aided by machine learning. Machine learning algorithms can automate various tasks once the algorithm is trained. ML models along with AI will enhance the capacity of machines and software to automatically improve and respond to changes according to the business requirements.
According to Industry Research, the Global Machine Learning market is projected to grow by USD11.16 billion between 2020 and 2024, progressing at a CAGR of 39% during the forecast period.
This data is enough to indicate the growth and acceptance of ML across the world. Let us understand how different industries are using ML.
Other industries leveraging ML include banking and finance, cybersecurity, manufacturing, media, automobile, and many more.
Executives and C-Suite professionals should consider it a norm to have a strategy or goal before putting out ML into practice. The true capability of this technology can only be extracted by developing a strategy for its use. Otherwise, the disruptive tech might remain inside closed doors just automating routine and mundane tasks. ML's capability to innovate should not be chained just to automate repetitive tasks.
According to McKinsey, companies should consist two types of people, quants, and translators to unleash the power of ML. Translators should be the ones connecting the vague lines between the complex data analysis by algorithms and convert it into readable and understandable business insights for the executives.
Machine learning is not an unfamiliar technology these days, but it still takes time and patience to leave the legacy systems behind and embrace the power of disruptive technologies. Companies should focus on democratizing ML and data analytics for their employees and create a transparent ecosystem to leverage the capabilities of these techs by demystifying them.
Join our WhatsApp Channel to get the latest news, exclusives and videos on WhatsApp
_____________
Disclaimer: Analytics Insight does not provide financial advice or guidance. Also note that the cryptocurrencies mentioned/listed on the website could potentially be scams, i.e. designed to induce you to invest financial resources that may be lost forever and not be recoverable once investments are made. You are responsible for conducting your own research (DYOR) before making any investments. Read more here.