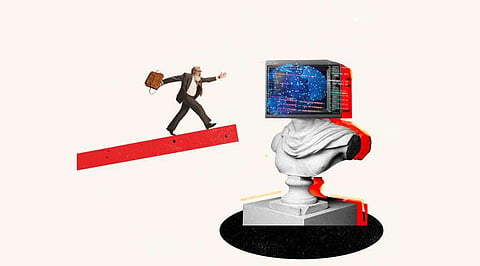
Machine learning (ML) has evolved from a futuristic concept to a cornerstone of modern technological advancement. Organizations across various sectors leverage ML to drive insights, automate processes, and make informed decisions. However, the path to successful ML implementation is challenging. Avoiding common mistakes is pivotal to harnessing the true power of ML and ensuring desired outcomes.
In this article, we'll explore five prevalent mistakes that can hinder the effectiveness of ML projects. By understanding and evading these errors, businesses, and individuals can navigate the complexities of ML implementation more effectively, unlocking its transformative potential. Let's delve into the critical mistakes to sidestep on the journey to successful ML integration.
Data quality forms the bedrock of successful machine learning projects. Using complete, accurate, or biased data can severely impact the reliability of your models. When data is compromised, it leads to unreliable predictions and flawed insights.
Additionally, address potential biases in the data that can skew the results. A comprehensive understanding of your data's quality and integrity ensures that your models are built on a solid foundation, producing more accurate and meaningful outcomes.
Clearly defining the problem you intend to solve is crucial. This step is necessary to avoid wasted efforts and irrelevant solutions. Without a well-defined problem, you might build models that don't align with your needs.
Set measurable success criteria and ensure the ML solution effectively addresses the core challenge. By giving due diligence to problem definition, you lay the foundation for focused and impactful machine learning implementations.
EDA helps understand the data's characteristics and relationships, aiding in feature selection and model design. Ignoring this step might result in better feature choices or insights. Visualize data, identify outliers, and ensure a comprehensive understanding before modeling.
Selecting overly complex models without justification can lead to overfitting—where models perform well on training data but fail on new data. Strive for a balance between complexity and generalization using regularization and cross-validation techniques.
Failing to evaluate models thoroughly can lead to adopting suboptimal solutions. Use metrics like accuracy, precision, recall, and F1-score to assess performance. Employ validation and test sets to simulate real-world scenarios and validate results objectively.
Join our WhatsApp Channel to get the latest news, exclusives and videos on WhatsApp
_____________
Disclaimer: Analytics Insight does not provide financial advice or guidance. Also note that the cryptocurrencies mentioned/listed on the website could potentially be scams, i.e. designed to induce you to invest financial resources that may be lost forever and not be recoverable once investments are made. You are responsible for conducting your own research (DYOR) before making any investments. Read more here.