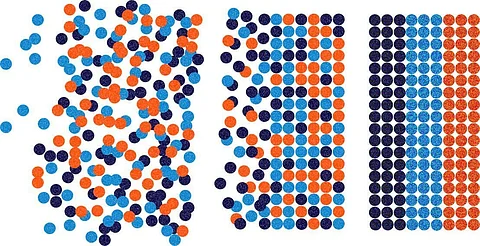
Fraud attacks are getting to be progressively sophisticated– as innovation advances, fraudsters have raised their game on payment fraud and money laundering. With access to faster and less expensive computing, fraudsters have moved their objectives to more beneficial weaker points in the financial services chain. 65% of companies with yearly incomes of $1 billion were victims of payments fraud in 2014 contrasted with 56% of organizations detailing yearly incomes of under $1 billion.
The adoption of machine learning has been accelerated with increasing processing power, accessibility of big data and headways in statistical modeling. Fraud management has been excruciating for banking and business industry. The quantity of transactions has expanded because of plenty of payment channels, credit/debit cards, cell phones, kiosks. Simultaneously, lawbreakers have gotten capable of discovering loopholes. Thus, it's getting intense for organizations to assess transactions. Data scientists have been fruitful in taking care of this issue with machine learning and predictive analytics.
Automated fraud screening frameworks controlled by machine learning can help organizations in lessening extortion. Actually, of the numerous issues that ML vows to fathom, online fraud detection has been one of the earliest examples of overcoming adversity. Before deploying ML for fraud prevention, let's look at some of the key pointers to keep in mind for successful implementation.
Since composed crime schemes are so sophisticated and snappy to adjust, protection systems dependent on any single, one-size-fits-all analytic strategy will deliver trashy outcomes. Each use case ought to be upheld by expertly made anomaly detection techniques that are ideal for the current issue. Subsequently, both supervised and unsupervised models play significant jobs in fraud recognition and must be woven into complete, cutting edge fraud strategies.
A supervised model, the most well-known type of ML overall controls, is a model that is trained on a rich arrangement of appropriately "labeled" transactions. Every transaction is labeled as either fraud or non-fraud. The models are trained by ingesting gigantic amounts of labeled transaction subtleties so as to learn patterns that best reflect real practices. When building up a supervised model, the amount of clean, relevant training data is straightforwardly connected with model accuracy.
Unsupervised models are intended to spot odd behavior in situations where labeled transaction information is moderately slim or non-existent. In these cases, a type of self-learning must be utilized to surface patterns in the information that is undetectable to different types of analytics.
By picking an ideal mix of supervised and unsupervised AI procedures you can identify beforehand inconspicuous types of suspicious behavior while rapidly perceiving the more unpretentious patterns of fraud that have been recently seen across billions of records.
Organizations need to create clear meanings of fraud, label their data, and guarantee each name neatly reflects set definitions. ML techniques are normally sympathetic to random labeling errors in the training set, yet truly vulnerable to methodical mistakes. Dissimilar to training, teams must attempt to fix even the most random labels in the test and validation sets, to make them reliable enough to evaluate the quality of their models.
Fraud teams are rivaling fraudsters who are getting progressively sophisticated in reproducing customer personalities. The most ideal approach to get these fraudsters is to assemble unique information from different merchants and partners and discover remarkable traits that distinguish the genuine human behind the digital character. Use every one of the information that could help with risk signaling, including device, personality, individual, and network behavioral patterns.
A centralized data repository will guarantee the data science team realizes what's accessible and can use it. Teams additionally should focus on keeping customer data secure. Pursue principles lined up with EU General Data Protection Act (GDPR, for example, gathering the data that companies will use to serve the clients' needs, just storing it until the time it is required for preventing fraud and giving clients full oversight of their information. To drive customer trust, organizations need to truly have confidence in these standards, not simply check the box.
Fraudsters guarantee that protecting customer's records is perplexing and dynamic, a challenge where AI flourishes. For constant performance improvement, fraud detection professionals should consider adaptive innovations intended to hone responses, especially on marginal choices. These are the transactions that are near to the investigative triggers, either simply above or just beneath the cutoff.
It is on these edges where accuracy is most crucial as there is a scarcely discernible difference between a false positive event (a genuine transaction which has scored excessively high) and a false negative event (a fake transaction which has scored excessively low). Adaptive analytics hone this distinction with exceptional learning of the threat vectors an establishment is confronting.
Adaptive analytics technologies improve sensitivity to moving fraud trends via naturally adjusting to recent confirmed case disposition, bringing about a progressively exact division between frauds and non-frauds. At the point when an investigator explores a transaction, the result, regardless of whether the transaction is affirmed as real or fake, is nourished over into the framework to precisely reflect the extortion environment that analysts are confronting, including new strategies and unpretentious fraud patterns that have been dormant for quite a while.
This adaptive modeling technique consequently adjusts the loads of predictive features within the basic misrepresentation models. It is an incredible asset that improves fraud discovery performance on the edges and stops new kinds of fraud attacks.
Realizing that machines and people have altogether different abilities, the best ML frameworks leverage these distinctions. People can deal with anomaly cases that probably won't have enough historical information or circumstances that require critical careful decisions. For instance, a business might be getting orders from another geology or displaying an exceptional behavior pattern. It is worth getting people engaged with these cases before summing up the outcomes to another ML model.
Use bi-directional feedback to improve both the machine and human sides. Human input improves model predisposition and upgrades the logic of models. Simultaneously, ML models can give extra data to make the human's tasks simpler or even help improve human aptitudes.
The human-level performance of an experienced manual review team is a sensible estimation of the best attainable model performance. Subsequently, a high gap between the model training mistake and the human-level blunder is an indicator that teams need to decrease the model bias.
Join our WhatsApp Channel to get the latest news, exclusives and videos on WhatsApp
_____________
Disclaimer: Analytics Insight does not provide financial advice or guidance. Also note that the cryptocurrencies mentioned/listed on the website could potentially be scams, i.e. designed to induce you to invest financial resources that may be lost forever and not be recoverable once investments are made. You are responsible for conducting your own research (DYOR) before making any investments. Read more here.