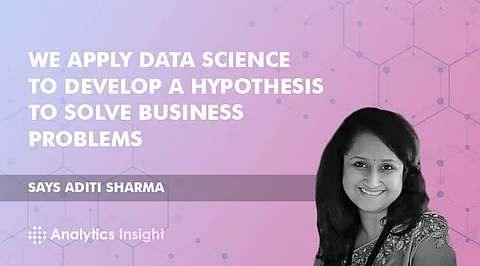
Shoppers, locally or globally, make purchases because they need something, or because they feel an emotional connection to it, and sometimes out of peer pressure. This is the common knowledge a well-minded retailer has about its customers. But does he know why certain purchases don't happen, the purchases that depend on long-range weather forecasts, household expenditures, property prices, or bank holidays? It's a hard bargain to guess such instances. Now that data-centric decisions are making these guesses possible, retailers and brands are heavily depending on data-derived customer sentiment to make great sales and grow brand value simultaneously. dunnhumby Ventures, a Data Science solutions company, says with its seed-stage retail technology, helps companies not just have 'customer centricity' but to live with it. Analytics Insight has engaged in an exclusive interview with Aditi Sharma, Applied Data Science Director at dunnhumby.
dunnhumby is a global leader in customer data science. We deal with transactions from nearly 800 million shoppers around the world and use our specialized knowledge of machine-learning algorithms to solve problems for retailers & consumer-packaged goods (CPGs). We strive to understand customer preference through their shopping behavior and attitudes, eventually supporting the retailer and brands to increase customer engagement and thus achieve financial targets (profits). We have hundreds of models and techniques powering our propositions through which we deliver value to customers. This could be in form of convenience, monetary benefits, and satisfaction. This in turn helps the retailers increase customer engagement through customer-first strategies and helps brand/CPGs through increased return on investment and higher brand awareness. Apart from providing insights into retail data, our media solutions leverage this data and use data science to run effective targeting and personalized promotional campaigns for CPGs. Such science-driven campaigns result in a win-win-win situation for the customer, CPG, and the retailer. We hold a unique position as we cater to a global client base and thus bring in learnings and efficiencies from each other.
Retail is a complex industry with several value levers such as inventory management, supply chain operations, and marketing campaigns. Our primary audience consists of store operations, marketing, and merchandising. On a typical day, we engage with commercial teams and client stakeholders to solve business problems using state-of-the-art statistical techniques and machine learning algorithms. We design innovative custom approaches when existing solutions and traditional techniques fail to address the uniqueness of clients' problem statements and their data.
We start by simplifying and defining the problem statement that the retailer needs to address. Once that is defined, we use sophisticated statistical tools and technology, our years of trained coding skills, and ML algorithms to refine the big data of customers' transactions. This refined data is then structured and visualized to derive insights. This is then combined with business context, helping us give recommendations that ultimately enable the client to take "Customer First" decisions. We do all this through three pillars:
dunnhumby has about 30 years of experience working with retailer first-party data to improve the experience for customers. We help retailers and brands create relevant experiences for their customers that in turn deliver profitable growth. We bring the benefit of our global industry knowledge to our clients and help retailers adopt customer-first ways of working through:
It's not enough to just talk about 'customer first' –an organization must LIVE 'customer first' – make it a part of their culture and core to be able to grow. Our strength lies in becoming an effective bridge to achieve that.
We have solved several new problem statements for retailers & explored new data sources. With eCommerce accelerating post-pandemic, customer behavior has altered for good. The in-store and online shopping compliments each other now vs. competing. Navigating through this, we gain new expertise in understanding multichannel engagement and looking through clickstream data for search results analysis, device performance, or taxonomy optimization. We as an organization continue to evolve and optimize our algorithms to make measurable improvements – be it answering acquisition related problem statements through semi-supervised binary classification techniques or measuring the impact of assortment changes through predictive science, in absence of test & control setup. Our increased use of visualization tools (such as PowerBI) to share results and recommendations through cloud-based dashboards & reporting speaks about our focus on gaining efficiency. Speaking of efficiency, making our models scalable and automated is another innovation win. Since we are dealing with billions of data points, some data science models could take even days to run through and give results and how we could do this at a fraction of the original time taken becomes our critical focus area.
The growth in big data analytics comes from three different angles. First is the explosion of the data. Almost every action is a data point that can be analyzed. Even our oxygen levels are monitored through our smartwatches. We all are living and breathing data. Analytics is all about making that data talk. Speaking from the retail industry & dunnhumby' s perspective, to start with, there is invaluable information on social media that is being tapped through and can be explored further to understand customers' sentiments and engagements. Using transactional data gives a partial picture of what is happening in-store and this is where elements of video analytics have great potential. All this requires a strong technological setup and infrastructure to succeed. This takes me to the second angle of growth – faster & better technologies. In the past decade, I have seen a very positive evolution of technology in analytics. Starting my career with SAS, where data preparation used to take weeks, and now moving to a setup where it is a matter of hours to do the same is one such example. And this doesn't stop here. We are now talking about everything being real-time – be it offers on products you buy or recommendations on what goes well with the outfit you just bought. With the world moving faster, there is much more coming our way, which is the third factor to create growth – market dynamics, especially post-pandemic. The world opened after the pandemic to difficult economic conditions. The inflationary pressure is not only a macroeconomic trend but a dynamic that impacts customers' behavior day in and out – making it an important area for analytics.
Growth in data science or analytics job opportunities is a well-known fact. The greatest testimony is the increasing number of available courses – not just as regular curriculum (MBA courses with a focus on analytics), but also distance/part-time learning to increase the workforce in the domain. Irrespective of size and location, the industry needs to analyze big data to stay relevant and progress at the same time.
A simple search from the internet throw mind-boggling numbers (some from recent search):
These are just some of the most used applications in our day-to-day lives. We have about 2.5 quintillion bytes of data created every day which has so much to speak. The world is waiting for specialists to translate them into actionable insights and recommendations. Leveraging digital age data and improving offerings requires the right skill set and thus opportunities in the field span from data wrangling to visualization to algorithm implementation to storytelling. The complete value chain of analytics has numerous opportunities and the potential to grow.
Join our WhatsApp Channel to get the latest news, exclusives and videos on WhatsApp
_____________
Disclaimer: Analytics Insight does not provide financial advice or guidance. Also note that the cryptocurrencies mentioned/listed on the website could potentially be scams, i.e. designed to induce you to invest financial resources that may be lost forever and not be recoverable once investments are made. You are responsible for conducting your own research (DYOR) before making any investments. Read more here.