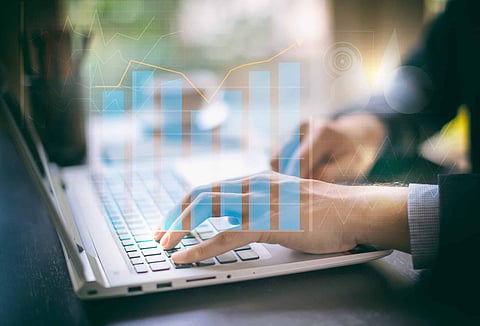
Predictive analytics demands a successful plan of design, creation, validation and implementation for variety of applications in the industries. There is a need for predictive analytics to converge with the business goals which helps each step to be related to the company-specific business needs and objective. The emphasis on business goal highlight the process to be more robust and pragmatic. The following are the steps for an effective predictive analytics strategy:
Framing the objective of a project is a very crucial step before implementing predictive analytics modeling. Before the start of the project, it is essential to align the model objective function in relation to the business goal as well as the overall strategy of the firm. It is also important to include the risk associated with a particular type of modeling along with the expected outcomes and lucid deliverables as well as the input which should be used. List down all the data sources to be used and the expected format for the analysis.
The three A's of data – accurate, actionable, accessible is a significant characteristic required for a successful model. There are numerous sources for data both internally and externally. There are generous number of ways to collect and generate valid samples for model development. Multiple scenarios in the industries describe data for acquisition, retention, risk, cross-selling and up-selling.
Most data scientists spend their 60% of their time prepping the data. The entire data preparation process starts with the description of different classifications of data and how they can be involved in predictive modeling. Several techniques are introduced for handling common data problems such as missing values and outliers.
Determining the best fit for a model is essential for a good performance. It is important to understand the underlying relationship of the independent variable to a dependent variable which helps to determine the power and longevity of the model. Processes like binning and transforming independent variables help to deliver the best fit with the dependent variable. Several programs can be coded which will automatically segment and transform the powerful variables and ensure the best fit. This helps the selection process to combine easily with the variables into the final stage of modeling.
The preparation groundwork laid so far helps to work this level seamlessly. When it reaches modeling it is important to use existing tools. There are numerous libraries, built on open sourcing programming languages like python and R. These libraries helps to determine the best fit for the model and is used to capture the information and display the data.
Models should perform justice to the development of data and the model performance should score with the validation data. A true test of the model performance is how well it delivers the result from real-time data from the market. There are generally three powerful models for ensuring the model fit, (i) scoring the alternate data is the best way to check whether the model will perform (ii) Bootstrapping uses simple resampling techniques to find confidence intervals around the estimates (iii) key variable analysis calculates the important market factors that might affect the model, thus reinforcing reasonable result.
Efficient implementation is a combination of business intelligence and well-designed practices. After the model is being calibrated, the results need to be interpreted and integrated into the business practices. Once the implementation is finished, the model should be incorporated into the daily rules and governing processes in the organization.
Join our WhatsApp Channel to get the latest news, exclusives and videos on WhatsApp
_____________
Disclaimer: Analytics Insight does not provide financial advice or guidance. Also note that the cryptocurrencies mentioned/listed on the website could potentially be scams, i.e. designed to induce you to invest financial resources that may be lost forever and not be recoverable once investments are made. You are responsible for conducting your own research (DYOR) before making any investments. Read more here.