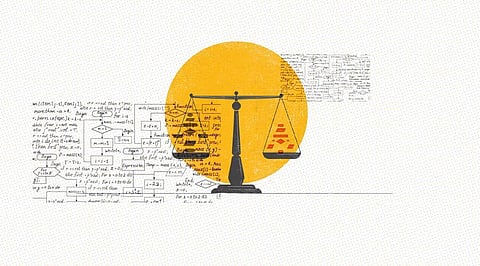
While businesses cannot completely remove bias from their data, they may greatly minimize bias by putting in place a governance framework and hiring a more diverse workforce. It's in our human nature to be biased. Each of us has distinct viewpoints, interests, and likes and dislikes. Therefore, it should come as no surprise that these biases can be detected in data.
Biased data can lead to distorted or incorrect machine learning (ML) models if left unchecked. Organizations may better understand their customers, manage their resources, streamline processes, and respond to ongoing market changes with the use of data. This data is more crucial than ever as businesses use AI and ML more and more.
Data can, however, also inject biases into ML models, and these biases might be challenging to identify. Each stage of the AI process has the potential to inject bias into algorithms in different ways. From data gathering efforts to data processing, analysis, and modeling, each stage brings a unique set of difficulties and chances for unintentional bias introduction into an ML model, training data set, or analysis.
Businesses need to be aware of the various biases in their data that could find their way into their machine-learning models. Organizations may identify and possibly fix some of the problems causing skewed, erroneous, or unsuitable outcomes for the machine learning models by understanding the different types of bias that may present.
Many contemporary businesses gather data in both organized and unstructured formats, in a variety of formats or modalities, including numerical, graph, text, image, and audio data. Bias can be introduced into the data collection process employed by businesses, and it can also be present in the language used in each of these many data forms. For instance, erroneous input data from a mislabeled graph may result in skewed results from a machine learning model.
Data collection frequently contains biases that cause some groups or categories to be overrepresented or underrepresented. This is particularly true when several data sets are merged to be used in aggregate. For smaller datasets, anomalies can be detected, but for larger datasets with millions or billions of data points, anomalies are very challenging to detect.
As a result, the models have bias, preferring or disfavoring particular data categories. When some data types are overrepresented in the data or, conversely, when other datasets are undervalued relative to their actual incidence in real-world data collection, modeling bias can happen.
Even when factors like gender, color, locality, and sexual orientation are eliminated, AI systems learn to make conclusions based on training data, which can include biased human decisions or reflect historical or social imbalances.
Businesses can more effectively identify and remove bias in their data by recognizing common data biases. In all stages of their data pipeline, organizations should consider ways to minimize the likelihood of skewed data sets.
Since not all data have an equal representation of the data pieces, there are various opportunities for bias to be introduced during the data collection process. Some sources might offer data that is insufficient, while others might not accurately reflect the real world or your modeling data set.
Biases can also be introduced during data processing, including data preparation and data labelling. The replacement of outdated or duplicate data is a part of data preparation. Businesses run the danger of unintentionally eliminating critical data, even though this might assist remove unnecessary data from training sets. Data anonymization, which removes personally identifiable information like a person's ethnicity or gender, contributes to privacy protection and makes it more challenging to identify or correct bias based on those variables.
Adding labels to unstructured data enables a computer to interpret and comprehend it. This technique is known as data labelling. Data labelling, however, depends on both people and technology. A human data labeler may add bias to the data if they incorrectly label a picture or use their own judgment when translating or tagging. Organizations should make sure they have established checks and balances and don't rely only on one system or data labeler for all human-based data labelling decisions in order to reduce errors.
Both false positives and false negatives can occur when using AI models. When examining whether data is biased, it's crucial to keep these measurements in mind, especially when certain groups show excessive susceptibility to false positives or false negatives. Organizations can improve model accuracy and precision by experimenting with a variety of modelling methodologies, various algorithms, the use of ensemble models, adjustments to the hyperparameters, and other aspects.
Join our WhatsApp Channel to get the latest news, exclusives and videos on WhatsApp
_____________
Disclaimer: Analytics Insight does not provide financial advice or guidance. Also note that the cryptocurrencies mentioned/listed on the website could potentially be scams, i.e. designed to induce you to invest financial resources that may be lost forever and not be recoverable once investments are made. You are responsible for conducting your own research (DYOR) before making any investments. Read more here.