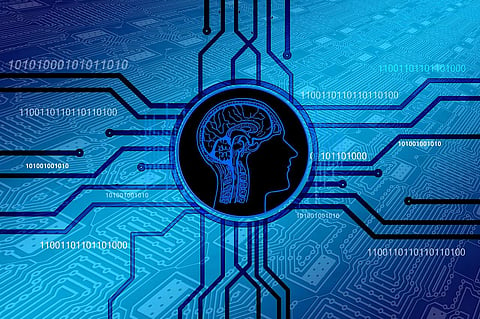
Big data, artificial intelligence and machine learning are ruling the tech structure of most industries. We all know how Amazon combines a customer's historical data and other customers' data to power recommendations. Likewise, for Google, it's not difficult to predict our preferences and interests. They make use of big data, analytics and machine learning to be able to process huge amounts of data, identify patterns, analyze them and consequently indulge in predictive analysis.
The most complicated disease of the most important organ of the body – the brain, is a clear beneficiary of this AI approach. In cases of a stroke or a brain injury, two million cells a minute get damaged in the brain due to lack of oxygen. One of the most commonly used diagnostic tools in the emergency rooms is the head CT scan for patients with injury or symptoms of stroke. Immediate imaging and interpretation of CT scans in case of patients suspecting internal hemorrhage is critical.
More often than not, radiologists have a backlog of cases and it might be too late before a case's criticality gets detected. Abnormalities are found only in a small number of cases but these are time sensitive. Automating the initial screening and triage process to streamline the CT scan interpretation workflow, will significantly decrease the time to identify critical cases. The automation would lead to an efficient queue management system in busy traumatic centers. Not only in busy centers, even in remote areas, immediate help and advice of a radiologist can be sought.
Qure.ai, a company that develops deep learning solutions to assist physicians, has come up with automated deep learning algorithms in radiology to detect abnormalities requiring urgent attention. These include intracranial hemorrhage, mass effect, cranial fractures and midline shift.
The deep learning algorithms have been trained using labeled scans and the dataset used was large and from diverse sources. In the past as well, deep learning has been used for image interpretation purposes, grading purposes and identifying whether a particular tumor or lesion is benign or malignant. The algorithms' decisions have been proved to have an accuracy equal to that of a specialized physician. In radiology itself, classification algorithms have been used in cases of chest radiographs, head CT's to localize and quantify disease patterns.
NVIDIA's TITAN X and GeForce GTX 1080 GPU's along with cuDNN (accelerated Pytorch deep learning framework), were used by Qure.ai to train their algorithms on more than 30,000 head CT scans and results. In comparison to the 97 percent accuracy rate achieved by a panel of three senior radiologists, the algorithms achieved an accuracy rate of 95 percent.
Radiologists are in demand everywhere but there is a shortage due to difficulty in accessibility and high costs. For instance, for every 275,000 people Kenya has one radiologist and in India has one for every 65,000 people. A highly developed country like the UK is also struggling with figures of 7.5 radiologists for every 100,000 people.
Qure.ai has made its solutions available to many centers across India and is still seeking the FDA approval to implement its solutions in three U.S. centers. Tests across the European Union medical institutes have been planned as well. Qure.ai is working towards its goal of getting cost-effective radiological services to regions radiologists deprived regions.
Join our WhatsApp Channel to get the latest news, exclusives and videos on WhatsApp
_____________
Disclaimer: Analytics Insight does not provide financial advice or guidance. Also note that the cryptocurrencies mentioned/listed on the website could potentially be scams, i.e. designed to induce you to invest financial resources that may be lost forever and not be recoverable once investments are made. You are responsible for conducting your own research (DYOR) before making any investments. Read more here.