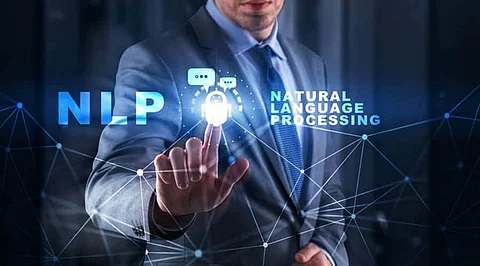
Healthcare companies have more access than ever to information-driven innovation that can improve healthcare results and drive business opportunities. It is difficult for these enterprise systems to explore the trillions of gigabytes of health information and web content, however, Natural Language Processing (NLP) in healthcare is a promising segment of the solution.
NLP based chatbots as of now have the capacities of truly and genuinely emulating human behavior and executing a heap of tasks. With regards to implementing the same on a much bigger use case, similar to a hospital– it very well may be utilized to parse data and extract critical strings of data, along these lines offering an open door for us to use unstructured data.
Reviewing and merging this data to shape an exhaustive perspective on a member's health is a huge challenge for most health plans, yet it is fundamental to inform appropriate care management, research, reporting, and analytics.
Luckily, cutting-edge innovation is available that can augment and accelerate the labor-intensive procedure of extricating applicable clinical insights for high-value use cases.
Natural Language Processing in healthcare has penetrated into scientific documentation, freeing up doctors from the guide and confounded structure of EHRs(Electronic wellbeing record), allowing them to zero in increasingly more on care delivery. This has been feasible on account of speech-to-text dictation and formulated facts access that catch structures realities on the factor of care. As NLP in healthcare propels, we will have the option to pull relevant records from various arising resources and improve analytics used to drive PHM and VBC endeavors.
Significant data is scattered all through a member's diagram, which can be many pages long, and that makes physically auditing it for pertinent clinical information tedious for health plans. Generally, plans employ costly subject matter experts to physically peruse different records, look for keywords, and glue key discoveries into isolated structures. In a particularly significant exercise, guaranteeing that reviewers keep a steady degree of precision and culmination is crucial, adding to the administrative weight.
In evaluating NLP innovation to assist with the clinical chart review process, payers should search for partners that have a powerful library of clinical terms and acronyms, synonyms, shorthand, and incorrect spellings common in clinical notes to successfully recognize secretive clinical terms secured in unstructured content and appropriately codify them to standards. This will empower the identification of important context encompassing the data to accumulate a better clinical understanding.
Notwithstanding patient experience improvements, NLP can help healthcare companies oversee online reviews in an exceptionally managed industry.
Natural Language Processing innovation can gather and analyze the large number of healthcare reviews posted each day on third-party postings, finding protected health information (PHI), obscenity or other content pertinent to HIPAA compliance. It can likewise rapidly examine and assess human sentiment of unstructured remarks, alongside the context of how they are being utilized.
Numerous healthcare frameworks additionally use text analytics to screen the Voice of Consumer in reviews, so doctors see how patients talk about their care and can more readily communicate utilizing a shared jargon. Also, NLP frameworks can track consumer sentiment about your healthcare brand by pulling insights from positive and negative words or expressions within reviews or social media posts.
ID of high-risk patients, as well as progress of the analysis cycle, can be finished through conveying predictive analytics related to natural language processing in healthcare close by predictive analytics.
It's far fundamental for emergency departments to have whole data fast, within reach. For example, the deferral in analysis of Kawasaki diseases prompts significant difficulties in case if it's far overlooked or mistreated in any way. As demonstrated utilizing scientific impacts, a NLP based set of rules identified at-risk patients of Kawasaki disease with an affectability of 93. 6% and particularity of 77.5% compared with the guide overview of clinician's notes.
A bunch of researchers from France chipped away at building up every other NLP based algorithms that would screen, distinguish and save from medical institutions acquired contaminations (HAI) among patients. NLP helped in delivering unstructured records which changed into then used to become aware of early signs and symptoms and intimate clinicians accordingly.
Join our WhatsApp Channel to get the latest news, exclusives and videos on WhatsApp
_____________
Disclaimer: Analytics Insight does not provide financial advice or guidance. Also note that the cryptocurrencies mentioned/listed on the website could potentially be scams, i.e. designed to induce you to invest financial resources that may be lost forever and not be recoverable once investments are made. You are responsible for conducting your own research (DYOR) before making any investments. Read more here.