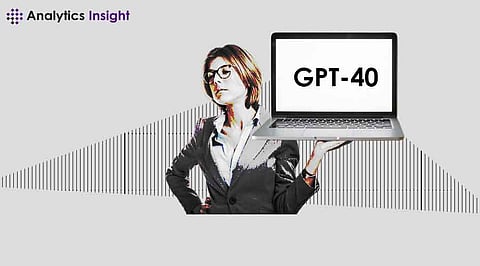
The ability to fine-tune AI models such as GPT-4o has become a crucial practice. Fine-Tuning GPT-4o refers to the process of adapting the base GPT-4o AI model to perform optimally on specific tasks or datasets. This customization can lead to significant improvements in model performance, making it more relevant and efficient for specialized applications.
However, fine-tuning AI models is not without its challenges. While the Benefits of Fine-Tuning GPT-4o are substantial, ranging from improved accuracy to enhanced task-specific performance, there are also complexities that need to be managed. In this article, we will explore the Benefits of Fine-Tuning GPT-4o, examine the Challenges of Fine-Tuning GPT-4o, and provide a comprehensive overview of the fine-tuning process.
Fine-tuning the GPT-4o AI model involves several steps, including:
Data Collection: Of getting a good quality fine-tuning dataset of domain specific data.
Preprocessing: To ensure the data was ready to be put through the model, the data was cleaned and standardized to fit the model needs.
Training: Fine-tuning is the process of modifying one or some of the given model parameters utilizing the fine-tuning dataset.
Evaluation: Validation data analysis concerning the performance of the fine-tuned model in compliance with the specified criteria.
Deployment: Dealing with the challenges that arise while deploying the fine-tuned model into applications or systems for functionality.
Fine-tuning GPT-4o offers a range of benefits that can significantly enhance the performance of AI models for specific tasks. Here are some key advantages:
1. Enhanced Performance on Specialized Tasks
The fact that one of the main advantages of Fine-Tuning GPT-4o is its ability to enhance performance on dedicated tasks is one of the first things that come to mind. The AI learning model refers to the usual GPT-4o which is fed a wide variety of data, so it possesses a flexible feature, yet it does not fit all the usages. Fine-tuning gives the model the chance to alter the parameters according to the target areas or mesmerizing businesses, which then results in generating more accurate and worthwhile responses.
For example, a GPT-4o AI model tuned specifically for medical text in the context of healthcare can supply more precise and contextually appropriate information compared to the general model.
2. Improved Relevance and Accuracy
The most relevant and accurate data can be retrieved by fine-tuning the GPT-4 AI model, which is done via training on the domain-specific dataset. It is the process by which the model is better able to understand the subject and create output that is in line with the particular industry, the trends, and the needs of the users' expectations.
For instance, fine-tuning for legal documents ensures that the model produces outputs with the appropriate legal terminology and context, making it a valuable tool for legal professionals.
3. Increased Efficiency and Effectiveness
Fine-tuning can also lead to increased efficiency and effectiveness of the GPT-4o AI model. Customization reduces the need for additional filtering and processing of outputs, as the model is already aligned with the specific requirements of the task at hand. This results in faster response times and more effective use of the model in practical applications.
4. Personalization and User Experience
A customized user experience is a side effect of fine-tuning AI which is not only a polar opposite of standard training but also makes it easy to segment the users. Users can now create a GPT-40 dynamic model that brings feedback much closer to their individual or organizational needs. This will, on the other hand, leave them satisfied and engaged. This personalization enhances user satisfaction and engagement.
5. Competitive Advantage
Implementing a GPT-4o AI model that is finely tuned can result in a business gaining a competitive edge. The differences in the nuances of the models can introduce special features and capabilities that will distinguish the products and services from the rest in the market. This competitive advantage can play an important role in areas where proficiency and precision are two of the most wanted qualities.
While the Benefits of Fine-Tuning GPT-4o are considerable, the process is not without its challenges. Here are some common hurdles associated with fine-tuning AI:
1. Data Quality and Quantity
The first and possibly the biggest of them is the need to have high-quality and at the same time, a sufficient quantity of training data as fine-tuning GPT-4o. Fine-tuning seems to necessarily call for a large amount of data in the related realm, sufficiently good to fine-tune the model. Acquiring and compiling such data may prove to be rather lengthy and even expensive in terms of work time.
If the fine-tuning involves low-quality or insufficient data, the quality of fine-tuning can be low, and it can cause overfitting; that is, the model performs well when tested on the training data but poorly when tested on new data.
2. Computational Resources
Since the tuning of a GPT-4o AI model is a complex process, a lot of computational power is used in the process. This involves retraining the model which takes time and is done with specific data sets and hence it calls for powerful hardware and a lot of processing capability. As we have seen, it can be a problem for the organizations that have not many resources to work with.
3. Overfitting and Generalization
The first threat is fitting the model to the fine-tuning data set more than it should be fitted, to yield an optimal performance. Overfitting is the condition where the model is learnt to a level where it performs best only on the training data and poorly on the general or unseen data. One of the most important considerations in fine-tuning is striking the right balance on the one hand not to over-optimise while retaining the ability to generalise on the other.
4. Maintaining Model Integrity
In fine tuning the model, it is important to avoid any loss of quality of the developed GPT-4o AI. It is desirable that with fine-tuning the model should be made better for the specific task without any noises being added or it loses its general capabilities. It becomes quite challenging have to deal with while also having to ensure that the model integrity is preserved and at the same time analyze how well or poorly specific fine-tuning objectives have been met.
5. Ethical and Bias Considerations
Updates also brings about ethical issues and bias if the data used for training is also not properly chosen. It is important to make sure that the model does not continue to put forward damaging bias, or the wrong idea. To handle these problems, audits and ethically-oriented reviews should be performed to keep the model free from biases and as accurate as possible.
Fine-tuning GPT-4o has several advantages, namely the strengthened capabilities of the system in specialized tasks, better relevance and accuracy, and higher efficiency. On the other hand, the process is not without challenges such as maintaining data quality, handling computational resources, and resolving ethical issues.
Investigating the Benefits of Fine-Tuning GPT-4o and the Challenges of Fine-Tuning GPT-4o is a core competence for exploiting this robust AI model. Through the collaboration of the mentioned issues and their solutions, organizations can accept the GPT-4o AI model for a variety of advanced applications where AI has been a major development.
1. What are the key benefits of fine-tuning GPT-4o?
The key benefits include enhanced performance on specialized tasks, improved relevance and accuracy, increased efficiency, personalization, and a competitive advantage.
2. What challenges are associated with fine-tuning GPT-4o?
Challenges include ensuring data quality and quantity, managing computational resources, avoiding overfitting, maintaining model integrity, and addressing ethical and bias considerations.
3. How does fine-tuning improve the performance of GPT-4o?
Fine-tuning adapts the GPT-4o AI model to specific tasks or domains, improving its accuracy and relevance by training it on specialized data.
4. What steps are involved in the fine-tuning process?
The fine-tuning process involves data collection, preprocessing, training, evaluation, and deployment of the model.
5. How can organizations overcome the challenges of fine-tuning GPT-4o?
Organizations can overcome challenges by ensuring high-quality data, using adequate computational resources, balancing fine-tuning to avoid overfitting, maintaining model integrity, and conducting regular ethical reviews.