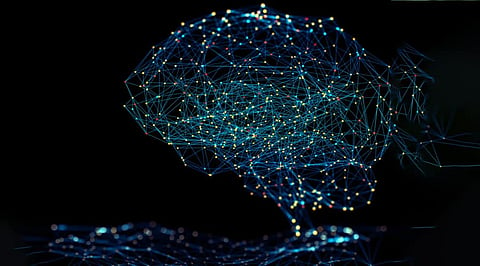
The universal sentence encoder makes getting sentence-level embeddings as easy as it has historically been to lookup the embeddings for individual words. The sentence embeddings can then be trivially used to compute sentence-level meaning similarity as well as to enable better performance on downstream classification tasks using less supervised training data. The universal sentence encoder model encodes textual data into high dimensional vectors known as embeddings which are numerical representations of the textual data. It specifically targets transfer learning to other NLP tasks, such as text classification, semantic similarity, and clustering. The pre-trained universal sentence encoder is publicly available in Tensorflow-hub.
It is trained on a variety of data sources to learn for a wide variety of tasks. The sources are Wikipedia, web news, web question-answer pages, and discussion forums. The input is the variable-length English text and the output is a 512-dimensional vector. It has shown excellent performance on the semantic textual similarity (STS) benchmark. Earlier sentence embeddings were calculated by averaging all the embeddings of the words in the sentence, however, just adding or averaging had limitations and was not suited for deriving the true semantic meaning of the sentence. The universal sentence encoder makes getting sentence-level embeddings easy. After knowing how the universal sentence encoder works, it's best to have hands-on experience starting from how to load the pre-trained model to using the embeddings in getting similarity measures between sentences. Below are a few examples of how to use the model.
The universal sentence encoder encodes text into high dimensional vectors that can be used for text classification, semantic similarity, clustering, and other natural language tasks. The pre-trained universal sentence encoder is publicly available in Tensorflow-hub. It comes with two variations i.e., one trained with transformer encoder and the other trained with Deep Averaging Network (DAN). The two have a trade-off of accuracy and computational resource requirement. While the one with the transformer encoder has higher accuracy, it is computationally more intensive. The one with DNA encoding is computationally less expensive and with little lower accuracy.
Pre-trained sentence embeddings have been shown to be very useful for a variety of NLP tasks. Due to the fact that training such embeddings requires a large amount of data, they are commonly trained on a variety of text data. An adaptation to specific domains could improve results in many cases, but such a finetuning is usually problem-dependent and poses the risk of over-adapting to the data used for adaptation. In this paper, we present a simple universal method for finetuning Google's universal sentence encoder (USE) using a Siamese architecture. We demonstrate how to use this approach for a variety of data sets and present results on different data sets representing similar problems. The approach is also compared to traditional finetuning on these data sets. As a further advantage, the approach can be used for combining data sets with different annotations. We also present an embedding finetuned on all data sets in parallel.
Join our WhatsApp Channel to get the latest news, exclusives and videos on WhatsApp
_____________
Disclaimer: Analytics Insight does not provide financial advice or guidance. Also note that the cryptocurrencies mentioned/listed on the website could potentially be scams, i.e. designed to induce you to invest financial resources that may be lost forever and not be recoverable once investments are made. You are responsible for conducting your own research (DYOR) before making any investments. Read more here.