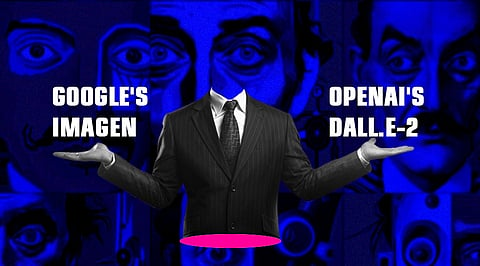
The AI imagery competition is getting tough. Google this week unveiled a new challenger to OpenAI's vaunted DALLE-2 text-to-image generator — and took shots at its rival's efforts. Both models convert text prompts into pictures. But Google's researchers claim their system provides "unprecedented photorealism and deep language understanding." Greetings humanoids. Qualitative comparisons between Imagen and DALL-E 2 on DrawBench prompts from the Conflicting category.
Early last year OpenAI showed off a remarkable new AI model called DALL-E (a combination of WALL-E and Dali), capable of drawing nearly anything and in nearly any style. But the results were rarely something you'd want to hang on the wall. Now DALL-E 2 is out, and it does what its predecessor did much, much better, in fact. But the new capabilities come with new restrictions to prevent abuse. DALL-E was described in detail in our original post on it, but the gist is that it is able to take quite complex prompts, such as "A bear riding a bicycle through a mall, next to a picture of a cat stealing the Declaration of Independence." It would gladly comply, and out of hundreds of outputs find the most likely to meet the user's standards. DALL-E 2 does the same thing fundamentally, turning a text prompt into a surprisingly accurate image. But it has learned a few new tricks. First, it's just plain better at doing the original thing. The images that come out the other end of DALL-E 2 are several times bigger and more detailed. It's actually faster despite producing more imagery, meaning more variations can be spun out in the handful of seconds a user might be willing to wait. DALL-E 2 runs on a hosted platform for now, an invite-only test environment where developers can try it out in a controlled way. Part of that means that all their prompts for the model are evaluated for violations of a content policy that prohibits, as they put it, "images that are not G-rated."
Google Research has developed a competitor for OpenAI's text-to-image system, with its own AI model that can create artworks using a similar method. Text-to-image AI models are able to understand the relationship between an image and the words used to describe it. Once a description is added, a system can generate images based on how it interprets the text, combining different concepts, attributes, and styles. For example, if the description is 'a photo of a dog', the system can create an image that looks like a photograph of a dog. But if this description is altered to 'an oil painting of a dog', the image generated would look more like a painting. Imagen's team has shared a number of example images that the AI model has created – ranging from an acute corgi in a house made from sushi, to an alien octopus reading a newspaper. OpenAI created the first version of its text-to-image model called DALL-E last year. But it unveiled an improved model called DALL-E 2 last month, which it said: "generates more realistic and accurate images with four times greater resolution". The AI company explained that the model uses a process called diffusion, "which starts with a pattern of random dots and gradually alters that pattern towards an image when it recognises specific aspects of that image". In a newly published research paper, the team behind Imagen claims to have made several advances in terms of image generation. It says large frozen language models trained only on text data are "surprisingly very effective text encoders" for text-to-image generation. It also suggests that scaling a pretrained text encoder improves sample quality more than scaling an image diffusion model size. Google's research team created a benchmark tool to assess and compare different text-to-image models, called DrawBench. Using DrawBench, Google's team said human raters preferred Imagen over other models such as DALL-E 2 in side-by-side comparisons "both in terms of sample quality and image-text alignment".
Similarly, to OpenAI, Google Research said there are several ethical challenges to be considered with text-to-image research. The team said these models can affect society in "complex ways" and that the risk of misuse raises concerns in terms of creating open-source code and demos. "The data requirements of text-to-image models have led researchers to rely heavily on large, mostly uncurated, web-scraped datasets," the research paper said. While this approach has enabled rapid algorithmic advances in recent years, datasets of this nature often reflect social stereotypes, oppressive viewpoints, and derogatory, or otherwise harmful, associations to marginalized identity groups. The researchers also said that preliminary analysis of Imagen suggests that the model encodes a range of "social and cultural biases" when generating images of activities, events, and objects. When Open-AI unveiled DALL-E 2 last month, concerns were raised that this technology could help people spread disinformation online through the use of authentic-looking fake images.
Join our WhatsApp Channel to get the latest news, exclusives and videos on WhatsApp
_____________
Disclaimer: Analytics Insight does not provide financial advice or guidance. Also note that the cryptocurrencies mentioned/listed on the website could potentially be scams, i.e. designed to induce you to invest financial resources that may be lost forever and not be recoverable once investments are made. You are responsible for conducting your own research (DYOR) before making any investments. Read more here.