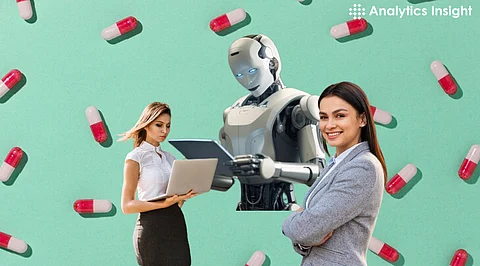
Generative AI is already a sub-branch of artificial intelligence involved in the invention of new varieties of such content, either visual, textual or even molecular. The changes right at the very end, by Generative AI, are too many and across a wide array of industries.
The power of generative AI lies in deep delivery for quicker drug discovery, optimum clinical trials, and hence personalized medicine for better health outcomes. Still, further on what the shared article elucidates is how some of the world-leading pharma companies are using generative AI to attain such transformational benefits.
Also, there are reasons to look for cybersecurity solutions in the pharma industry. Pharma businesses need to be aware of the main cybersecurity dangers in 2024 since they could cost them money and tarnish their reputations. Pharmaceutical organizations may be shielded from dangerous actors with the aid of cyber security solutions, and they can feel secure knowing that their data is safe.
Innovation is evolving extremely rapidly throughout every sector. The pharmaceutical industry is keeping up with its operating base and stringent regulatory climate. The industry must find ways to keep these improvements up to the mark to guarantee that products are still suitable to produce.
There have been a growing number of indications that the use of this AI branch in pharmaceutical research and development is being taken up by these top ultra-competitors in the niche.
Increasingly, pharma and biotech organizations are embracing more effective, automated processes that integrate data-driven decisions and utilize predictive analytics tools. The next development of this approach to deal with cutting-edge data analytics fuses machine learning and AI. Thus, the pharma industry has a new drug and innovations.
This paves way for drug makers and pharma companies to invest hugely in artificial intelligence and machine learning with the hope that these technologies will make the drug discovery process faster and cheaper.
Novartis embraces generative AI algorithms in molecular property estimation, key in the drug discovery process. It has been one of the very early company adopters of the application of artificial intelligence in robust analytics of massive molecular data sets. This is to increase the search pace of potential drug candidates and the pointing of the design of molecules.
Not new to running clinical trials, Pfizer uses generative AI in the process of patient response anticipation to treatments that allow them to optimize the design of clinical trials. In that regard,, it reduces the time and money needed to bring new therapies to market.
One of the large pharmaceutical houses growing generative AI in target identification and validation is AstraZeneca.
It brings new medical insights that will fuel the process of target identification, thus increasing the pace of discovering new drugs and maximizing opportunity possibilities for developing effective therapy against many diseases by engaging with the grand challenges of understanding massive and complex biological data.
Now, Merck is engaged in AI-generative target molecule generation and optimization. With automation in the design of molecules, Merck will develop newer therapies with better safety and efficacy profiling for treating ill patients.
One such program is the Johnson & Johnson drug repurposing event, whereby the drugs available to the firm with alternative indications are considered. With deep clinical data and real-world evidence mining, Johnson & Johnson is not in an easy position to pinpoint new indication opportunities for developed drugs but in the development of new uses for established drugs.
Generative AI confers several critical advantages that shift pharma as follows:
Accelerated Drug Discovery: Traditional approaches to drug discovery are very laborious and costly. Normally, large chemical compound data sets allow generative AI algorithms to compute biochemical activities against an exponential rendering process in the name of quick drug candidate identification. These really can end up meeting the immediate medicinal need with a lot better treatment methods for patients.
This is not an easy exercise in the design of clinical trials since many variables occur that take place and have to be considered individually, patient demographics, treatment efficacy, which may give rise to rules of suspension, and the concept of safety of a new treatment.
Generative AI could be applied in such a way that it will be generativity optimized to the successful designing of clinical trials inclusive of patient risks and predictions of the response of patients, hence the associated risks and costs in case of unsuccessful trial sentiments.
Optimization in this regard increases the pace of development and reduces the associated risk with the chances of success.
This is because different patients have variable responses to therapy that can be genetic or based on lifestyle and medical history. This is what defines medicine as evidence-based yet personalized medicine empowered by generative AI.
It represents big data associated with patient information and finds, together with predictive analytics, the optimum fit for customized treatment plans according to characteristics. It hereby raises the overall efficiency of treatment, reduces the chances of side effects, and gives better care and satisfaction to patients.
Identification and validation of targets: This simply means the identification of suitable targets for which drugs are to be developed. Generative AI/ML-based analysis of sophisticated biological data types, with special regard to genomics and proteomics, shall help in better defining the mechanisms of diseases and potential drug targets arising from traditional analyses of data.
Such an output would then be produced with precision great enough to lead to an acceleration in the creation of new therapies with a high likelihood for the developed therapies targeting the cause of the disease.
Generative AI applications in the pharmaceutical sector are vast and growing. Among the best current uses are:
Drug Repurposing: AI-driven insight into molecular properties and biological activity of existing drugs can help apply them for new therapeutic uses.
Clinical Decision Support: Help healthcare providers in making informed decisions based on differential analyzes of patient data and provide mosaic-like treatment recommendations accordingly by AI-powered algorithms.
Predictive Analytics: Incorporate such AI-driven predictive models for predicting disease trends in order to optimize supply chains and generally improve health care delivery using large-scale epidemiological and healthcare data analysis.
Generative AI has a very high value and can shape the future of pharmaceutical research and development. If one considers the rising set of possibilities that technology is just about to offer, Artificial Intelligence would bring implications all along in the process of discovery, clinical trials, and personalized medicine.
First and foremost, therefore, AI will hugely benefit pharma companies using generative AI with innovations that enhance treatment efficacy, reduce healthcare costs, and foster better patient results across the world.
Now, with the current adoption of generative AI by leading pharma firms, a tremendous shift should come into their approach toward much more efficient and targeted healthcare solutions. Therefore, such firms will be better positioned to lead in the process of the discovery and development of new therapies. These therapies, with the help of AI-driven insights and analytics their scientists help create, cater much better to health challenges across the world.
1. How Can One Apply Generative AI Across Pharmaceuticals?
Pharma companies using generativeAI can be primarily referred to as a generation of new molecules or optimization of other relevant periods in the process related to drug discovery and personalization of treatment plans through patient data.
2. How Artificial Intelligence Can Help Pharmaceutical Companies in Drug Discovery?
The pharma companies using generative AI here can make use of AI for more efficient analyses of huge data sets concerning chemical compounds and predict biological activities. Thus, with this method, defining the probable drug candidates is done in less laborious ways than usual.
3. Can AI Help in Personalized Medicine?
Although it can, theoretically, go through patient data, genetic information, and medical history in the course of developing treatment plans and concomitant setting or determination of a patient's therapeutic responses in the course of development of PM, it proved to be very problematic when applied in real-world applications.
4. What is the advantage of AI within a clinical trial setting?
AI makes clinical trials rigorous through the prediction of patient response, optimality of trial design, and minimization of associated risks and costs in trial failures that enable faster acceleration in new therapy developments.
5. Is AI replacing traditional means in pharma research?
AI is an add-up to the traditional modes of contribution towards the pharma companies using generative AI through enhanced efficiency and cost reduction, going through novel therapies beyond what conventional means could do or see.