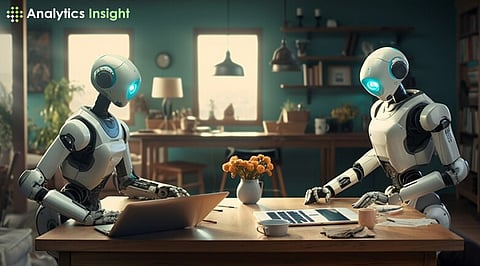
Generative AI has made significant strides in recent years, evolving from niche technologies to powerful tools that impact various industries. Among these advancements are multimodal AI models, small language models, autonomous agents, open models, and cloud-native solutions. Here, we will explore the most influential generative AI models that are shaping the future, highlighting their key features, applications, and transformative potential:
Multimodal AI models are at the forefront of generative AI advancements. Unlike traditional models that process a single type of data, multimodal AI integrates text, images, and audio to provide a more comprehensive understanding of information. This holistic approach enhances decision-making and creates immersive user experiences.
A standout example is Google's Gemini 1.0, unveiled in December 2023. This model exemplifies versatility and integration by seamlessly handling multiple data types. Gemini 1.0's ability to operate across various platforms from data centers to mobile devices, demonstrates its flexibility and scalability. Its advanced reasoning and problem-solving capabilities even rival those of human experts, showcasing the transformative potential of multimodal AI.
Small Language Models (SLMs) are gaining traction for their specialized capabilities in specific applications. These models are increasingly used to develop domain-specific language models tailored to unique business needs. They offer a focused approach that can outperform larger models in certain benchmarks.
Microsoft's Phi-2, introduced in December 2023, is a prime example of this trend. With 2.7 billion parameters, Phi-2 was trained on 1.4 trillion tokens of synthetic data and demonstrated superior performance on various benchmarks compared to larger models. Its ability to excel in coding, reasoning, and language understanding underscores the growing importance of specialized, compact language models.
Autonomous agents can be said to be a rather higher level in AI functionality. These are independent software programs which are capable of learning and functioning and setting their responses to various conditions that exist and are thus self-acting in their accomplishment of the targets. They are destined to transform the world of business processes, and user interfaces.
Smart Eye's Emotion AI, launched in January 2024, exemplifies the potential of autonomous agents in automotive technology. With the help of advanced automotive sensing and LLM, Emotion AI helps in-car assistants in identifying drivers’ emotions. This innovation enhances road safety and creates more personalized and intuitive driving experiences.
Open models are emerging and refacing the generative AI solutions. These models use open-source large language models, and the architecture and components can be fine-tuned or extended to fit the application’s requirements. They represent a step towards achieving Artificial General Intelligence (AGI) and have broad applications across various sectors.
A good example is the Meta and Microsoft’s Llama 2 that was launched in July 2023. Now part of the Azure AI model catalog, Llama 2 gives developers a clear pipeline of how to use generative AI effectively. It stresses the necessity of the open models with the tradition in the enhancement of the effectiveness of the AI systems as exemplified by its integration with Microsoft Azure.
Cloud-native infrastructure is crucial for the growth of generative AI, offering scalable and efficient environments for AI workloads. Cloud platforms are evolving to support large language models (LLMs) and provide optimized architectures and tools for AI applications.
According to EY, in August 2023, 78% of the enterprises have adopted or are planning to adopt cloud as part of the technology refresh and integration of intelligence in business applications. Therefore, cloud technologies’ implementation is crucial for maximizing generative AI capabilities and not repeating mistakes made with traditional CRM systems.
StyleGAN and StyleGAN2 have turned the world of generative adversarial networks (GANs) on its head to allow for the generation of photorealistic images. These models improve image quality and the range of image variations when compared to the previous GAN and brought the concept of style vectors to exist.
Different applications include, logo design, graphical user interfaces, and in the healthcare industry among others brought by StyleGAN and StyleGAN2. Their excelled capability in realism has led to new possibilities in design experiences and generation of virtual contents.
Contrastive Language-Image Pretraining (CLIP) represents a breakthrough in multimodal learning by integrating textual and visual data. This approach trains models on extensive datasets of images paired with textual descriptions, bridging the gap between text and visuals.
The developments in the technology and the StableRep+ variant are now some of the benchmarks for AI training effectiveness. Doing so is especially relevant for now utilized text-to-image generation competencies of CLIP, contextually most helpful within the healthcare domain, and depending on the accurate integration of lots of visual and textual information.
The Vision Transformer (ViT) has impacted the computer vision tasks through the use of the transformer architecture. Unlike standard CNNs that feed images as patches, extending the methods to tasks such as classification, detection, and segmentation, ViTs have outperformed them.
Current studies and future improvements, such as FastViT, search for ways to optimize the ViT and asset memory consumption. This trend is illustrated by Apple’s FastViT, unveiled in August 2023, which provides high speed with reduced latency in operation on mobile gadgets and desk- top GPUs.
Hybrid models that combine generative and predictive AI methodologies are gaining prominence, particularly in medical imaging. What is more important about these models is that they combine the generative creativity and accurate prediction of future events to solve various issues.
Lenovo and NVIDIA's expanded partnership, announced in October 2023, illustrates the potential of hybrid models. Their new solutions allow for AI to compute from edge to cloud and empower tailored applications as well as the application of generative AI.
Edge computing and on-device AI are transforming generative AI by enabling real-time, localized processing. Deploying AI models and AI applications transform the use of smartphone and IoT devices by improving privacy, low latency, and developing new high impact use cases.
Edge computing is best illustrated by Micron Technology’s LPDDR5X memory unveiled in October 2023. This is a low-power memory tailored for Qualcomm’s Snapdragon 8 Gen 3 platform to expedite performance necessary for edge-meshed generative AI with advantages such as low power use and enhanced computational speed.
The development of these ten influential generative AI models underscores the swift growth and broadening features of AI technologies. From models that merge different data formats to mixed models that blend generative and forecasting techniques, these progressions are molding the future of AI. As these technologies progress, they will propel innovation throughout sectors, improving user experiences and changing the way companies function.