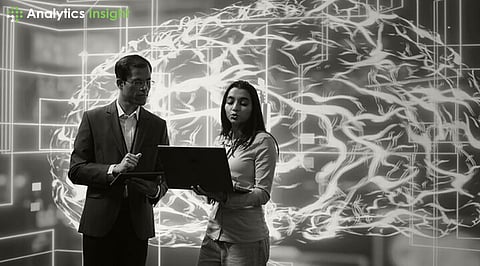
Generative AI models have revolutionized the field of artificial intelligence, with applications ranging from art creation to realistic image generation. Among these models, StyleGAN and its successor, StyleGAN2, stand out for their remarkable ability to generate high-quality, photorealistic images. Developed by NVIDIA, these models have set new standards in the world of generative AI. This article provides a detailed analysis of generative AI models, specifically comparing StyleGAN and StyleGAN2, to understand their features, improvements, and implications for the future of AI.
Generative AI models are a class of machine learning frameworks designed to generate new data instances that resemble existing data. These models are trained on vast datasets and learn to create outputs that mimic the patterns and features of the input data. StyleGAN and StyleGAN2, based on Generative Adversarial Networks (GANs), are among the most advanced models in this category.
StyleGAN, introduced by NVIDIA in 2018, marked a significant advancement in the field of generative AI. This model uses a novel approach to image synthesis, enabling the generation of highly detailed and realistic images. The key innovation of StyleGAN lies in its ‘style’ based architecture, which allows for unprecedented control over the image generation process.
Style-Based Generator Architecture: Unlike traditional GANs, StyleGAN introduces an intermediate latent space, enabling more control over image attributes at various levels of detail.
Progressive Growing: This technique trains the model to generate images starting from low resolution and gradually increasing to higher resolutions, improving image quality.
Separation of High-Level Attributes: StyleGAN separates high-level attributes (e.g., pose, identity) from stochastic variations (e.g., freckles, hair) to enhance control over the generated images.
StyleGAN2, released in 2019, builds upon the foundation of its predecessor with several key improvements. The enhancements in StyleGAN2 address some of the limitations of StyleGAN and further push the boundaries of what generative AI models can achieve.
Revised Architecture: StyleGAN2 refines the generator and discriminator networks, leading to improved training stability and image quality.
Path Length Regularization: This new technique ensures more consistent and smoother latent space interpolations, resulting in more realistic image transitions.
Removal of Artifacts: StyleGAN2 addresses common artifacts in generated images, such as droplet-like imperfections, producing cleaner and more natural images.
Adaptive Discriminator Augmentation: This technique enhances the discriminator’s ability to generalize, further improving the fidelity of generated images.
One of the most significant differences between StyleGAN and StyleGAN2 is the improvement in image quality. StyleGAN2 generates images with higher fidelity, reduced artifacts, and better overall realism. The enhancements in architecture and training techniques contribute to these improvements, making StyleGAN2 the superior model in terms of output quality.
StyleGAN2’s revised architecture addresses several issues present in StyleGAN. The introduction of path length regularization and the removal of artifacts are crucial advancements that lead to more stable and reliable image generation. These architectural refinements make StyleGAN2 a more robust model for various generative tasks.
Both StyleGAN and StyleGAN2 offer significant control over the image generation process. However, StyleGAN2’s improvements in the latent space representation and interpolation consistency provide even greater flexibility and precision. This enhanced control is particularly beneficial for applications requiring fine-grained adjustments to the generated images.
StyleGAN2 introduces adaptive discriminator augmentation, which improves the training efficiency of the model. This technique allows the discriminator to generalize better, reducing the amount of training data required and accelerating the training process. Consequently, StyleGAN2 can achieve high-quality results more efficiently than its predecessor.
Generative AI models like StyleGAN and StyleGAN2 have found widespread use in the realm of art and creativity. Artists and designers use these models to generate unique artworks, explore new styles, and even create digital content that would be impossible to produce manually. The control offered by these models allows for the creation of highly personalized and imaginative pieces.
StyleGAN and StyleGAN 2 gained popularity in the field of medical imaging and autonomous driving because they are used for data simulation. These types of models are performed by generating convincing artificial data that aid in machine learning training, which in turn, delivers accuracy and robustness. Primarily, it is the superb graphical quality of StyleGAN2-derived images that adds specific value to them being applied in those areas.
The gaming and virtual reality industries to the greatest extent benefit from the progress in generative AI models. StyleGAN and StyleGAN2 are provided to enable the creation of realistic characters, environments, and textures making the users live prisoners in a real-life game. The ability to provide various and intricate assets within a short time and without high costs is a revolutionary force in these fields.
The shift from StyleGAN to StyleGAN2 has proved to be an important landmark in the evolution of generative AI models. The improvements in the image quality of StyleGAN2, the architectural refinements, and training efficiency are the applications of these models that increase their utilization in various fields.
The development of generative AI will spawn new horizons and give rise to innovation in society. The detailed research of the generative AI method such as the analysis of the StyleGAN and StyleGAN2 models emphasizes the significance of continuous improvement and adaptation in the rapidly changing world of artificial intelligence.