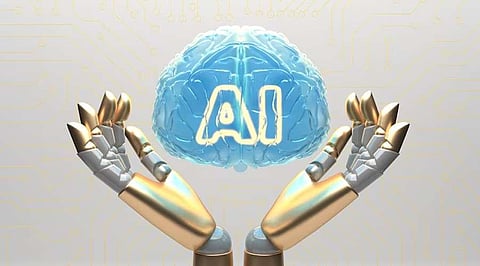
Generative AI is a branch of artificial intelligence that can create new content or data from existing ones, such as images, text, audio, or video. Generative AI has many applications, such as content creation, data augmentation, image synthesis, text summarization, and more. Some examples of generative AI models are ChatGPT, BERT, LaMDA, GPT-3, DALL-E-2, MidJourney, and Stable Diffusion.
However, generative AI also has a significant environmental impact that is often overlooked. The development and use of these models require huge amounts of energy and water, which contribute to greenhouse gas emissions and resource depletion. Moreover, generative AI can also have other negative effects on the environment, such as reducing nature experiences and worsening the effects of misuse and bias.
This article will explore generative AI's environmental impact and suggest ways to make it greener.
The environmental impact of generative AI can be measured by three factors: the carbon footprint from training the model, the carbon footprint from running inference with the model, and the carbon footprint from producing the computing hardware and cloud data center capabilities.
Training a generative AI model involves feeding a large amount of data to a neural network and adjusting its parameters to minimize errors. This process requires much computational power, which consumes a lot of energy. The energy consumption depends on the model's size and complexity, the data's amount and quality, the type and number of processors used, and the duration of the training.
According to a 2019 report by Strubell et al., training a generative AI model called BERT (with 110 million parameters) consumed about 626 kWh of electricity and emitted about 284 kg of CO2 equivalent. This is equivalent to the carbon footprint of a round-trip transcontinental flight for one person using graphics processing units (GPUs) to train the model.
In comparison, training a more advanced generative AI model called GPT-3 (with 175 billion parameters) consumed about 1,287 MWh of electricity and emitted about 552 tons of CO2 equivalent. This is equivalent to the carbon footprint of 123 gasoline-powered passenger vehicles driven for one year or 125 homes' electricity use for one year.
Running inference with a generative AI model involves using the trained model to generate new content or data based on new input data, such as a prompt or a query. This process also requires computational power, but usually less than training. However, the energy consumption depends on the frequency and volume of inference requests, the complexity and length of the generated output, and the type and number of processors used.
According to a study by Saenko et al., running inference with a generative AI model called ChatGPT (with 1.5 billion parameters) consumed about 0.0004 kWh per query. It emitted about 0.0002 kg of CO2 equivalent. This is four to five times higher than a simple search engine query.
Producing computing hardware and cloud data center capabilities involves manufacturing, transporting, installing, maintaining, and disposing of various components such as servers, processors, memory chips, cooling systems, cables, etc. This process also consumes energy and emits greenhouse gases throughout its lifecycle.
According to a study by Masanet et al., producing one server consumed about 5.12 GJ of primary energy and emitted about 630 kg of CO2 equivalent in 2010. These numbers are expected to increase due to the growing demand for more powerful and efficient hardware.
Moreover, producing cloud data center capabilities involves building and operating physical facilities that house thousands of servers and other equipment. These facilities also consume energy and water for cooling and power generation. According to an IEA (International Energy Agency) report, data centers accounted for about 1% of global electricity use in 2018. This share will grow as more data and services move to the cloud.
The environmental impact of generative AI is not inevitable or irreversible. There are several ways to make generative AI greener and more sustainable, such as:
We can enjoy its benefits and protect our planet by making generative AI greener.
Join our WhatsApp Channel to get the latest news, exclusives and videos on WhatsApp
_____________
Disclaimer: Analytics Insight does not provide financial advice or guidance. Also note that the cryptocurrencies mentioned/listed on the website could potentially be scams, i.e. designed to induce you to invest financial resources that may be lost forever and not be recoverable once investments are made. You are responsible for conducting your own research (DYOR) before making any investments. Read more here.